Causal predictive inference and target trial emulation
arxiv(2022)
摘要
Causal inference from observational data can be viewed as a missing data problem arising from a hypothetical population-scale randomized trial matched to the observational study. This links a target trial protocol with a corresponding generative predictive model for inference, providing a complete framework for transparent communication of causal assumptions and statistical uncertainty on treatment effects, without the need for counterfactuals. The intuitive foundation for the work is that a whole population randomized trial would provide answers to any observable causal question with certainty. Thus, our fundamental problem of causal inference is the missingness of the hypothetical target trial data, which we solve through repeated imputation from a generative predictive model conditioned on the observational data. Causal assumptions map to intuitive conditions on the transportability of predictive models across populations and conditions. We demonstrate our approach on a real data application to studying the effects of maternal smoking on birthweights using extensions of Bayesian additive regression trees and inverse probability weighting.
更多查看译文
关键词
causal predictive inference,trial emulation,target
AI 理解论文
溯源树
样例
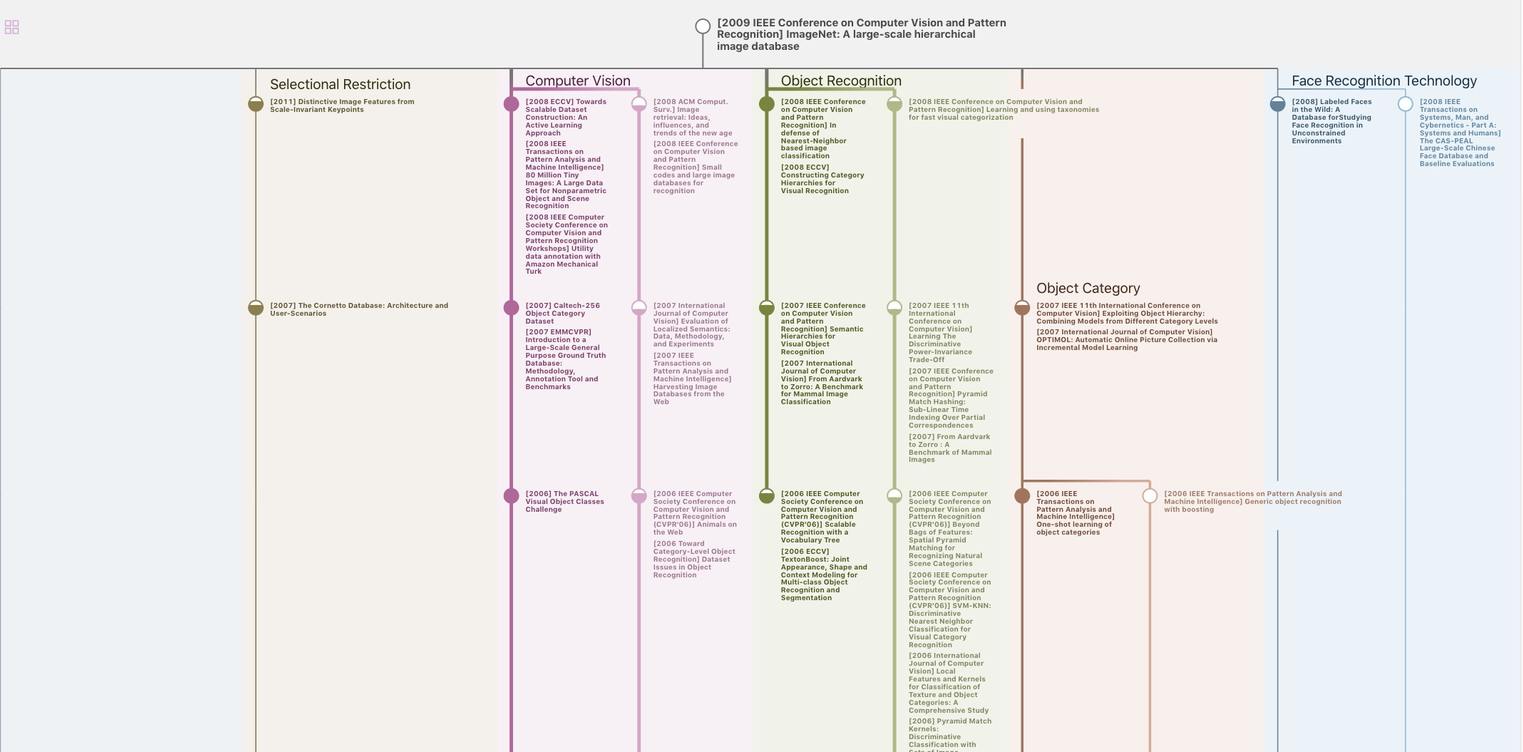
生成溯源树,研究论文发展脉络
Chat Paper
正在生成论文摘要