Online Reinforcement Learning in Periodic MDP
arXiv (Cornell University)(2023)
摘要
We study learning in periodic Markov Decision Process (MDP), a special type of non-stationary MDP where both the state transition probabilities and reward functions vary periodically, under the average reward maximization setting. We formulate the problem as a stationary MDP by augmenting the state space with the period index, and propose a periodic upper confidence bound reinforcement learning-2 (PUCRL2) algorithm. We show that the regret of PUCRL2 varies linearly with the period $N$ and as $\mathcal{O}(\sqrt{Tlog T})$ with the horizon length $T$. Utilizing the information about the sparsity of transition matrix of augmented MDP, we propose another algorithm PUCRLB which enhances upon PUCRL2, both in terms of regret ($O(\sqrt{N})$ dependency on period) and empirical performance. Finally, we propose two other algorithms U-PUCRL2 and U-PUCRLB for extended uncertainty in the environment in which the period is unknown but a set of candidate periods are known. Numerical results demonstrate the efficacy of all the algorithms.
更多查看译文
关键词
reinforcement learning,periodic mdp
AI 理解论文
溯源树
样例
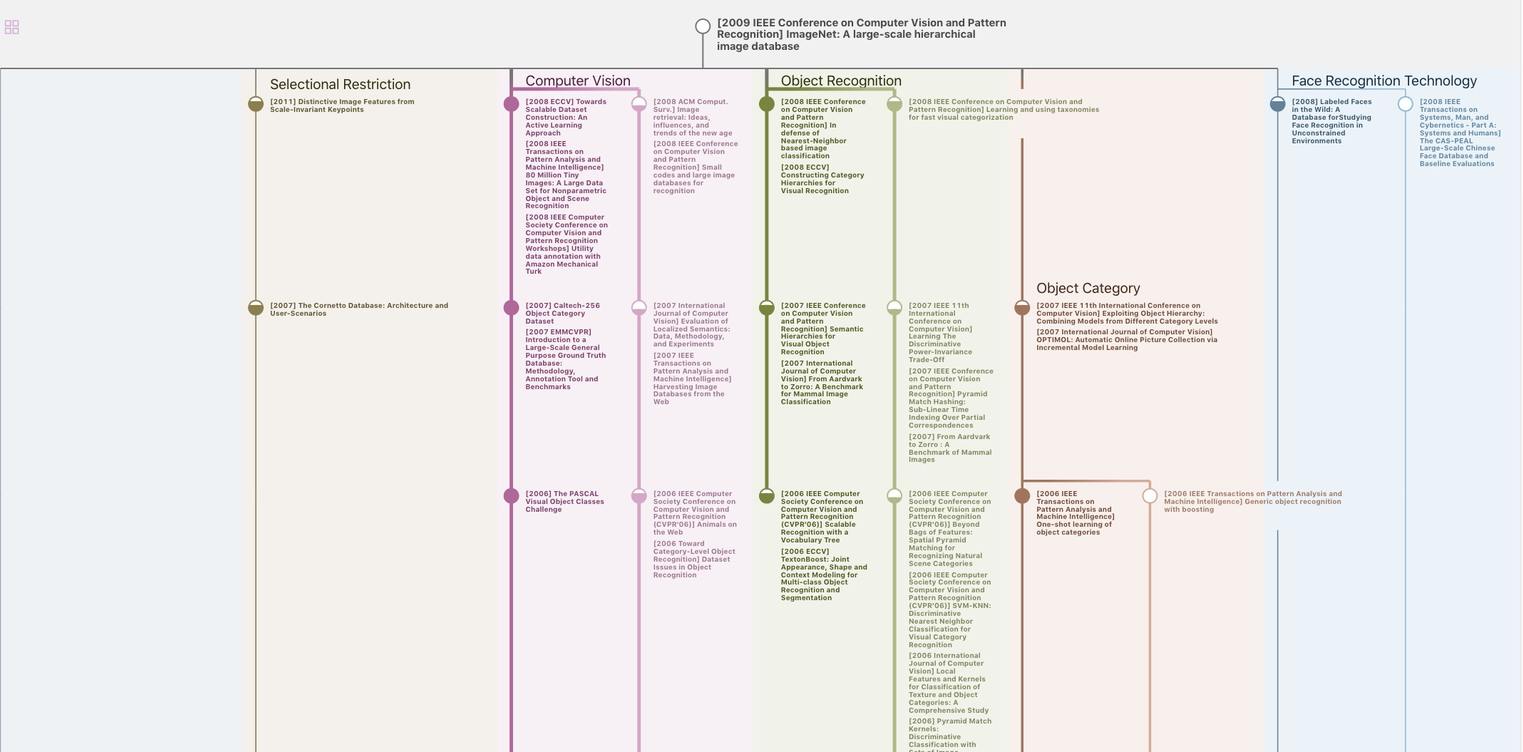
生成溯源树,研究论文发展脉络
Chat Paper
正在生成论文摘要