A Faster Quantum Algorithm for Semidefinite Programming via Robust IPM Framework
arxiv(2023)
摘要
This paper studies a fundamental problem in convex optimization, which is to solve semidefinite programming (SDP) with high accuracy. This paper follows from the existing robust SDP-based interior point method analysis due to [Huang, Jiang, Song, Tao and Zhang, FOCS 2022]. While, the previous work only provides an efficient implementation in the classical setting. This work provides a novel quantum implementation. We give a quantum second-order algorithm with high-accuracy in both the optimality and the feasibility of its output, and its running time depending on $\log(1/\epsilon)$ on well-conditioned instances. Due to the limitation of quantum itself or first-order method, all the existing quantum SDP solvers either have polynomial error dependence or low-accuracy in the feasibility.
更多查看译文
AI 理解论文
溯源树
样例
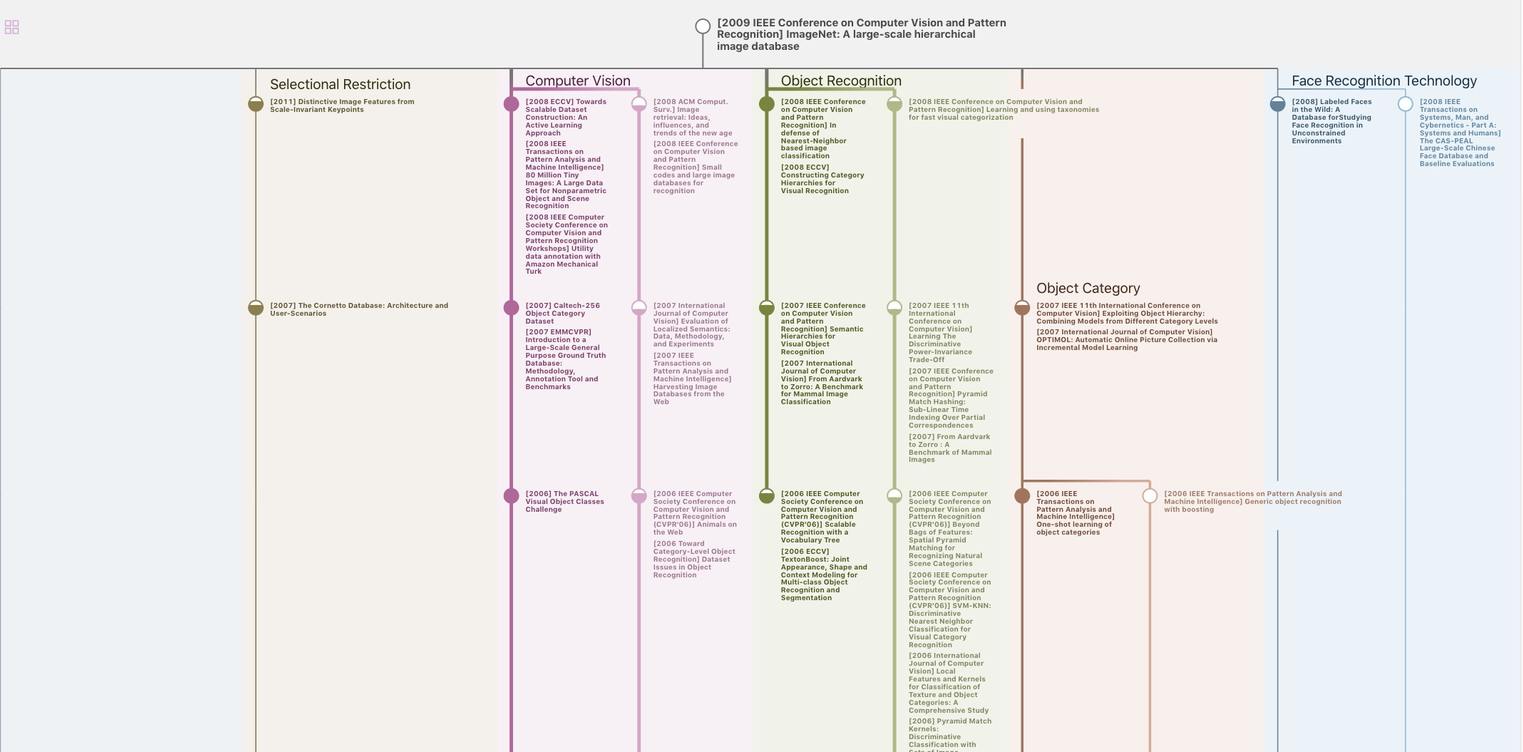
生成溯源树,研究论文发展脉络
Chat Paper
正在生成论文摘要