BMSS2: A Unified Database-Driven Modeling Tool for Systematic Biomodel Selection.
ACS synthetic biology(2022)
摘要
Modeling in synthetic biology constitutes a powerful means in our continuous search for improved performance with a rational Design-Build-Test-Learn approach. Particularly, kinetic models unravel system dynamics and enable system analysis for guiding experimental design. However, a systematic yet modular pipeline that allows one to identify the appropriate model and guide the experimental designs while tracing the entire model development and analysis is still lacking. Here, we develop BMSS2, a unified tool that streamlines and automates model selection by combining information criterion ranking with upstream and parallel analysis algorithms. These include Bayesian parameter inference, and identifiability analysis, and global sensitivity analysis. In addition, the database-driven design supports interactive model storage/retrieval to encourage reusability and facilitate automated model selection. This allows ease of model manipulation and deposition for the selection and analysis, thus enabling better utilization of models in guiding experimental design.
更多查看译文
关键词
genetic circuits,identifiability analysis,kinetic model,model selection,python package,synthetic and systems biology
AI 理解论文
溯源树
样例
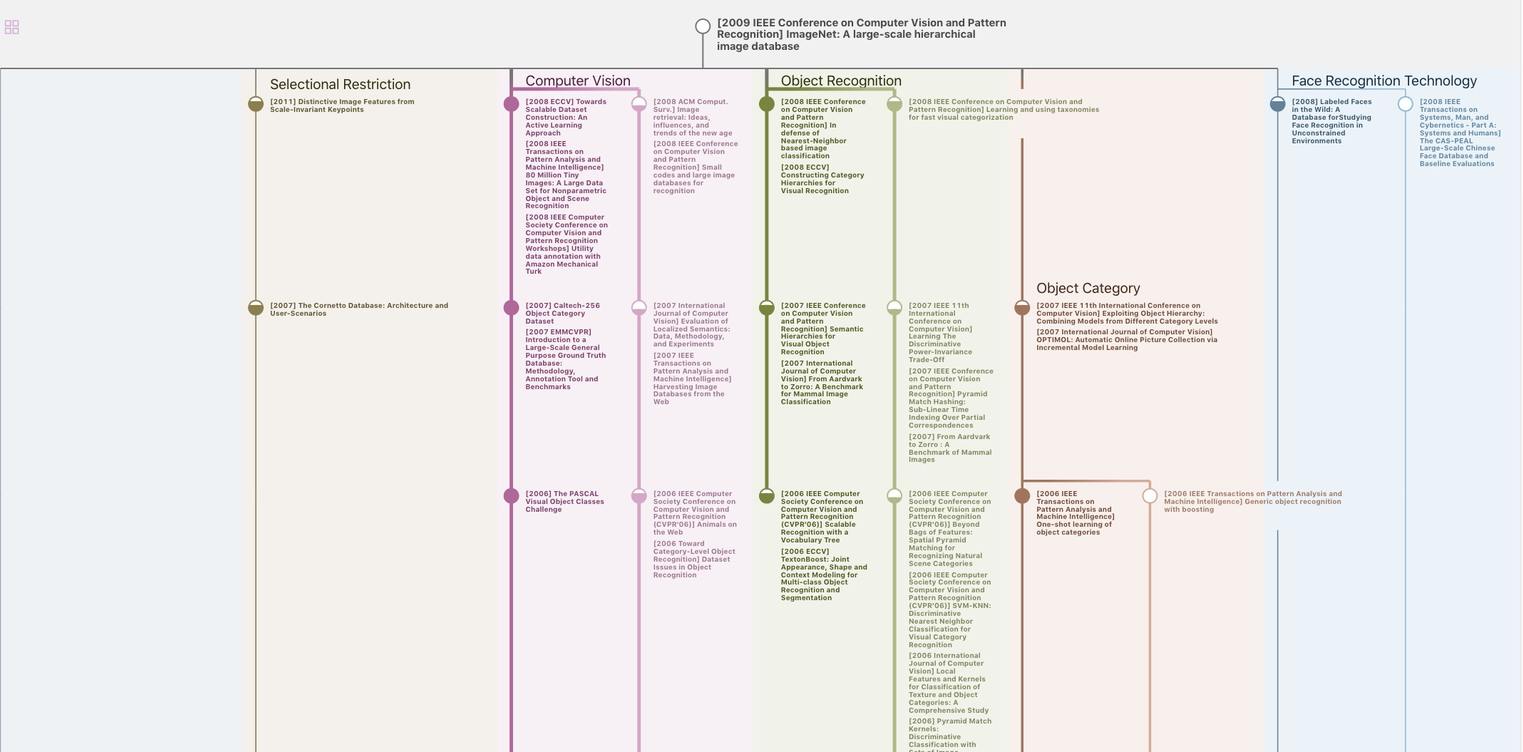
生成溯源树,研究论文发展脉络
Chat Paper
正在生成论文摘要