Prediction of Mortality and Major Adverse Kidney Events in Critically Ill Patients with Acute Kidney Injury
American journal of kidney diseases the official journal of the National Kidney Foundation(2022)
摘要
Rationale & ObjectiveRisk prediction tools for assisting acute kidney injury (AKI) management have focused on AKI onset but have infrequently addressed kidney recovery. We developed clinical models for risk stratification of mortality and major adverse kidney events (MAKE) in critically ill patients with incident AKI.Study DesignMulticenter cohort study.Setting & Participants9,587 adult patients admitted to heterogeneous intensive care units (ICUs; March 2009 to February 2017) who experienced AKI within the first 3 days of their ICU stays.PredictorsMultimodal clinical data consisting of 71 features collected in the first 3 days of ICU stay.Outcomes(1) Hospital mortality and (2) MAKE, defined as the composite of death during hospitalization or within 120 days of discharge, receipt of kidney replacement therapy in the last 48 hours of hospital stay, initiation of maintenance kidney replacement therapy within 120 days, or a ≥50% decrease in estimated glomerular filtration rate from baseline to 120 days from hospital discharge.Analytical ApproachFour machine-learning algorithms (logistic regression, random forest, support vector machine, and extreme gradient boosting) and the SHAP (Shapley Additive Explanations) framework were used for feature selection and interpretation. Model performance was evaluated by 10-fold cross-validation and external validation.ResultsOne developed model including 15 features outperformed the SOFA (Sequential Organ Failure Assessment) score for the prediction of hospital mortality, with areas under the curve of 0.79 (95% CI, 0.79-0.80) and 0.71 (95% CI, 0.71-0.71) in the development cohort and 0.74 (95% CI, 0.73-0.74) and 0.71 (95% CI, 0.71-0.71) in the validation cohort (P < 0.001 for both). A second developed model including 14 features outperformed KDIGO (Kidney Disease: Improving Global Outcomes) AKI severity staging for the prediction of MAKE: 0.78 (95% CI, 0.78-0.78) versus 0.66 (95% CI, 0.66-0.66) in the development cohort and 0.73 (95% CI, 0.72-0.74) versus 0.67 (95% CI, 0.67-0.67) in the validation cohort (P < 0.001 for both).LimitationsThe models are applicable only to critically ill adult patients with incident AKI within the first 3 days of an ICU stay.ConclusionsThe reported clinical models exhibited better performance for mortality and kidney recovery prediction than standard scoring tools commonly used in critically ill patients with AKI in the ICU. Additional validation is needed to support the utility and implementation of these models.Plain-Language SummaryAcute kidney injury (AKI) occurs commonly in critically ill patients admitted to the intensive care unit (ICU) and is associated with high morbidity and mortality rates. Prediction of mortality and recovery after an episode of AKI may assist bedside decision making. In this report, we describe the development and validation of a clinical model using data from the first 3 days of an ICU stay to predict hospital mortality and major adverse kidney events occurring as long as 120 days after hospital discharge among critically ill adult patients who experienced AKI within the first 3 days of an ICU stay. The proposed clinical models exhibited good performance for outcome prediction and, if further validated, could enable risk stratification for timely interventions that promote kidney recovery.
更多查看译文
关键词
Mortality,kidney recovery,acute kidney injury (AKI),critically ill patients,machine learning,major adverse kidney events (MAKE),risk prediction tool,intensive care unit (ICU),risk stratification,renal prognosis,AKI staging,clinical decision making
AI 理解论文
溯源树
样例
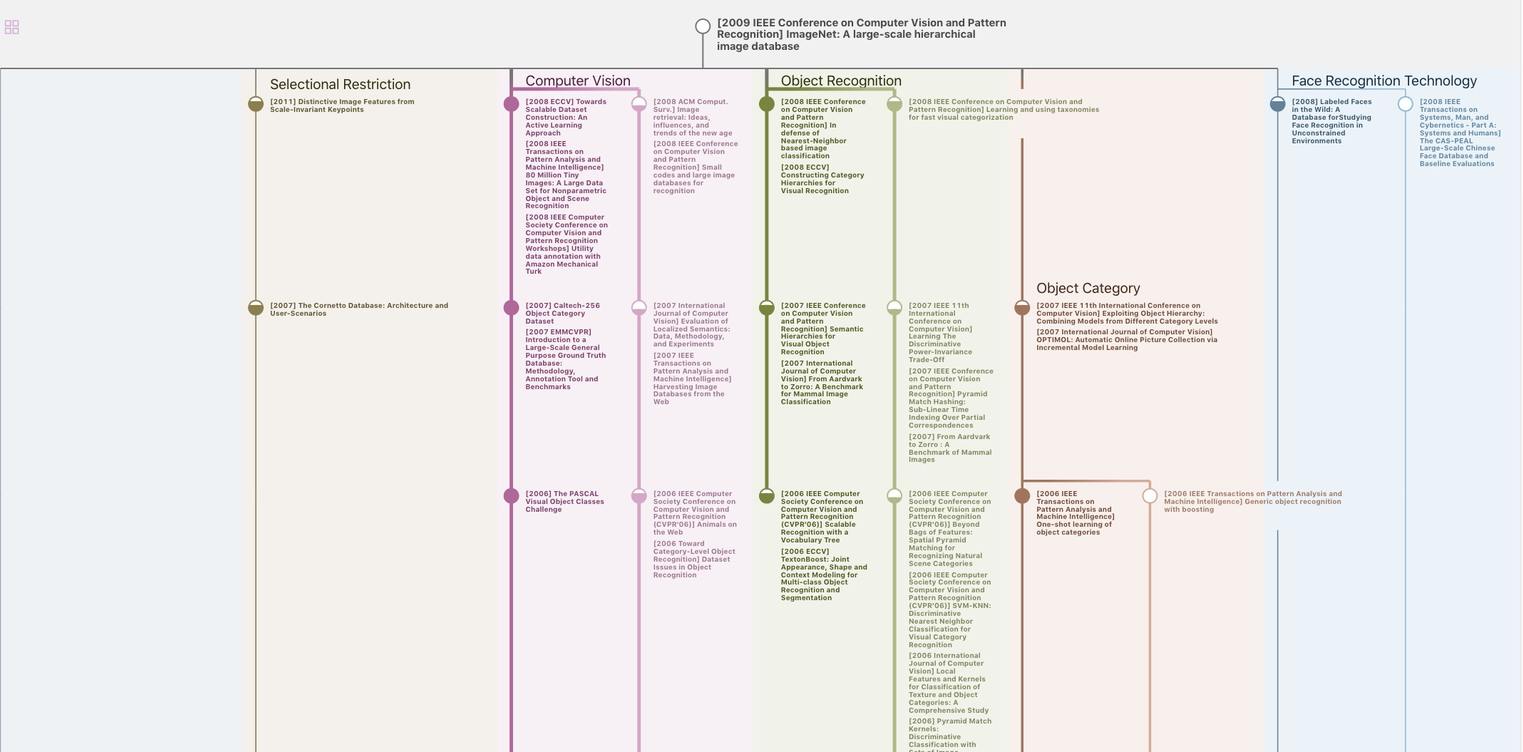
生成溯源树,研究论文发展脉络
Chat Paper
正在生成论文摘要