DynaMarks: Defending Against Deep Learning Model Extraction Using Dynamic Watermarking
arxiv(2022)
摘要
The functionality of a deep learning (DL) model can be stolen via model extraction where an attacker obtains a surrogate model by utilizing the responses from a prediction API of the original model. In this work, we propose a novel watermarking technique called DynaMarks to protect the intellectual property (IP) of DL models against such model extraction attacks in a black-box setting. Unlike existing approaches, DynaMarks does not alter the training process of the original model but rather embeds watermark into a surrogate model by dynamically changing the output responses from the original model prediction API based on certain secret parameters at inference runtime. The experimental outcomes on Fashion MNIST, CIFAR-10, and ImageNet datasets demonstrate the efficacy of DynaMarks scheme to watermark surrogate models while preserving the accuracies of the original models deployed in edge devices. In addition, we also perform experiments to evaluate the robustness of DynaMarks against various watermark removal strategies, thus allowing a DL model owner to reliably prove model ownership.
更多查看译文
关键词
deep learning model extraction,deep learning,dynamarks
AI 理解论文
溯源树
样例
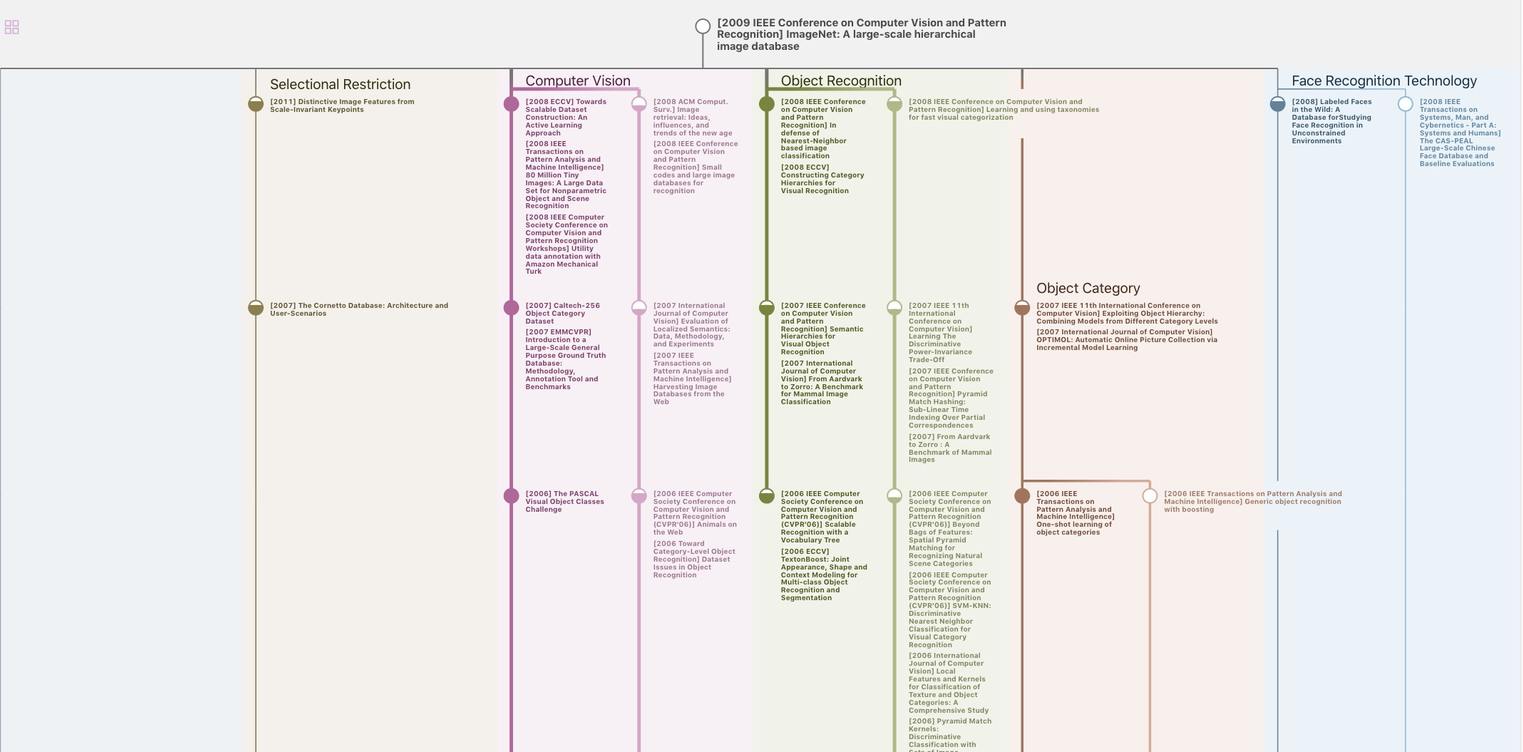
生成溯源树,研究论文发展脉络
Chat Paper
正在生成论文摘要