Bearing remaining useful life prediction of fatigue degradation process based on dynamic feature construction
INTERNATIONAL JOURNAL OF FATIGUE(2022)
摘要
In industry, the fatigue failure of bearings will lead to unexpected shutdown of mechanical equipment. Therefore, it is necessary to predict the remaining useful life (RUL) for guiding the maintenance process. The precision of prediction depends on the construction of degradation features. However, the selection and construction of feature set is complex and changeable, and the selection of degradation index is highly subjective. In the article, the bearing RUL prediction of fatigue degradation process based on dynamic features construction is proposed to solve these two problems. In this method, the degradation process is divided into two stages by the frequency domain analysis. The improved convolution neural network (CNN) by deep mutual learning (DML) is used to extract features automatically for the former stage. The prediction results of the latter stage can be achieved through the long short-term memory (LSTM) network. The average percentage error of prediction on the two data sets is 5.05%. The experimental results show the effectiveness of the proposed method.
更多查看译文
关键词
Fatigue degradation process,LSTM,RUL,Two stages
AI 理解论文
溯源树
样例
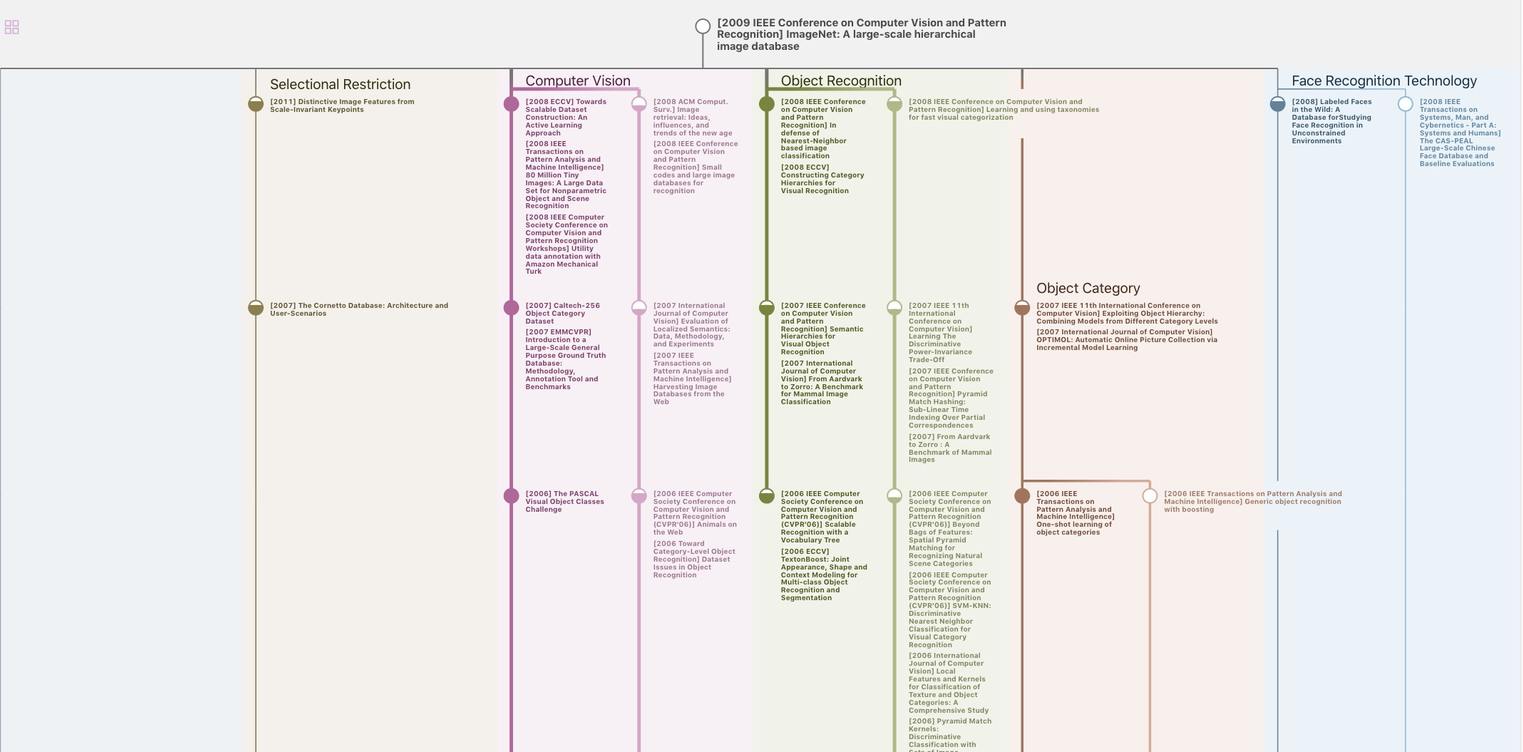
生成溯源树,研究论文发展脉络
Chat Paper
正在生成论文摘要