Delayed Feedback in Generalised Linear Bandits Revisited
arxiv(2023)
摘要
The stochastic generalised linear bandit is a well-understood model for sequential decision-making problems, with many algorithms achieving near-optimal regret guarantees under immediate feedback. However, the stringent requirement for immediate rewards is unmet in many real-world applications where the reward is almost always delayed. We study the phenomenon of delayed rewards in generalised linear bandits in a theoretical manner. We show that a natural adaptation of an optimistic algorithm to the delayed feedback achieves a regret bound where the penalty for the delays is independent of the horizon. This result significantly improves upon existing work, where the best known regret bound has the delay penalty increasing with the horizon. We verify our theoretical results through experiments on simulated data.
更多查看译文
关键词
generalised linear bandits,feedback
AI 理解论文
溯源树
样例
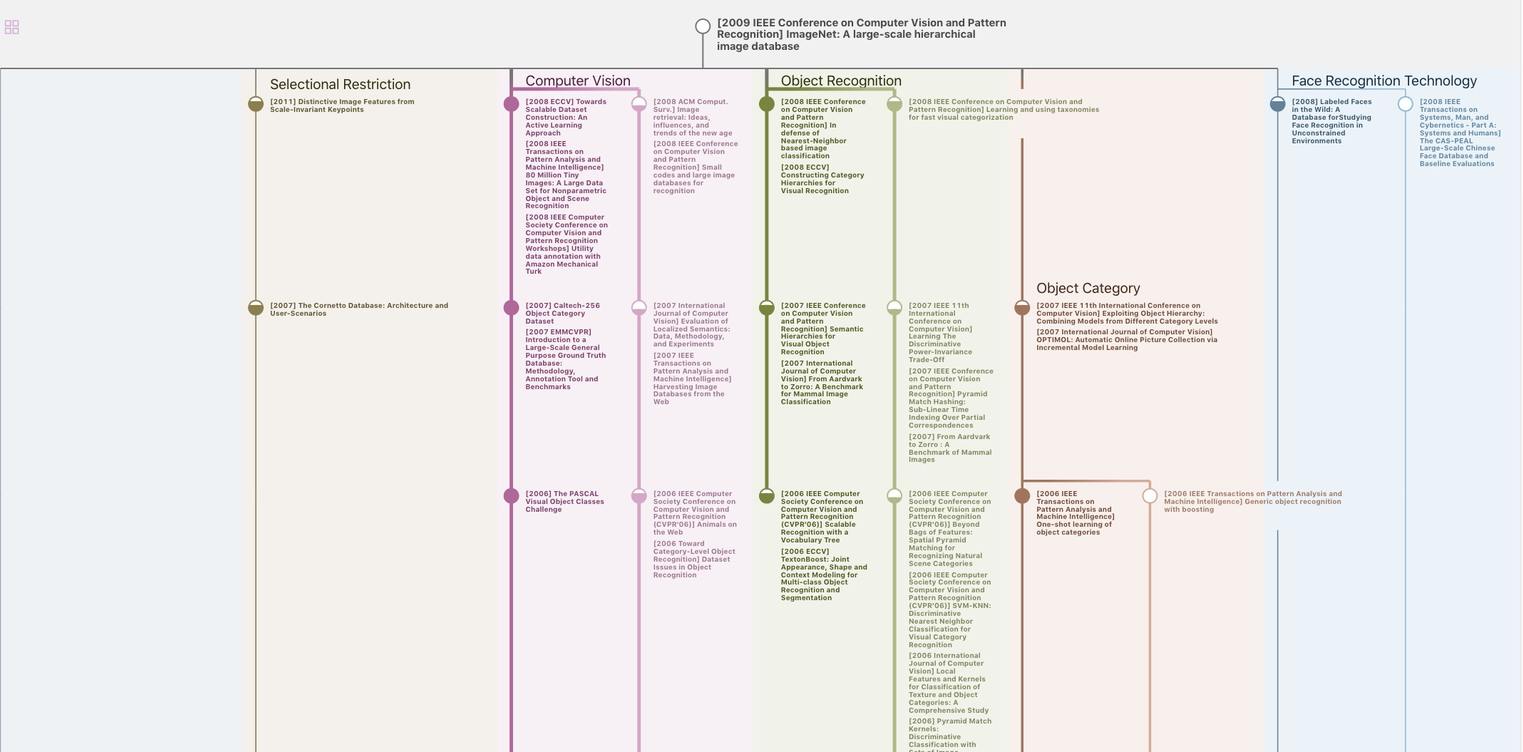
生成溯源树,研究论文发展脉络
Chat Paper
正在生成论文摘要