A New Graph Autoencoder-Based Consensus-Guided Model for scRNA-seq Cell Type Detection
IEEE TRANSACTIONS ON NEURAL NETWORKS AND LEARNING SYSTEMS(2024)
摘要
Single-cell RNA sequencing (scRNA-seq) technology is famous for providing a microscopic view to help capture cellular heterogeneity. This characteristic has advanced the field of genomics by enabling the delicate differentiation of cell types. However, the properties of single-cell datasets, such as high dropout events, noise, and high dimensionality, are still a research challenge in the single-cell field. To utilize single-cell data more efficiently and to better explore the heterogeneity among cells, a new graph autoencoder (GAE)-based consensus-guided model (scGAC) is proposed in this article. The data are preprocessed into multiple top-level feature datasets. Then, feature learning is performed by using GAEs to generate new feature matrices, followed by similarity learning based on distance fusion methods. The learned similarity matrices are fed back to the GAEs to guide their feature learning process. Finally, the abovementioned steps are iterated continuously to integrate the final consistent similarity matrix and perform other related downstream analyses. The scGAC model can accurately identify critical features and effectively preserve the internal structure of the data. This can further improve the accuracy of cell type identification.
更多查看译文
关键词
Feature extraction,Representation learning,Genetic algorithms,Sequential analysis,Optimization,Data models,Mathematical models,Distance fusion,graph autoencoder (GAE),integration algorithm,single-cell RNA-seq
AI 理解论文
溯源树
样例
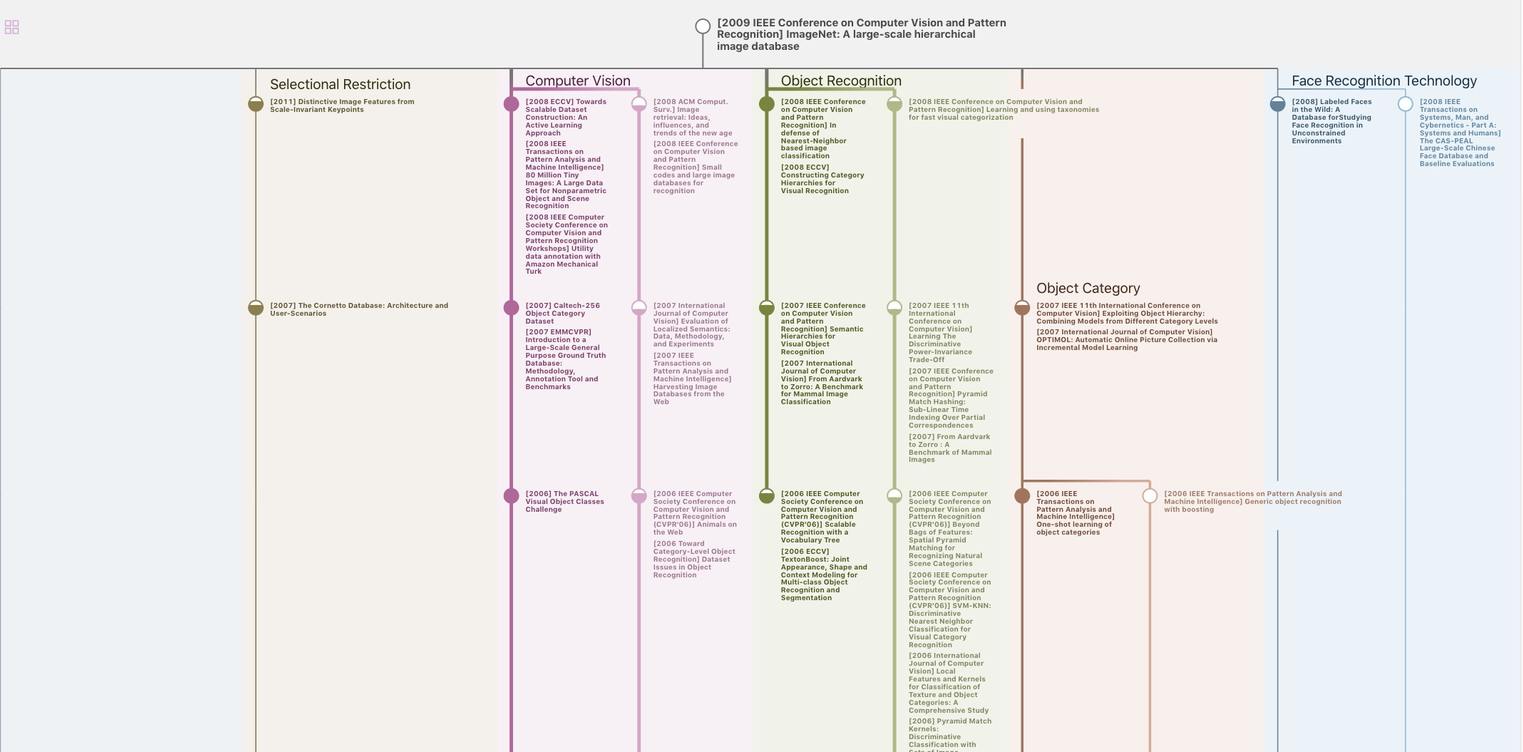
生成溯源树,研究论文发展脉络
Chat Paper
正在生成论文摘要