Multi-level adaptive few-shot learning network combined with vision transformer
Journal of Ambient Intelligence and Humanized Computing(2022)
摘要
Most few-shot learning methods usually focus on the improvement of the single high-level feature extractor, however, a lot of practices have proved that the low-level features also contain abundant visual information and play an important role in the learning of feature extractors. In this paper, we propose a multi-level adaptive vision transformer few-shot learning network (MLVT-FSL). First, we propose a two-branch feature extraction network, which employs the multi-level feature extractor and vision transformer to extract the multi-level features and obtain the global relationship. It can bring 1.16% improvement to the baseline model under the setting of 5-way 5-shot on the MiniImageNet dataset. Then we use a feature adjustment module (FAM) to adaptively adjust features to achieve task-specific and more discriminative embeddings, which can bring 0.65% improvement to our network under the 5-way 5-shot condition on the MiniImageNet dataset. To further evaluate the performance of MLVT-FSL, we conduct some extensive experiments on several standard few-shot classification benchmarks on MiniImageNet, TieredImageNet and the fine-grained dataset CUB-200. Particularly, the proposed MLVT-FSL can achieve 82.46% and 84.97% top-1 classification accuracy under the setting of 5-way 5-shot on the benchmark dataset MiniImageNet and TieredImageNet. In addition, MLVT-FSL can obtain 87.04% 5-way 5-shot classification accuracy on CUB-200. All these results verify the effectiveness and performance of the proposed model.
更多查看译文
关键词
Few-shot learning,Multi-level feature extractor,Vision transformer,Feature adjustment
AI 理解论文
溯源树
样例
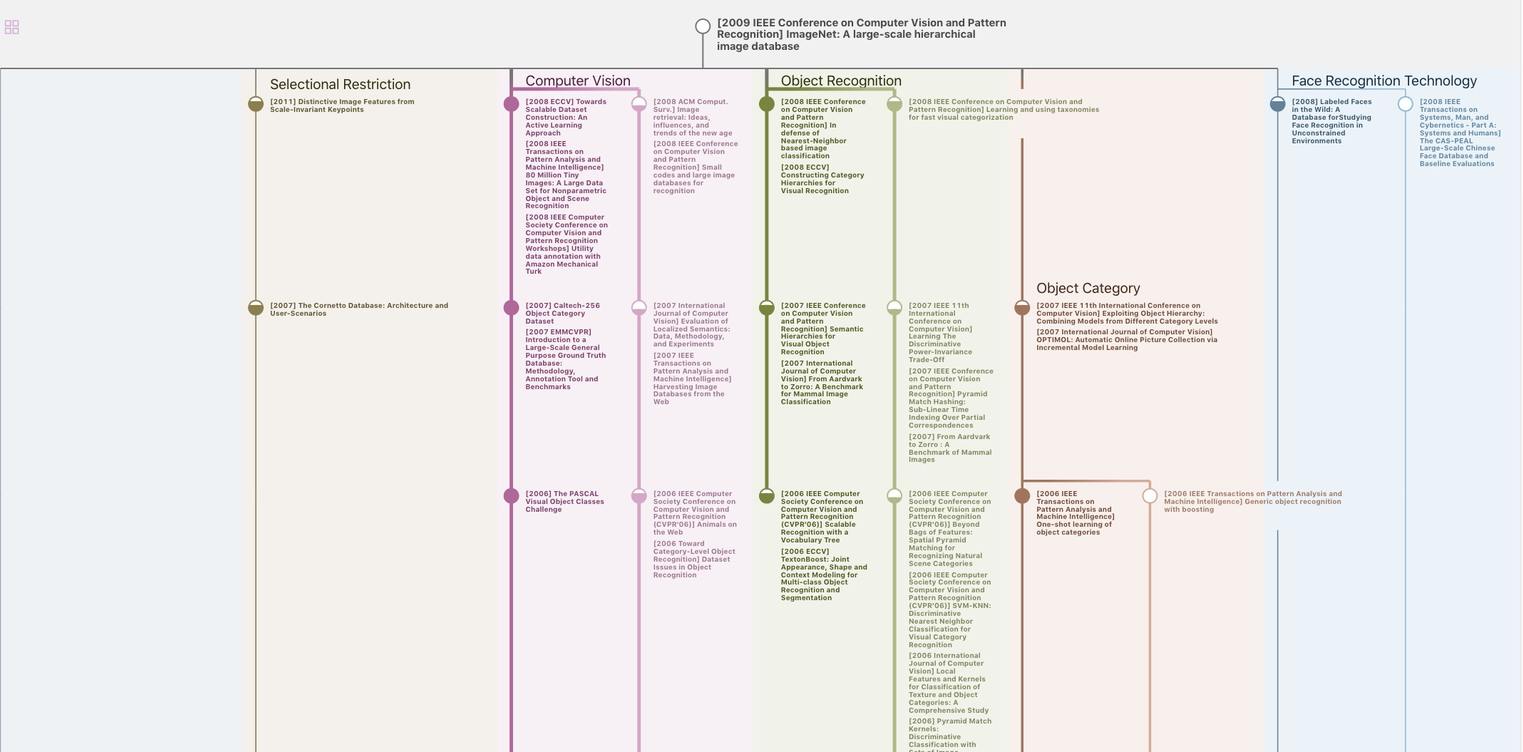
生成溯源树,研究论文发展脉络
Chat Paper
正在生成论文摘要