Modeling gene regulatory networks using neural network architectures
Nature Computational Science(2021)
摘要
Gene regulatory networks (GRNs) encode the complex molecular interactions that govern cell identity. Here we propose DeepSEM, a deep generative model that can jointly infer GRNs and biologically meaningful representation of single-cell RNA sequencing (scRNA-seq) data. In particular, we developed a neural network version of the structural equation model (SEM) to explicitly model the regulatory relationships among genes. Benchmark results show that DeepSEM achieves comparable or better performance on a variety of single-cell computational tasks, such as GRN inference, scRNA-seq data visualization, clustering and simulation, compared with the state-of-the-art methods. In addition, the gene regulations predicted by DeepSEM on cell-type marker genes in the mouse cortex can be validated by epigenetic data, which further demonstrates the accuracy and efficiency of our method. DeepSEM can provide a useful and powerful tool to analyze scRNA-seq data and infer a GRN. The authors propose a deep learning model that analyzes single-cell RNA sequencing (scRNA-seq) data by explicitly modeling gene regulatory networks (GRNs), outperforming the state-of-art methods on various tasks, including GRN inference, scRNA-seq analysis and simulation.
更多查看译文
关键词
Computational biology and bioinformatics,Gene regulatory networks,Machine learning,Computer Science,general
AI 理解论文
溯源树
样例
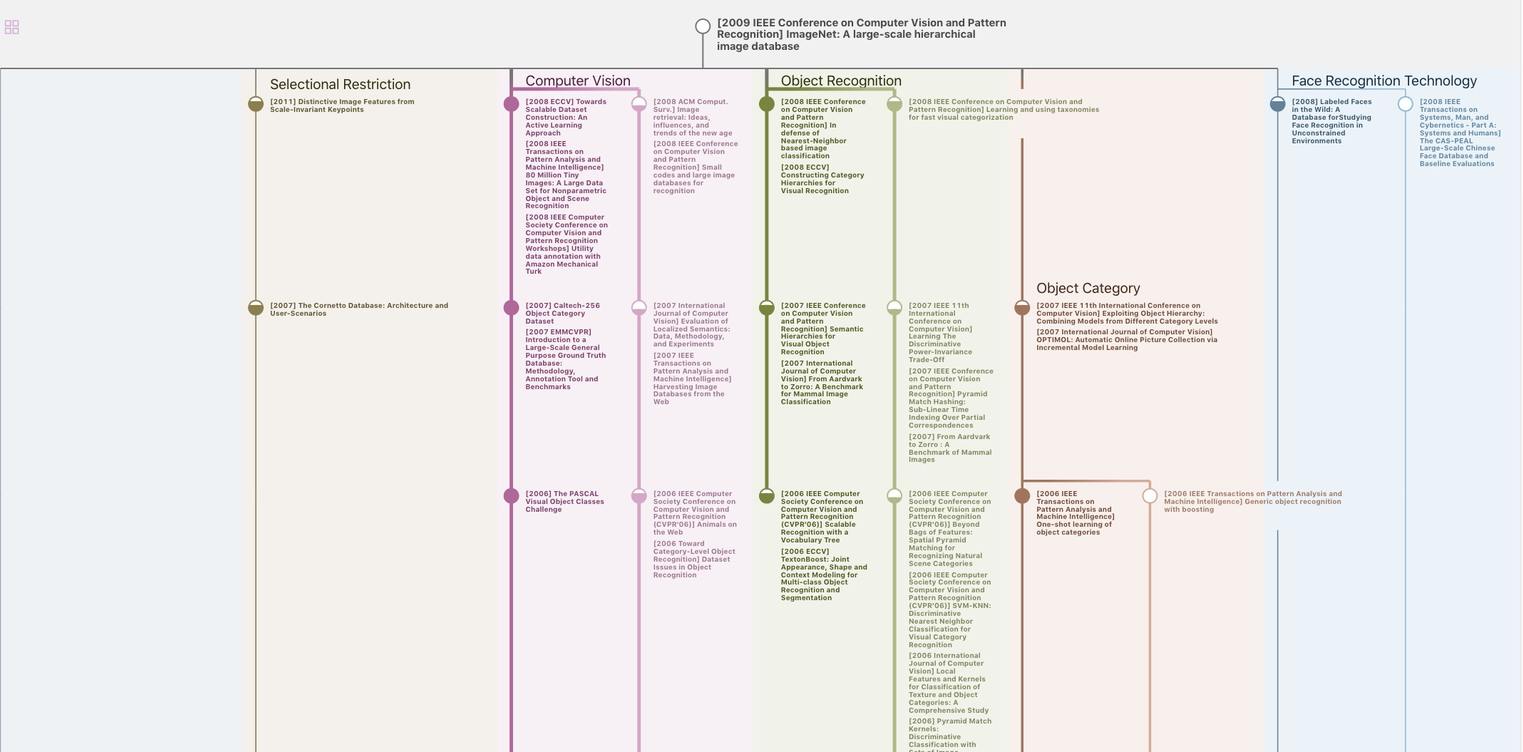
生成溯源树,研究论文发展脉络
Chat Paper
正在生成论文摘要