Deep learning for de-convolution of Smad2 versus Smad3 binding sites
BMC Genomics(2022)
摘要
Background The transforming growth factor beta-1 (TGF β -1) cytokine exerts both pro-tumor and anti-tumor effects in carcinogenesis. An increasing body of literature suggests that TGF β -1 signaling outcome is partially dependent on the regulatory targets of downstream receptor-regulated Smad (R-Smad) proteins Smad2 and Smad3. However, the lack of Smad-specific antibodies for ChIP-seq hinders convenient identification of Smad-specific binding sites. Results In this study, we use localization and affinity purification (LAP) tags to identify Smad-specific binding sites in a cancer cell line. Using ChIP-seq data obtained from LAP-tagged Smad proteins, we develop a convolutional neural network with long-short term memory (CNN-LSTM) as a deep learning approach to classify a pool of Smad-bound sites as being Smad2- or Smad3-bound. Our data showed that this approach is able to accurately classify Smad2- versus Smad3-bound sites. We use our model to dissect the role of each R-Smad in the progression of breast cancer using a previously published dataset. Conclusions Our results suggests that deep learning approaches can be used to dissect binding site specificity of closely related transcription factors.
更多查看译文
关键词
Machine learning, Transcription regulation, Feature engineering
AI 理解论文
溯源树
样例
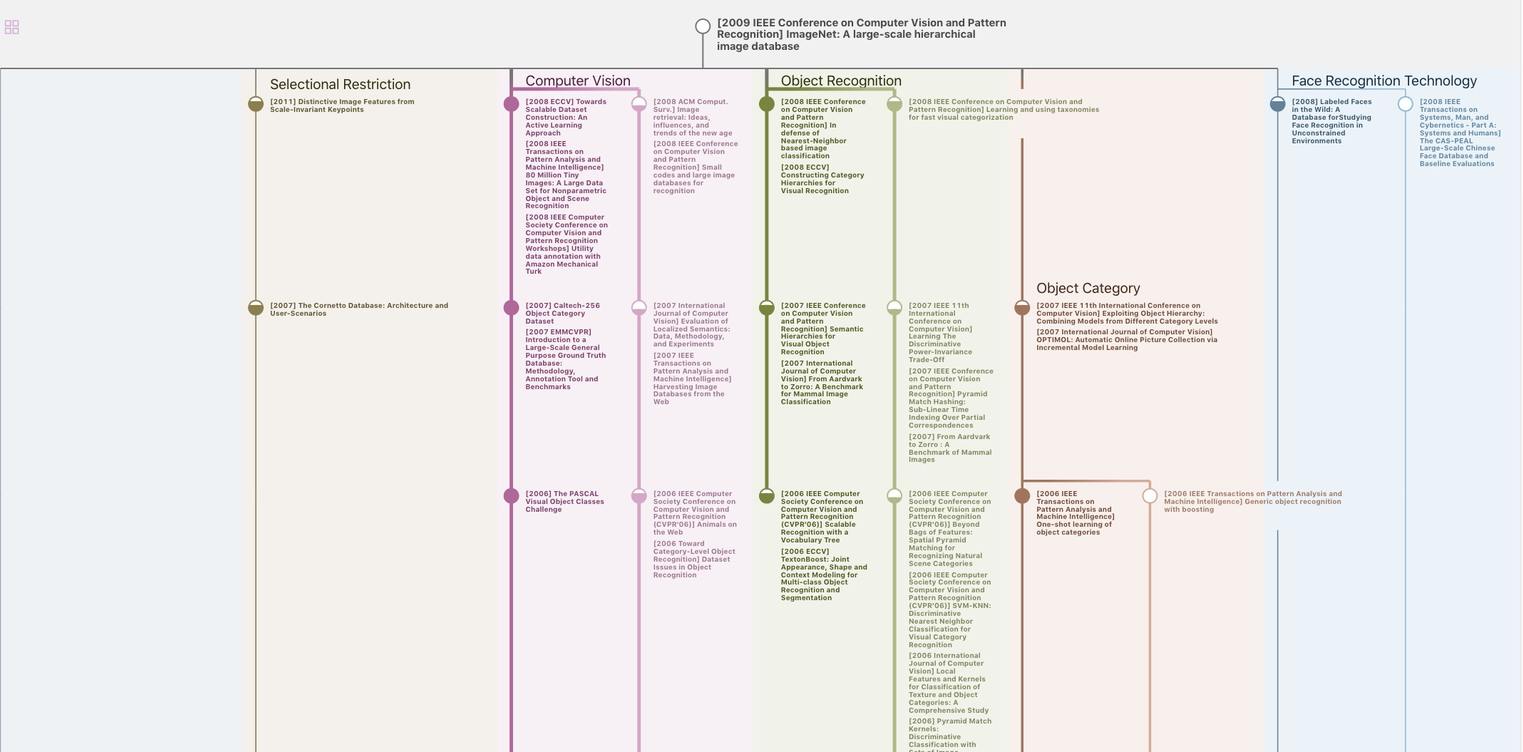
生成溯源树,研究论文发展脉络
Chat Paper
正在生成论文摘要