End-to-end Distantly Supervised Information Extraction with Retrieval Augmentation
SIGIR '22: Proceedings of the 45th International ACM SIGIR Conference on Research and Development in Information Retrieval(2022)
摘要
Distant supervision (DS) has been a prevalent approach to generating labeled data for information extraction (IE) tasks. However, DS often suffers from noisy label problems, where the labels are extracted from the knowledge base (KB), regardless of the input context. Many efforts have been devoted to designing denoising mechanisms. However, most strategies are only designed for one specific task and cannot be directly adapted to other tasks. We propose a general paradigm (Dasiera) to resolve issues in KB-based DS. Labels from KB can be viewed as universal labels of a target entity or an entity pair. While the given context for an IE task may only contain partial/zero information about the target entities, or the entailed information may be vague. Hence the mismatch between the given context and KB labels, i.e., the given context has insufficient information to infer DS labels, can happen in IE training datasets. To solve the problem, during training, Dasiera leverages a retrieval-augmentation mechanism to complete missing information of the given context, where we seamlessly integrate a neural retriever and a general predictor in an end-to-end framework. During inference, we can keep/remove the retrieval component based on whether we want to predict solely on the given context. We have evaluated Dasiera on two IE tasks under the DS setting: named entity typing and relation extraction. Experimental results show Dasiera's superiority to other baselines in both tasks.
更多查看译文
关键词
Information Extraction, Named Entity Typing, Relation Extraction, Distant Supervision, Retrieval Augmentation
AI 理解论文
溯源树
样例
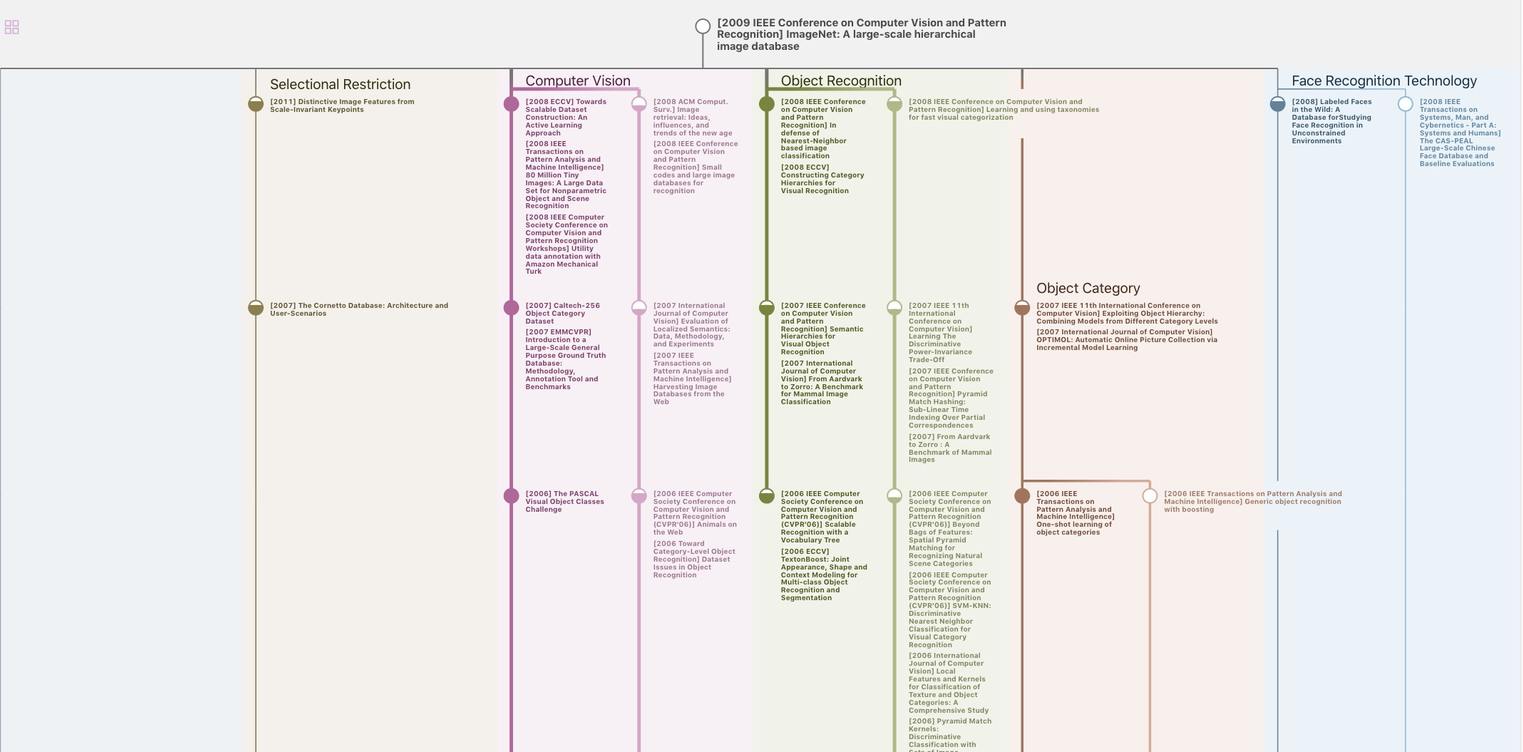
生成溯源树,研究论文发展脉络
Chat Paper
正在生成论文摘要