Optimizing Training and Transmission Overheads for Secure URLLC Against Randomly Distributed Eavesdroppers
IEEE Transactions on Vehicular Technology(2022)
摘要
In this article, we focus on the pilot-assisted transmission design for secure ultra-reliable and low-latency communication (URLLC) systems, which has not yet been studied in the existing literatures. In each transmission period of this system, the multi-antenna controller sends confidential URLLC messages integrated with artificial noise (AN) to a single-antenna actuator for some mission-critical operations (e.g., remote control), and while an unknown number of passive eavesdroppers attempt to intercept this confidential message in a non-colluding manner. Since the conventional ergodic secrecy capacity is not accurate to describe the maximal achievable secrecy rate of URLLC with short blocklength coding, we establish the concept of achievable effective secrecy rate (AESR) to measure the secrecy performance of our URLLC system, which is defined as the average number of information bits can be transmitted per channel use under given reliability and information leakage constraints. Due to the exact expression of AESR has an intractable form, we derive a closed-form approximation on the AESR to simplify the calculation, via leveraging the Laplace approximation and Gaussian-Chebyshev quadrature method. Following by this approximation, we propose an efficient two-dimensional search algorithm to determine the optimal transmission parameters that maximize the AESR, i.e., the training pilot length and the power allocation ratio between confidential data signals and AN. Furthermore, the asymptotic performance of AESR in the high-SNR regime is also analyzed to gain more insights. Numerical results validate the accuracy of theoretical approximations presented in this paper, and illustrate how the particular system parameters impact the AESR of our considered URLLC system.
更多查看译文
关键词
Artificial noise,channel training,physical-layer security,stochastic geometry,URLLC
AI 理解论文
溯源树
样例
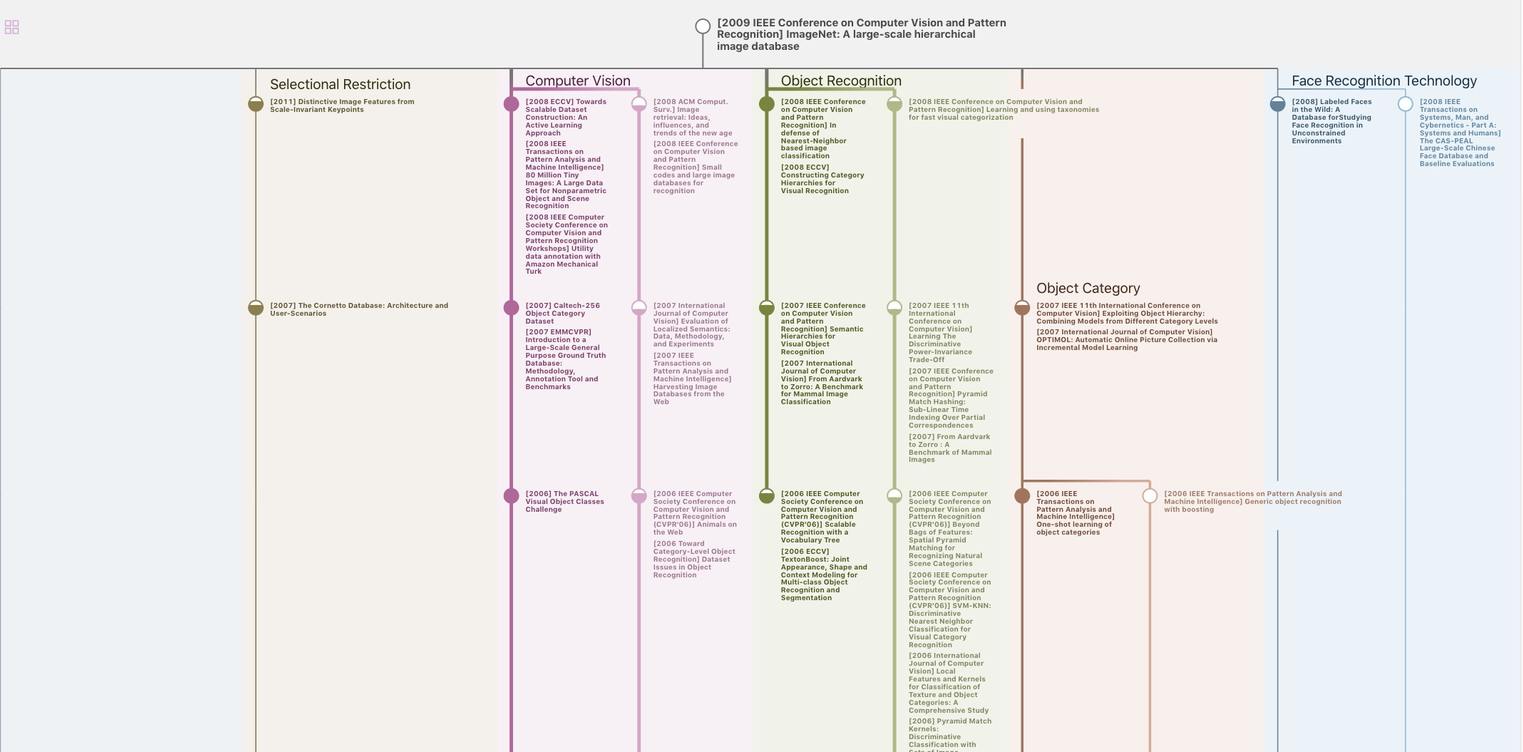
生成溯源树,研究论文发展脉络
Chat Paper
正在生成论文摘要