Enhancing Diversity by Local Subset Selection in Evolutionary Multiobjective Optimization
IEEE transactions on evolutionary computation(2023)
摘要
The main target of multiobjective evolutionary algorithms (MOEAs) is to find a set of evenly distributed nondominated solutions that approximate the Pareto front (PF) of a multiobjective optimization problem (MOP). This means that the approximated set should be as close to the PF as possible, and as diverse as possible. The former is usually called a convergence criterion and the latter is called a diversity criterion. A variety of strategies have been proposed to meet the two criteria. However, as far as the diversity criterion is concerned, it is still a challenge to achieve an evenly distributed approximation set with different sizes for a problem with a complicated PF shape. To deal with this challenge, we propose a local subset selection (LSS) -based environmental selection for evolutionary multiobjective optimization in this article. LSS considers the environmental selection as a subset selection problem by choosing promising solutions from the combination of the parent and offspring populations. In LSS, a potential energy function is utilized as the objective function, which provides a heavy selection pressure on diversity as well as has low computational complexity. Furthermore, to balance search efficiency and quality, a local search strategy is used in LSS to make full use of objective information for acceleration. The proposed LSS strategy is embedded into some state-of-the-art Pareto-domination-based MOEAs, and the experimental results suggest that LSS can produce shape-invariant and evenly distributed nondominated sets with different population sizes.
更多查看译文
关键词
algorithm,local search,multiobjective optimization,potential energy function,subset selection
AI 理解论文
溯源树
样例
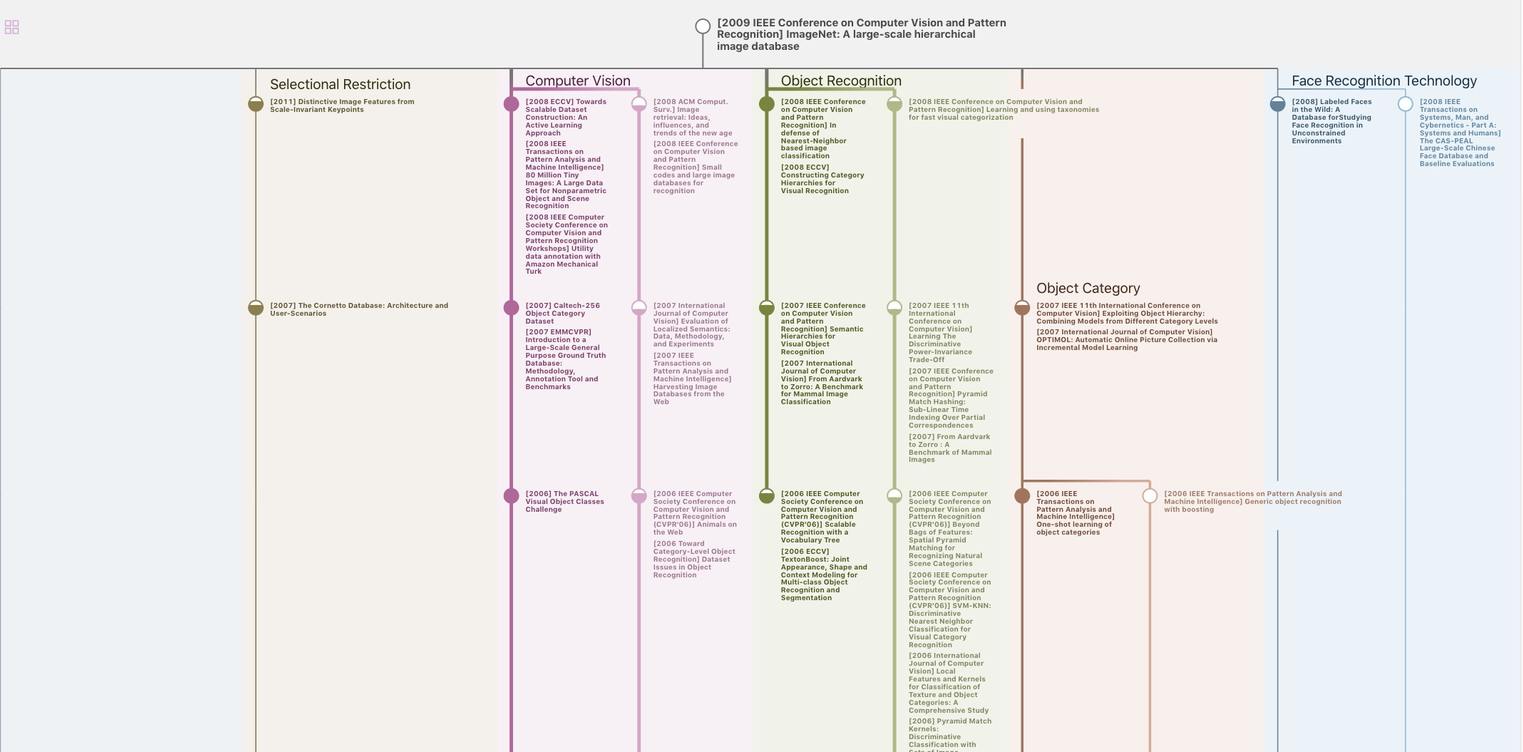
生成溯源树,研究论文发展脉络
Chat Paper
正在生成论文摘要