Minimax Rates for High-dimensional Double Sparse Structure over $\ell_q$-balls
arXiv (Cornell University)(2022)
摘要
In this paper, we focus on the high-dimensional double sparse structure, where the parameter of interest simultaneously encourages group-wise sparsity and element-wise sparsity in each group. Combining Gilbert-Varshamov bound and its variants, we develop a novel lower bound technique for the metric entropy of the parameter space, which is well suited for the double sparse structure over $\ell_q$-balls for $q \in [0,1]$. We prove the lower bounds on estimation error in an information theoretical manner, which is based on our proposed lower bound technique and Fano's inequality. The matching upper bounds are also established, whose proof follows from a direct analysis of the constrained least-squares estimators and results on empirical processes. Moreover, we extend the results over $\ell_q$-balls into the double sparse regression model and establish its minimax rate on the estimation error. Finally, we develop the DSIHT (Double Sparse Iterative Hard Thresholding) algorithm and show its optimality in the minimax sense for solving the double sparse linear regression.
更多查看译文
关键词
double,high-dimensional
AI 理解论文
溯源树
样例
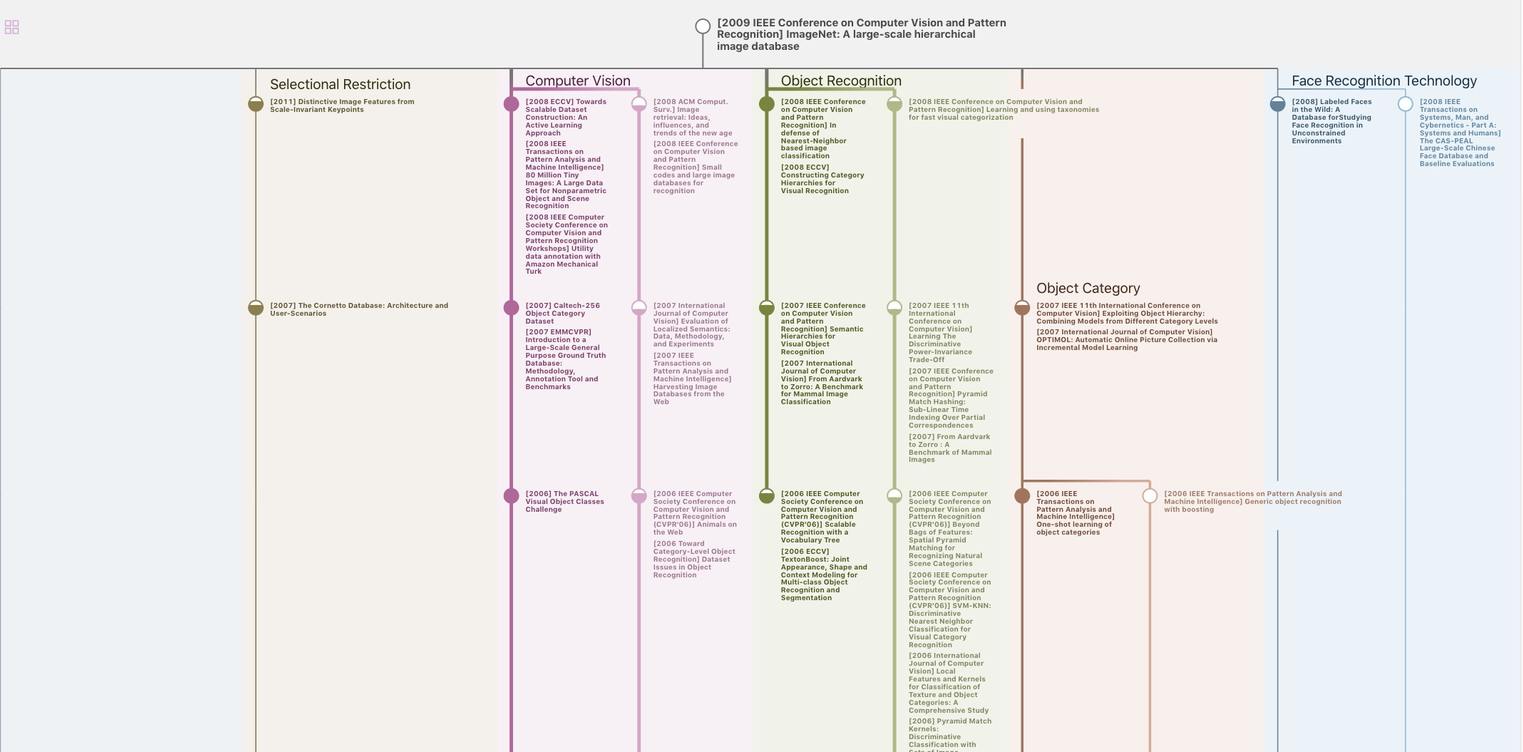
生成溯源树,研究论文发展脉络
Chat Paper
正在生成论文摘要