TSPNet: Translation supervised prototype network via residual learning for multimodal social relation extraction
Neurocomputing(2022)
摘要
Multimodal social relation extraction requires sufficient features fusion to identify the relation between different targets. Compared with traditional multimodal social relation extraction, there are many semantic gap issues for the few-shot scenario task, such as insufficient across-modality assistance, lacking explicit supervision, and unbalanced relations. To address the above problems, a novel Translation Supervised Prototype Network (TSPNet) is proposed, which extracts all the features of knowledge triples, not just relation features. First, the triple-level unimodal encoder learns textual and visual representation of knowledge triples from the entire information via two-stream encoding. Second, the triple-level multimodal extractor obtains multimodal knowledge triples by employing the residual learner to build the triple-level interaction across modalities. Finally, the intra-triple translation supervised decoder predicts the few-shot relations based on a prototype network supervised with the intra-triple translation as an explicit constraint. Our model achieves SOTA performance on three challenging benchmark datasets for few-shot multimodal social relation extraction, and further analysis shows that our model is effective and owns a strong generalization ability to avoid bias.
更多查看译文
关键词
Multimodal social relation,Knowledge triples,Few-shot scenario,Residual learning
AI 理解论文
溯源树
样例
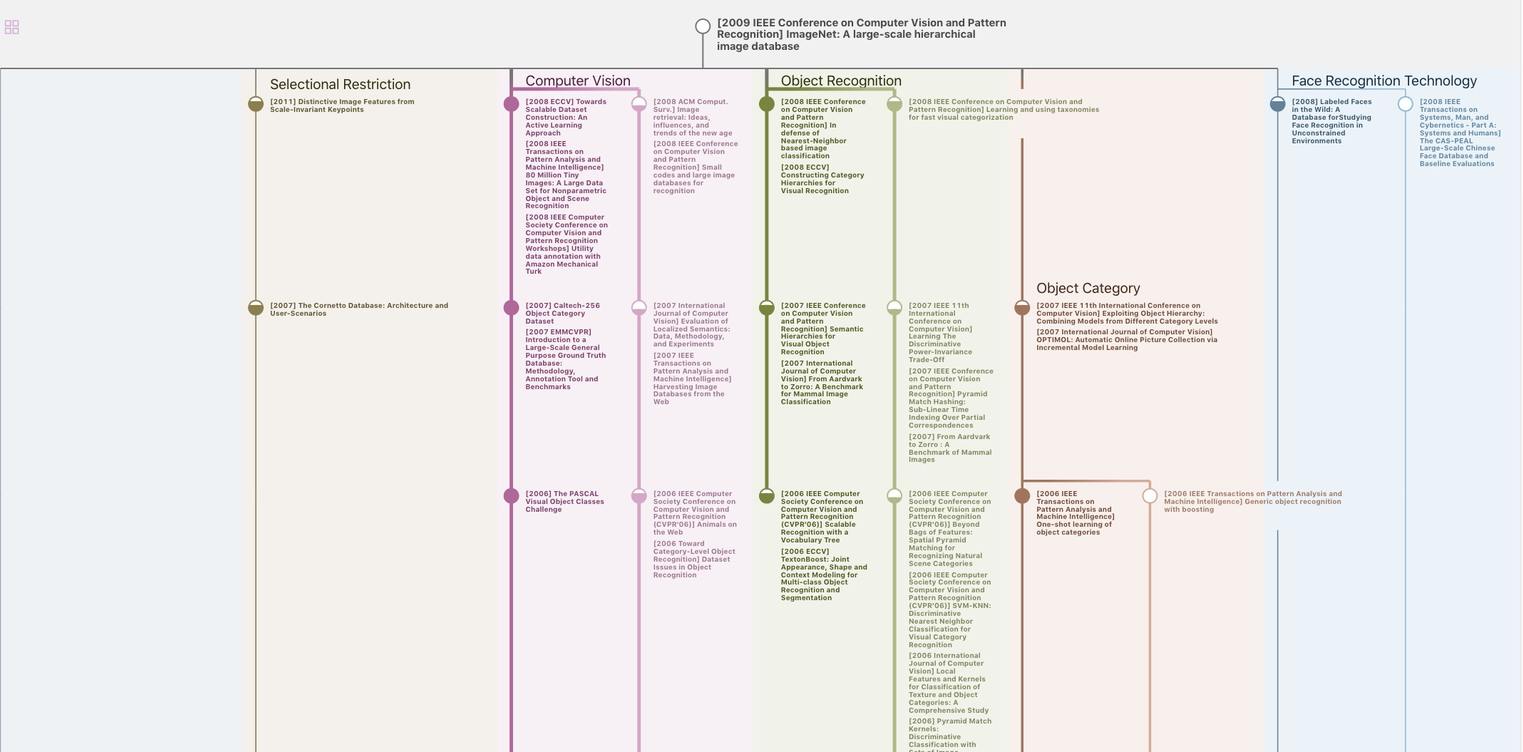
生成溯源树,研究论文发展脉络
Chat Paper
正在生成论文摘要