Too Many Relevants: Whither Cranfield Test Collections?
SIGIR '22: Proceedings of the 45th International ACM SIGIR Conference on Research and Development in Information Retrieval(2022)
摘要
This paper presents the lessons regarding the construction and use of large Cranfield-style test collections learned from the TREC 2021 Deep Learning track. The corpus used in the 2021 edition of the track was much bigger than the corpus used previously and it contains many more relevant documents. The process used to select documents to judge that had been used in earlier years of the track failed to produce a reliable collection because most topics have too many relevant documents. Judgment budgets were exceeded before an adequate sample of the relevant set could be found, so there are likely many unknown relevant documents in the unjudged portion of the corpus. As a result, the collection is not reusable, and furthermore, recall-based measures are unreliable even for the retrieval systems that were used to build the collection. Yet, early-precision measures cannot distinguish among system results because the maximum score is easily obtained for many topics. And since the existing tools for appraising the quality of test collections depend on systems' scores, they also fail when there are too many relevant documents. Collection builders will need new strategies and tools for building reliable test collections for continued use of the Cranfield paradigm on ever-larger corpora. Ensuring that the definition of 'relevant' truly reflects the desired systems' rankings is a provisional strategy for continued collection building.
更多查看译文
关键词
Cranfield, reusability, score saturation, test collections
AI 理解论文
溯源树
样例
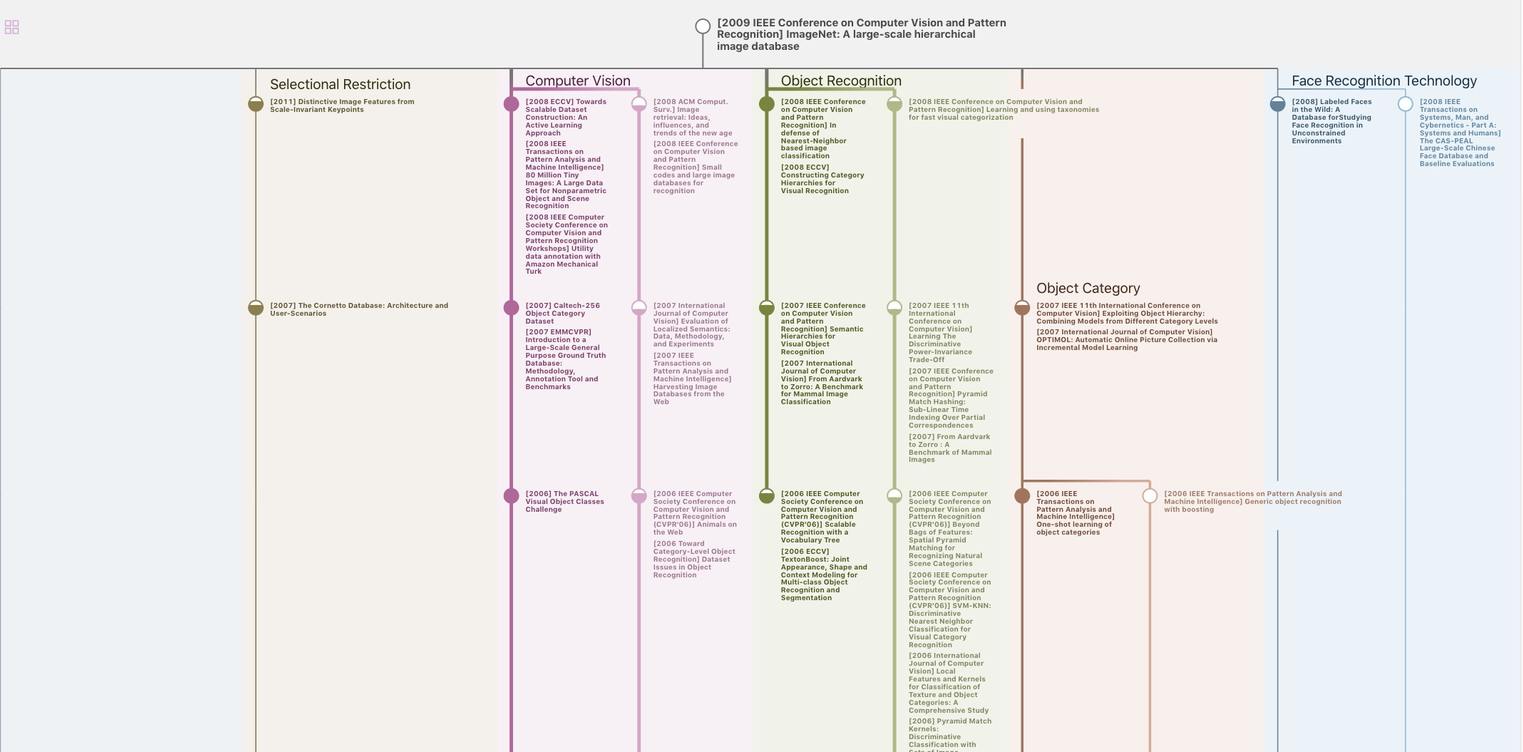
生成溯源树,研究论文发展脉络
Chat Paper
正在生成论文摘要