Wrht: Efficient All-reduce for Distributed DNN Training in Optical Interconnect Systems
arxiv(2023)
摘要
Communication efficiency is crucial for accelerating distributed deep neural network (DNN) training. All-reduce, a vital communication primitive, is responsible for reducing model parameters in distributed DNN training. However, most existing All-reduce algorithms, designed for traditional electrical interconnect systems, fall short due to bandwidth limitations. Optical interconnects, with superior bandwidth, low transmission delay, and less power consumption, emerge as viable alternatives. We propose Wrht (Wavelength Reused Hierarchical Tree), an efficient scheme for implementing the All-reduce operation in optical interconnect systems. Wrht leverages wavelength-division multiplexing (WDM) to minimize the communication time in distributed data-parallel DNN training. We calculate the required wavelengths, minimum communication steps, and optimal communication time, considering optical communication constraints. Simulations with real-world DNN models indicate that Wrht notably reduces communication time. On average, compared with three conventional All-reduce algorithms, Wrht achieves reductions of 65.23%, 43.81%, and 82.22% respectively in optical interconnect systems, and 61.23% and 55.51% compared with two algorithms in electrical systems. This highlights Wrht's potential to enhance communication efficiency in DNN training using optical interconnects.
更多查看译文
关键词
Optical interconnects,All-reduce,distributed training,DNN,WDM
AI 理解论文
溯源树
样例
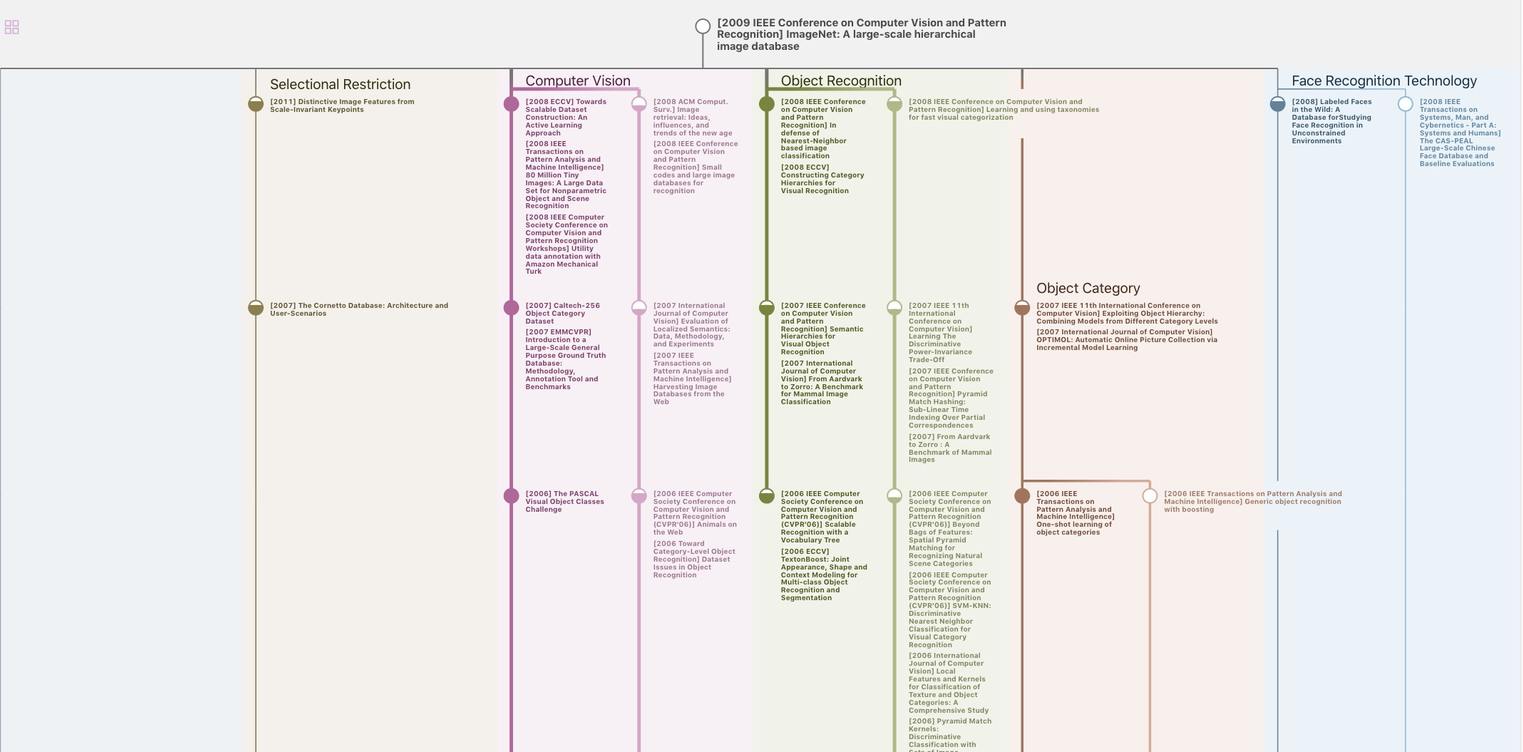
生成溯源树,研究论文发展脉络
Chat Paper
正在生成论文摘要