Forgery-Domain-Supervised Deepfake Detection With Non-Negative Constraint.
IEEE Signal Process. Lett.(2022)
摘要
Fake faces produced by deepfake techniques have attracted public concerns in recent years. Deepfake detection is a binary classification task that distinguishes fake faces from real ones. As the training data for deepfake detection is usually generated from real faces via various face forgery methods, it is difficult for a single binary decision boundary to distinguish fake faces. Besides that, the learned features often involve irrelevant information for identifying fake faces that are generated from diverse forgery methods. To deal with such challenges, unlike existing approaches that regard fake detection as a binary classification, we re-model the task as a multiclass forgery-domain classification task, where each forgery method is treated as a distinct class. This simplifies the complex decision boundary brought by the diversity of forgery patterns and provides more forgery-relevant information for the learning process. In addition, we introduce a non-negative constrained learning framework composed of non-negative features and a non-negative constrained classifier (NCC) to block irrelevant features with zero weights and enhance forgery-relevant features with positive weights, leading to a sparse structure of the classifier. Furthermore, to capture subtle and discriminative forgery-relevant features, we propose an integration module over augmented faces based on cross-attention. We demonstrate that our approach achieves competitive performance and generalization ability on widely-used benchmarks through extensive experiments.
更多查看译文
关键词
Faces, Forgery, Deepfakes, Task analysis, Feature extraction, Crops, Training, Classifier regularization, deepfake detection, face classification, face forensics, feature integration
AI 理解论文
溯源树
样例
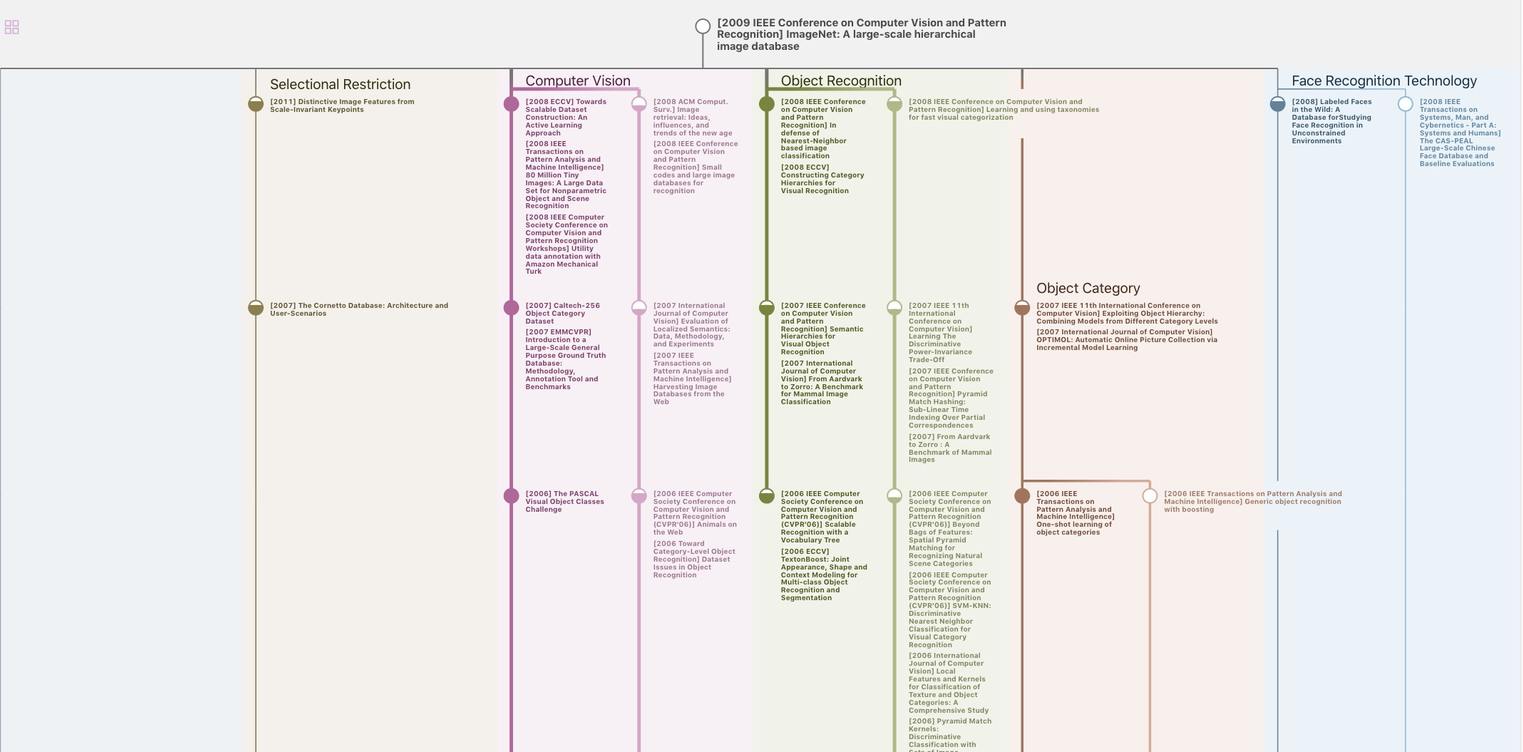
生成溯源树,研究论文发展脉络
Chat Paper
正在生成论文摘要