GCN and GRU Based Intelligent Model for Temperature Prediction of Local Heating Surfaces
IEEE Transactions on Industrial Informatics(2022)
摘要
A boiler heating surface is composed of hundreds of tubes, whose temperatures may be different because of their positions, the influences of attempering water, and flue gas. Using a criteria based on the Davies–Bouldin index, in this article, we propose to partition a heating surface into local ones, whose interactions in temperature are represented as a weighted heating surface graph (HSG) at each point of time, and whose current features are embedded in the HSG's nodes. Then, a local heating surface temperature prediction model based on weighted graph convolutional networks and gated recurrent units (WGCN-GRU), is proposed. Graph convolutional networks (GCNs) receive a series of HSGs, and extract the features of local heating surfaces and their spatial dependences in a time window. Features output by GCNs are finally directed to GRUs for temperature predictions. Experiments show that WGCN-GRU can averagely maintain the prediction error below 0.5 °C. Compared with other models, it can reduce the errors by a rate from 5.6% to 46.8%, and shows advantages in root-mean-squared error and
$R^{2}$
. It also shows that the node-to-node weights for the GCN can reduce the prediction error by 11.4%.
更多查看译文
关键词
Gated recurrent unit (GRU),graph convolutional network (GCN),heating surface,spatial-temporal features,temperature prediction
AI 理解论文
溯源树
样例
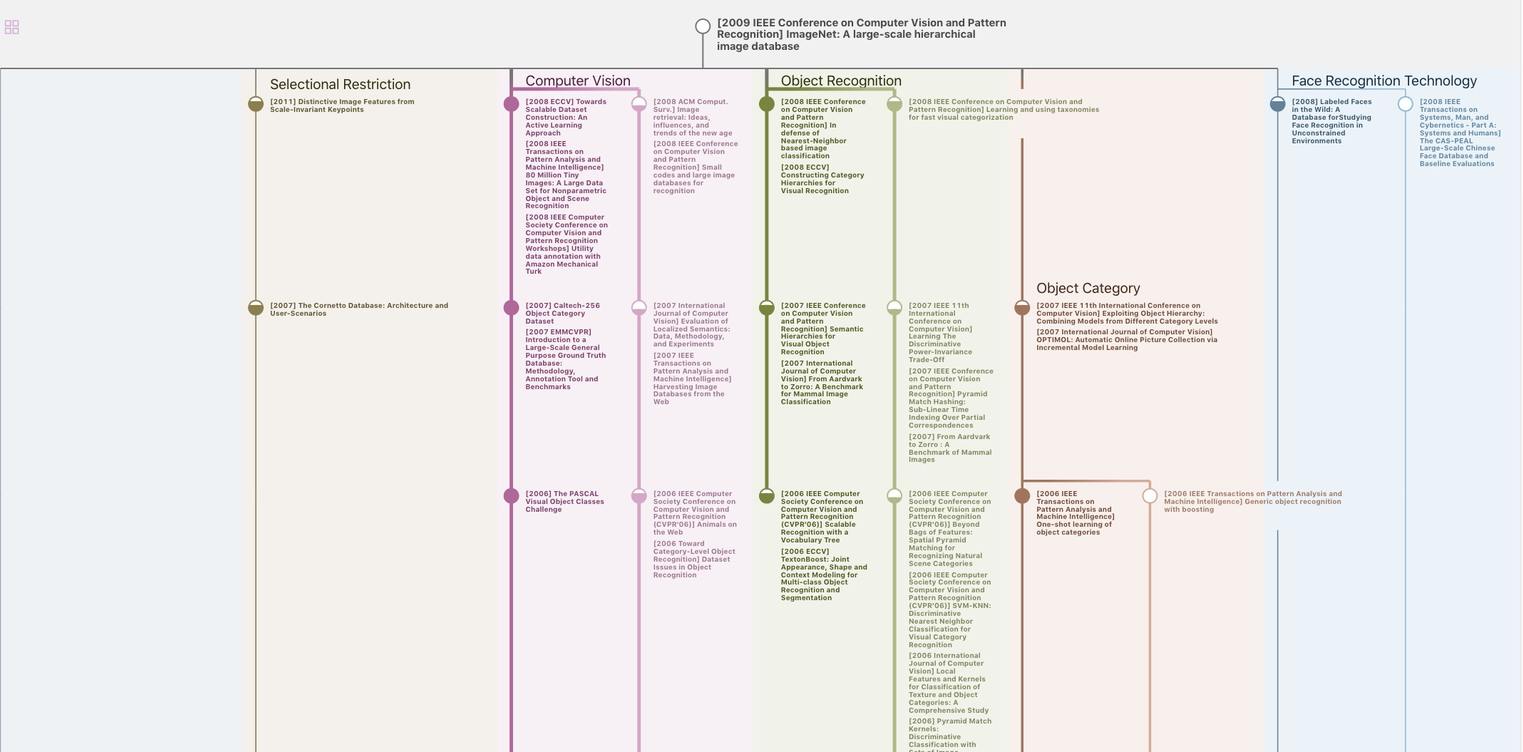
生成溯源树,研究论文发展脉络
Chat Paper
正在生成论文摘要