Towards a comprehensive evaluation of dimension reduction methods for transcriptomic data visualization
Communications biology(2022)
摘要
The authors provide an evaluation framework for dimension reduction methods that illuminates the strengths and weaknesses of different algorithms, and applies this framework to evaluate the PCA, t-SNE, UMAP, TriMap, PaCMAP, ForceAtlas2, and PHATE algorithms. Dimension reduction (DR) algorithms project data from high dimensions to lower dimensions to enable visualization of interesting high-dimensional structure. DR algorithms are widely used for analysis of single-cell transcriptomic data. Despite widespread use of DR algorithms such as t-SNE and UMAP, these algorithms have characteristics that lead to lack of trust: they do not preserve important aspects of high-dimensional structure and are sensitive to arbitrary user choices. Given the importance of gaining insights from DR, DR methods should be evaluated carefully before trusting their results. In this paper, we introduce and perform a systematic evaluation of popular DR methods, including t-SNE, art-SNE, UMAP, PaCMAP, TriMap and ForceAtlas2. Our evaluation considers five components: preservation of local structure, preservation of global structure, sensitivity to parameter choices, sensitivity to preprocessing choices, and computational efficiency. This evaluation can help us to choose DR tools that align with the scientific goals of the user.
更多查看译文
关键词
Data mining,Data processing,Machine learning,Life Sciences,general
AI 理解论文
溯源树
样例
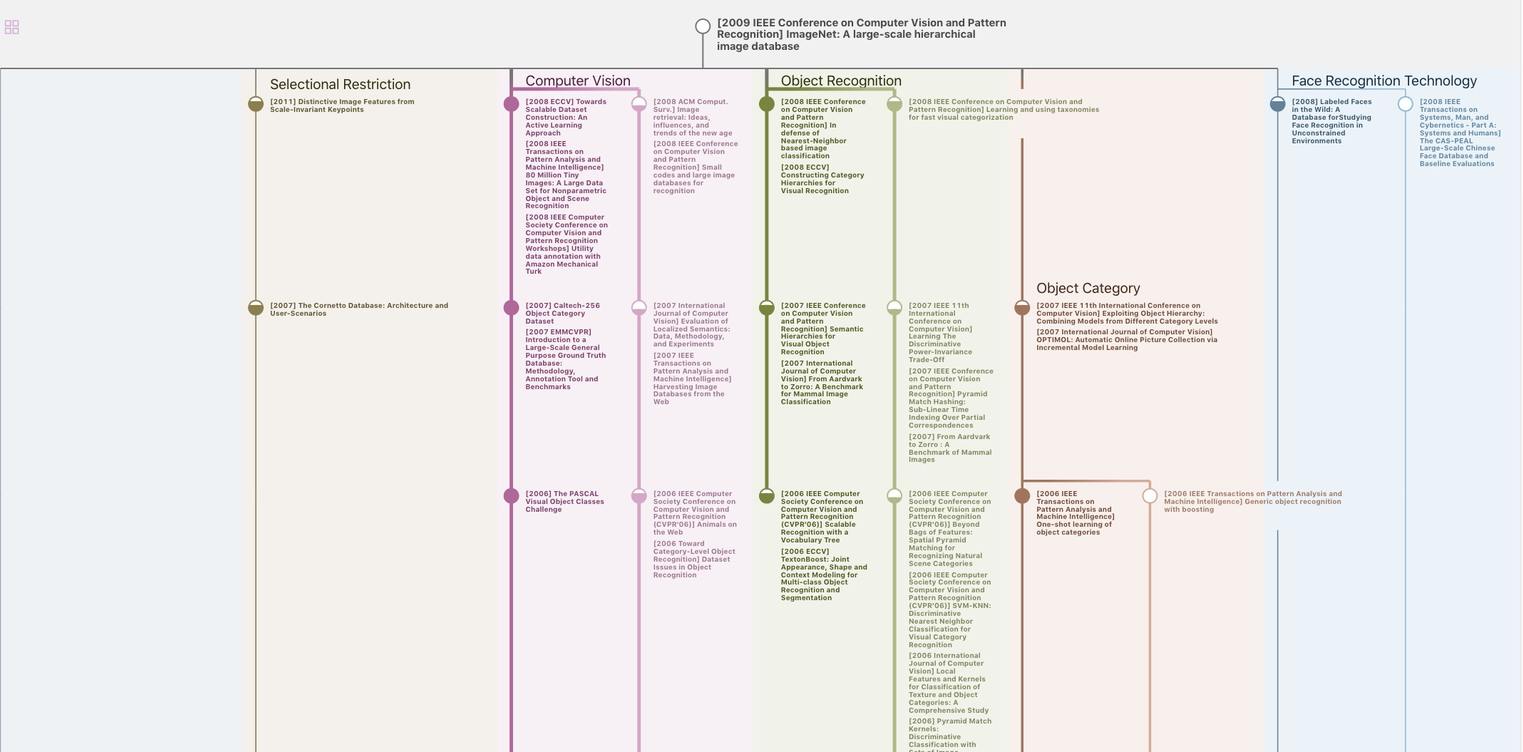
生成溯源树,研究论文发展脉络
Chat Paper
正在生成论文摘要