Sparse-Dyn: Sparse dynamic graph multirepresentation learning via event-based sparse temporal attention network
INTERNATIONAL JOURNAL OF INTELLIGENT SYSTEMS(2022)
摘要
Dynamic graph neural networks (DGNNs) have been widely used in modeling and representation learning of graph structure data. Current dynamic representation learning focuses on either discrete learning which results in temporal information loss, or continuous learning which involves heavy computation. In this study, we proposed a novel DGNN, sparse dynamic (Sparse-Dyn). It adaptively encodes temporal information into a sequence of patches with an equal amount of temporal-topological structure. Therefore, while avoiding using snapshots which cause information loss, it also achieves a finer time granularity, which is close to what continuous networks could provide. In addition, we also designed a lightweight module, Sparse Temporal Transformer, to compute node representations through structural neighborhoods and temporal dynamics. Since the fully connected attention conjunction is simplified, the computation cost is far lower than the current state-of-the-art. Link prediction experiments are conducted on both continuous and discrete graph data sets. By comparing several state-of-the-art graph embedding baselines, the experimental results demonstrate that Sparse-Dyn has a faster inference speed while having competitive performance.
更多查看译文
关键词
adaptive data encoding, dynamic graph neural network, link prediction, sparse temporal transformer
AI 理解论文
溯源树
样例
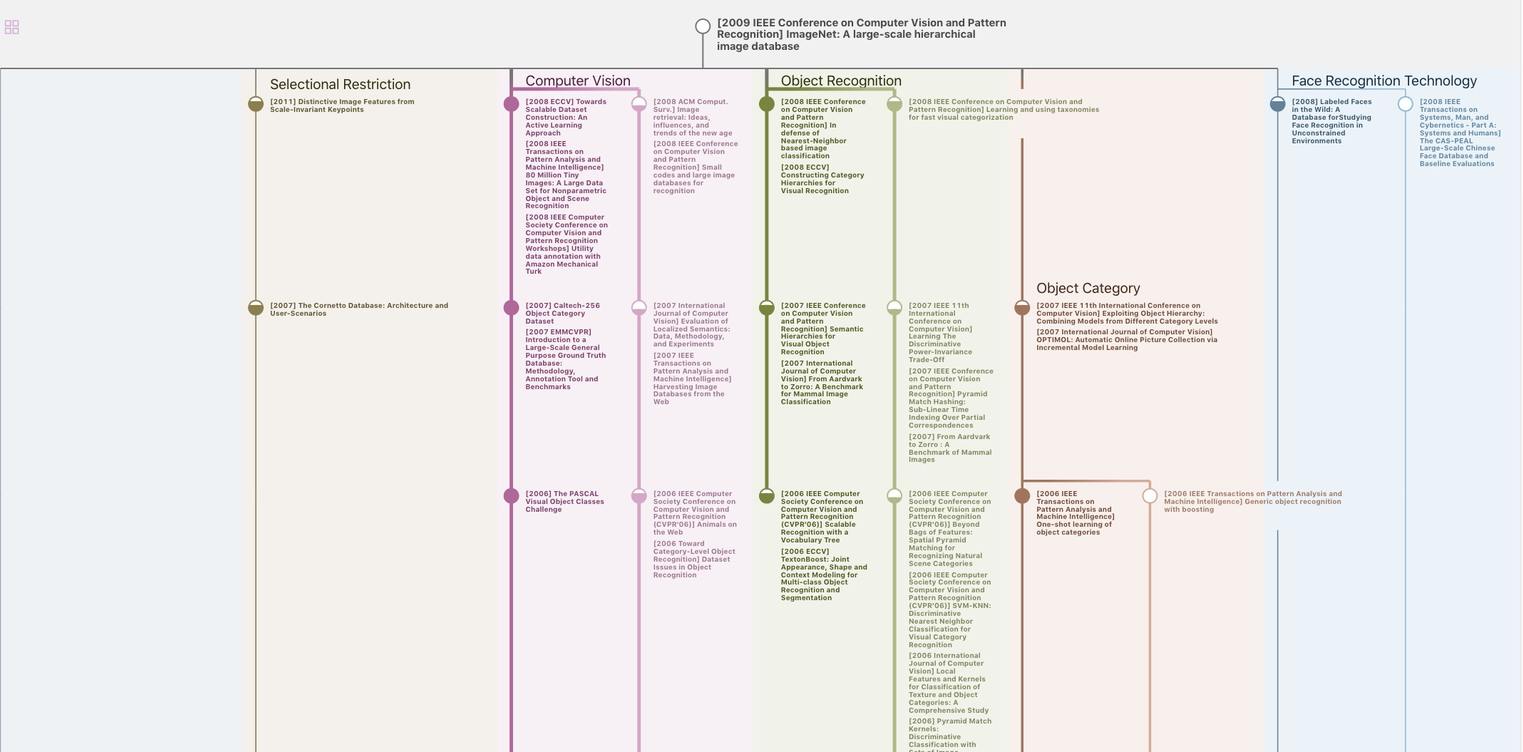
生成溯源树,研究论文发展脉络
Chat Paper
正在生成论文摘要