Learning an Adaptive Forwarding Strategy for Mobile Wireless Networks: Resource Usage vs. Latency
arxiv(2023)
摘要
Designing effective routing strategies for mobile wireless networks is challenging due to the need to seamlessly adapt routing behavior to spatially diverse and temporally changing network conditions. In this work, we use deep reinforcement learning (DeepRL) to learn a scalable and generalizable single-copy routing strategy for such networks. We make the following contributions: i) we design a reward function that enables the DeepRL agent to explicitly trade-off competing network goals, such as minimizing delay vs. the number of transmissions per packet; ii) we propose a novel set of relational neighborhood, path, and context features to characterize mobile wireless networks and model device mobility independently of a specific network topology; and iii) we use a flexible training approach that allows us to combine data from all packets and devices into a single offline centralized training set to train a single DeepRL agent. To evaluate generalizeability and scalability, we train our DeepRL agent on one mobile network scenario and then test it on other mobile scenarios, varying the number of devices and transmission ranges. Our results show our learned single-copy routing strategy outperforms all other strategies in terms of delay except for the optimal strategy, even on scenarios on which the DeepRL agent was not trained.
更多查看译文
关键词
adaptive forwarding strategy,mobile wireless networks
AI 理解论文
溯源树
样例
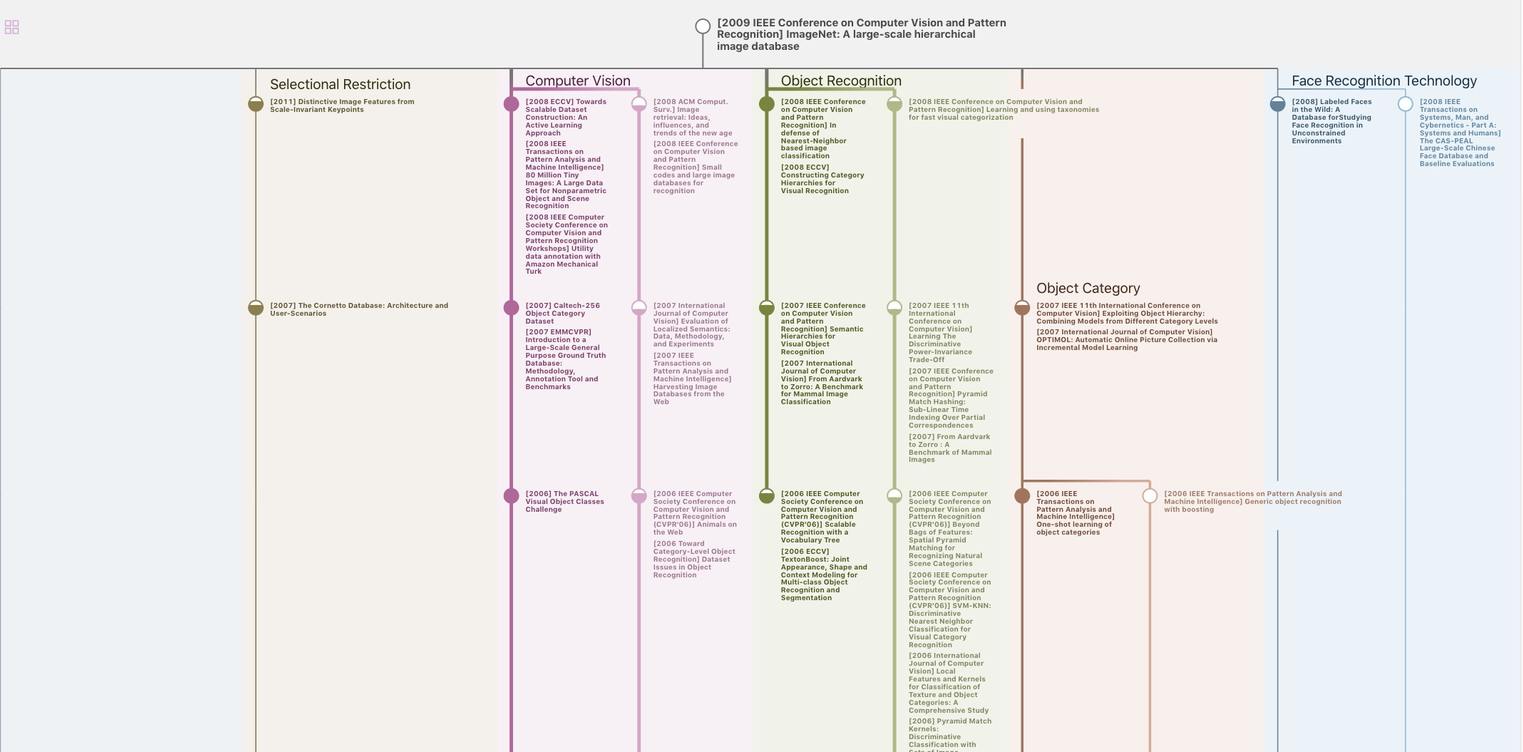
生成溯源树,研究论文发展脉络
Chat Paper
正在生成论文摘要