Boosting 3D Object Detection Via Object-Focused Image Fusion.
CoRR(2022)
- Pretraining has recently greatly promoted the development of natural language processing (NLP)
- We show that M6 outperforms the baselines in multimodal downstream tasks, and the large M6 with 10 parameters can reach a better performance
- We propose a method called M6 that is able to process information of multiple modalities and perform both single-modal and cross-modal understanding and generation
- The model is scaled to large model with 10 billion parameters with sophisticated deployment, and the 10 -parameter M6-large is the largest pretrained model in Chinese
- Experimental results show that our proposed M6 outperforms the baseline in a number of downstream tasks concerning both single modality and multiple modalities We will continue the pretraining of extremely large models by increasing data to explore the limit of its performance
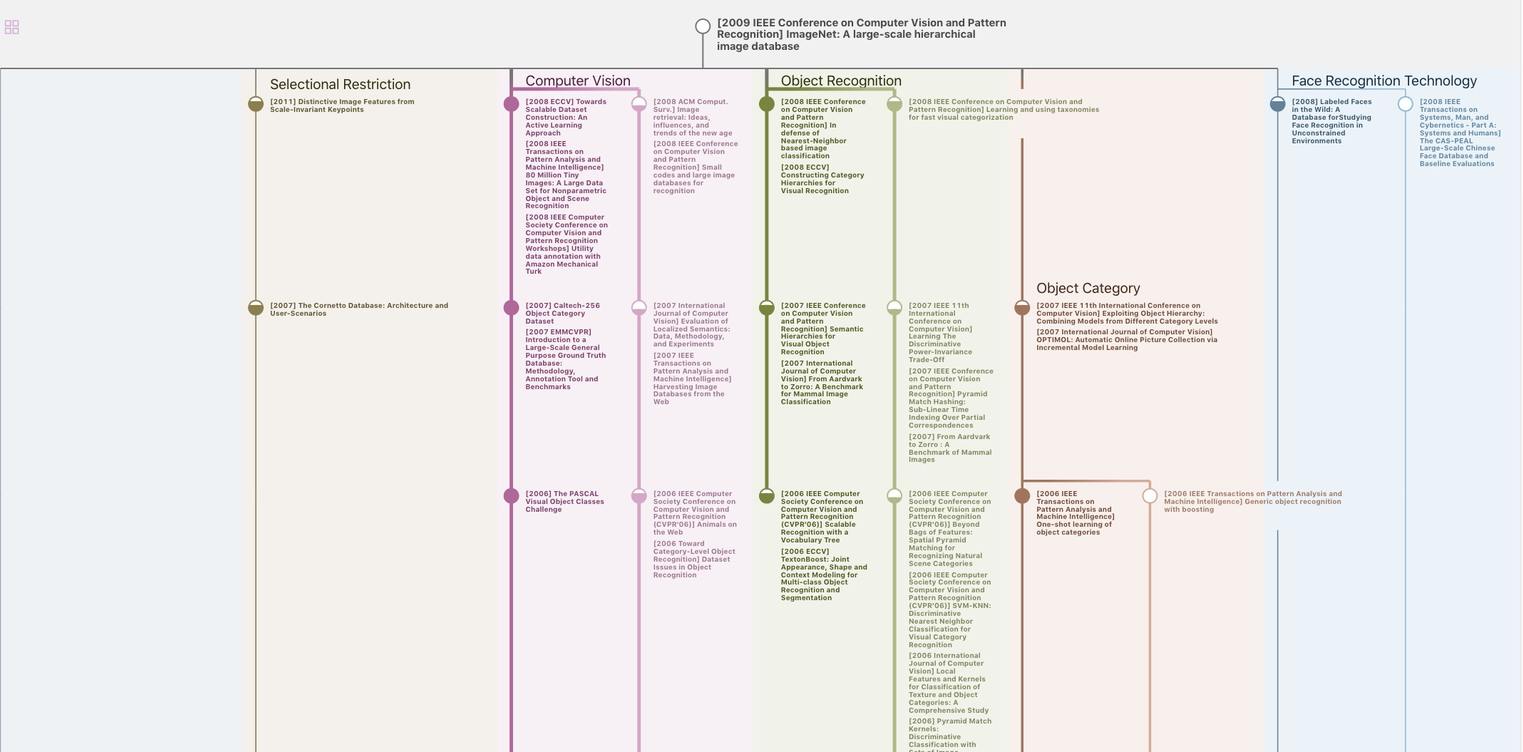
Review on 6D Object Pose Estimation with the Focus on Indoor Scene Understanding
被引用0
A Method to Create Real-Like Point Clouds for 3D Object Classification
被引用0
PiMAE: Point Cloud and Image Interactive Masked Autoencoders for 3D Object Detection.
被引用68
An Object SLAM Framework for Association, Mapping, and High-Level Tasks.
被引用3
Point-GCC: Universal Self-supervised 3D Scene Pre-training Via Geometry-Color Contrast
被引用13
UniTR: A Unified and Efficient Multi-Modal Transformer for Bird's-Eye-View Representation.
被引用8
TBFNT3D: Two-Branch Fusion Network with Transformer for Multimodal Indoor 3D Object Detection
被引用0
EFECL: Feature Encoding Enhancement with Contrastive Learning for Indoor 3D Object Detection.
被引用0
被引用0
CAF-RCNN: Multimodal 3D Object Detection with Cross-Attention
被引用0