Exploring Fine-Grained Sparsity in Convolutional Neural Networks for Efficient Inference
IEEE Transactions on Pattern Analysis and Machine Intelligence(2023)
摘要
Neural networks contain considerable redundant computation, which drags down the inference efficiency and hinders the deployment on resource-limited devices. In this paper, we study the sparsity in convolutional neural networks and propose a generic sparse mask mechanism to improve the inference efficiency of networks. Specifically, sparse masks are learned in both data and channel dimensions to dynamically localize and skip redundant computation at a fine-grained level. Based on our sparse mask mechanism, we develop SMPointSeg, SMSR, and SMStereo for point cloud semantic segmentation, single image super-resolution, and stereo matching tasks, respectively. It is demonstrated that our sparse masks are well compatible to different model components and network architectures to accurately localize redundant computation, with computational cost being significantly reduced for practical speedup. Extensive experiments show that our SMPointSeg, SMSR, and SMStereo achieve state-of-the-art performance on benchmark datasets in terms of both accuracy and efficiency.
更多查看译文
关键词
Neural network,efficient inference,point cloud,semantic segmentation,image super-resolution,stereo matching
AI 理解论文
溯源树
样例
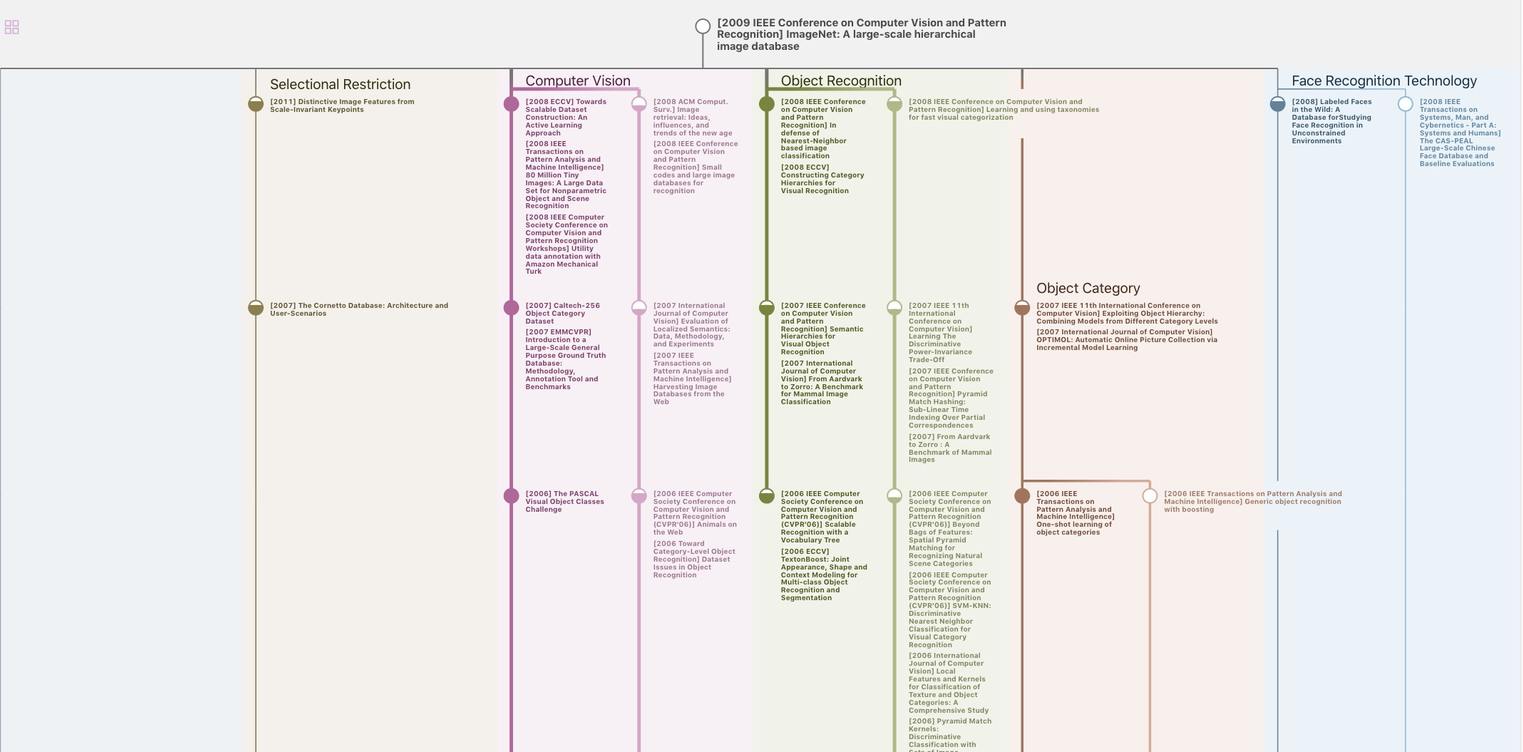
生成溯源树,研究论文发展脉络
Chat Paper
正在生成论文摘要