Comparing Few-Shot Learning with GPT-3 to Traditional Machine Learning Approaches for Classifying Teacher Simulation Responses
ARTIFICIAL INTELLIGENCE IN EDUCATION: POSTERS AND LATE BREAKING RESULTS, WORKSHOPS AND TUTORIALS, INDUSTRY AND INNOVATION TRACKS, PRACTITIONERS AND DOCTORAL CONSORTIUM, PT II(2022)
摘要
Teacher educators use digital clinical simulations (DCS) to provide improvisation opportunities within low-stakes classroom environments. In this study, we experimented with GPT-3 and few-shot learning to examine if it could be used with open-text DCS responses. We found that GPT-3 performed substantially worse than traditional machine learning (ML) models even on the same-sized training sets. However, the performance of GPT-3 decreased only marginally compared to traditional ML models with a training set of 20 examples (-0.06). Traditional ML models generally performed well and in some cases had similar performance to the human baseline. Future research will examine whether changes to labeling procedures or fine-tuning with existing data can improve the performance of GPT-3 with DCSs.
更多查看译文
关键词
Natural language processing,Few-shot learning,GPT-3,Simulations,Teacher education,Professional learning
AI 理解论文
溯源树
样例
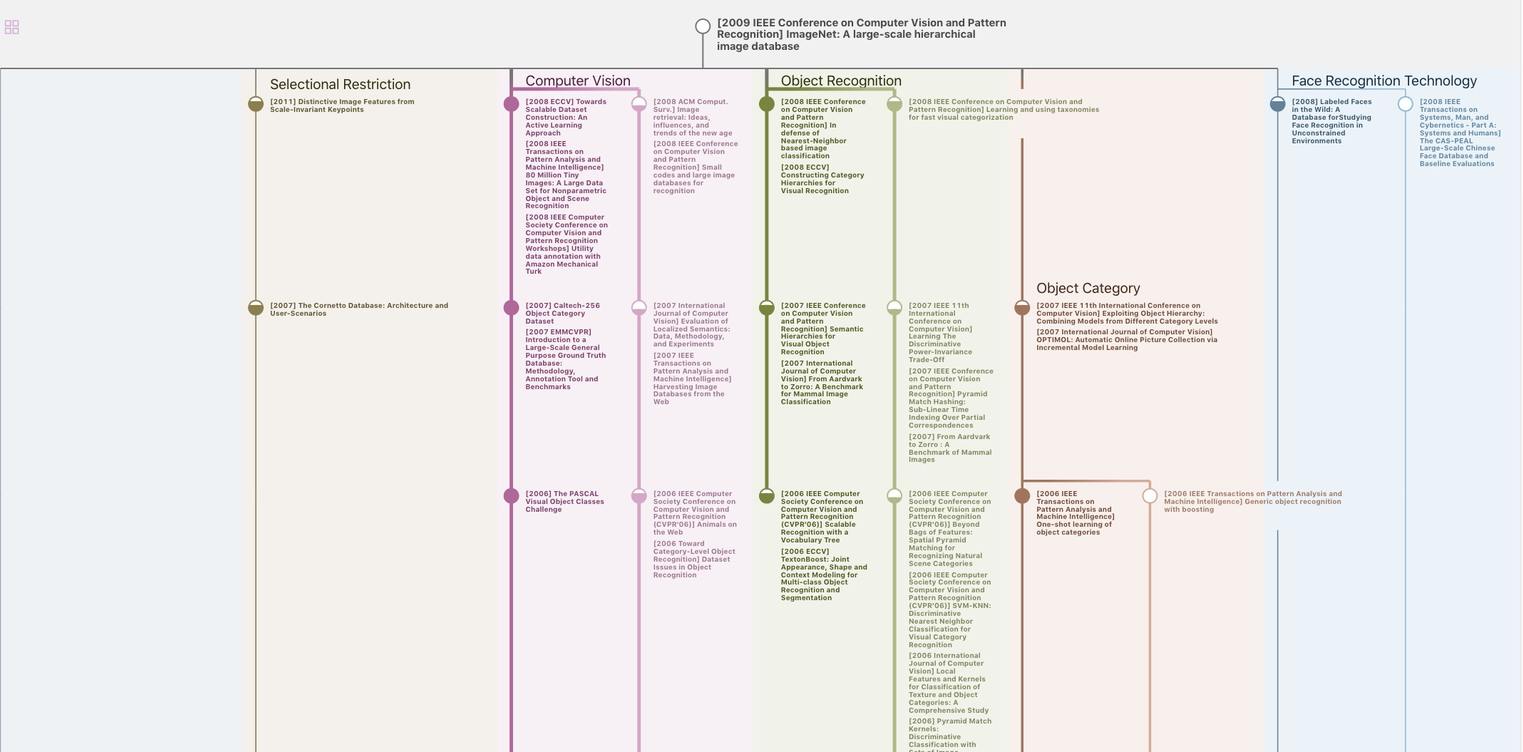
生成溯源树,研究论文发展脉络
Chat Paper
正在生成论文摘要