Adaptive Asynchronous Control Using Meta-Learned Neural Ordinary Differential Equations.
IEEE transactions on robotics(2024)
摘要
Model-based reinforcement learning and control have demonstrated great potential in various sequential decision making problem domains, including in robotics settings. However, real-world robotics systems often present challenges that limit the applicability of those methods. In particular, we note two problems that jointly happen in many industrial systems: first, irregular/asynchronous observations and actions and, second, dramatic changes in environment dynamics from an episode to another (e.g .$,$ varying payload inertial properties). We propose a general framework that overcomes those difficulties by meta-learning adaptive dynamics models for continuous-time prediction and control. The proposed approach is task-agnostic and can be adapted to new tasks in a straight-forward manner. We present evaluations in two different robot simulations and on a real industrial robot.
更多查看译文
关键词
Industrial robots,learning and adaptive systems,model learning for control,robust/adaptive control of robotic systems
AI 理解论文
溯源树
样例
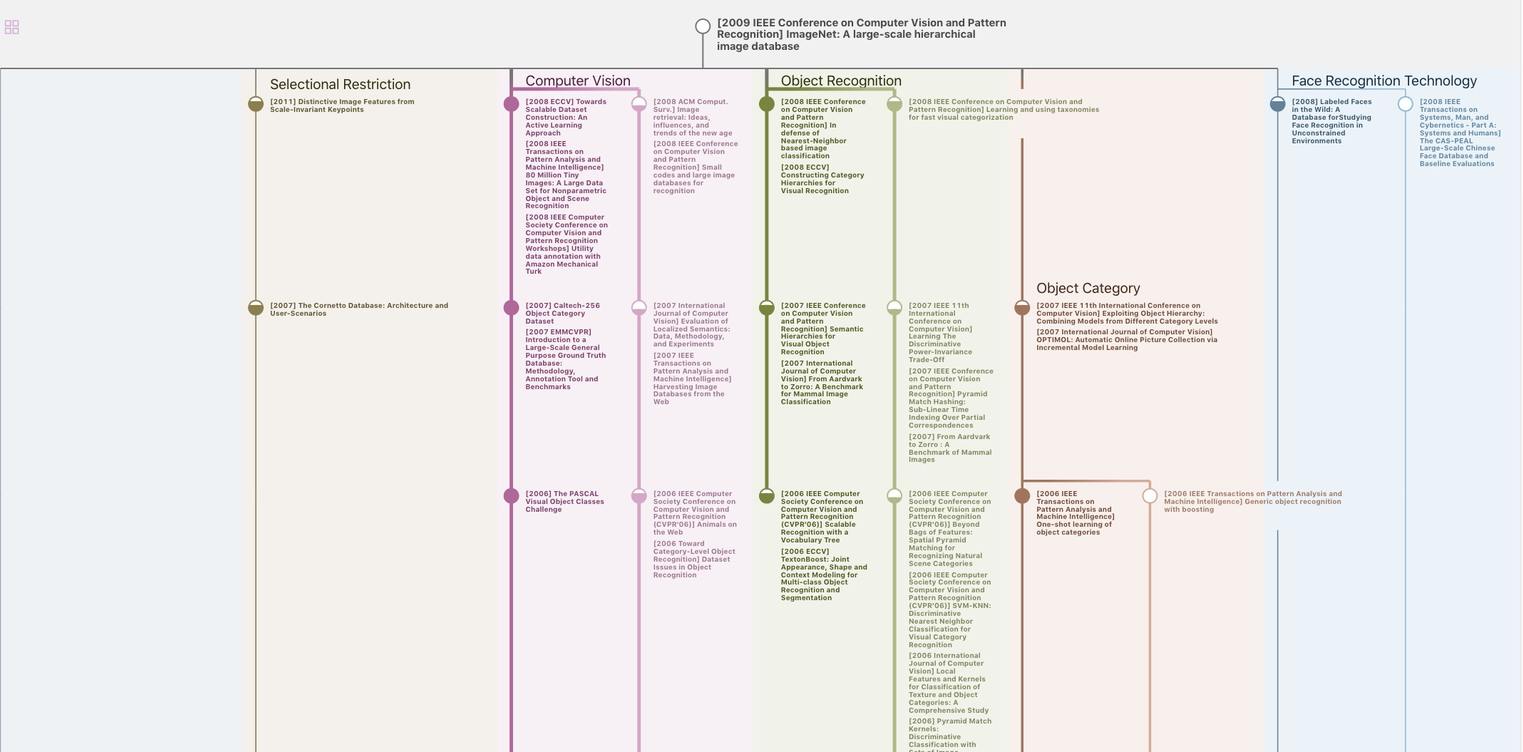
生成溯源树,研究论文发展脉络
Chat Paper
正在生成论文摘要