Structural Similarity for Improved Transfer in Reinforcement Learning
arxiv(2022)
摘要
Transfer learning is an increasingly common approach for developing performant RL agents. However, it is not well understood how to define the relationship between the source and target tasks, and how this relationship contributes to successful transfer. We present an algorithm called Structural Similarity for Two MDPS, or SS2, that calculates a state similarity measure for states in two finite MDPs based on previously developed bisimulation metrics, and show that the measure satisfies properties of a distance metric. Then, through empirical results with GridWorld navigation tasks, we provide evidence that the distance measure can be used to improve transfer performance for Q-Learning agents over previous implementations.
更多查看译文
关键词
reinforcement learning,improved transfer,similarity
AI 理解论文
溯源树
样例
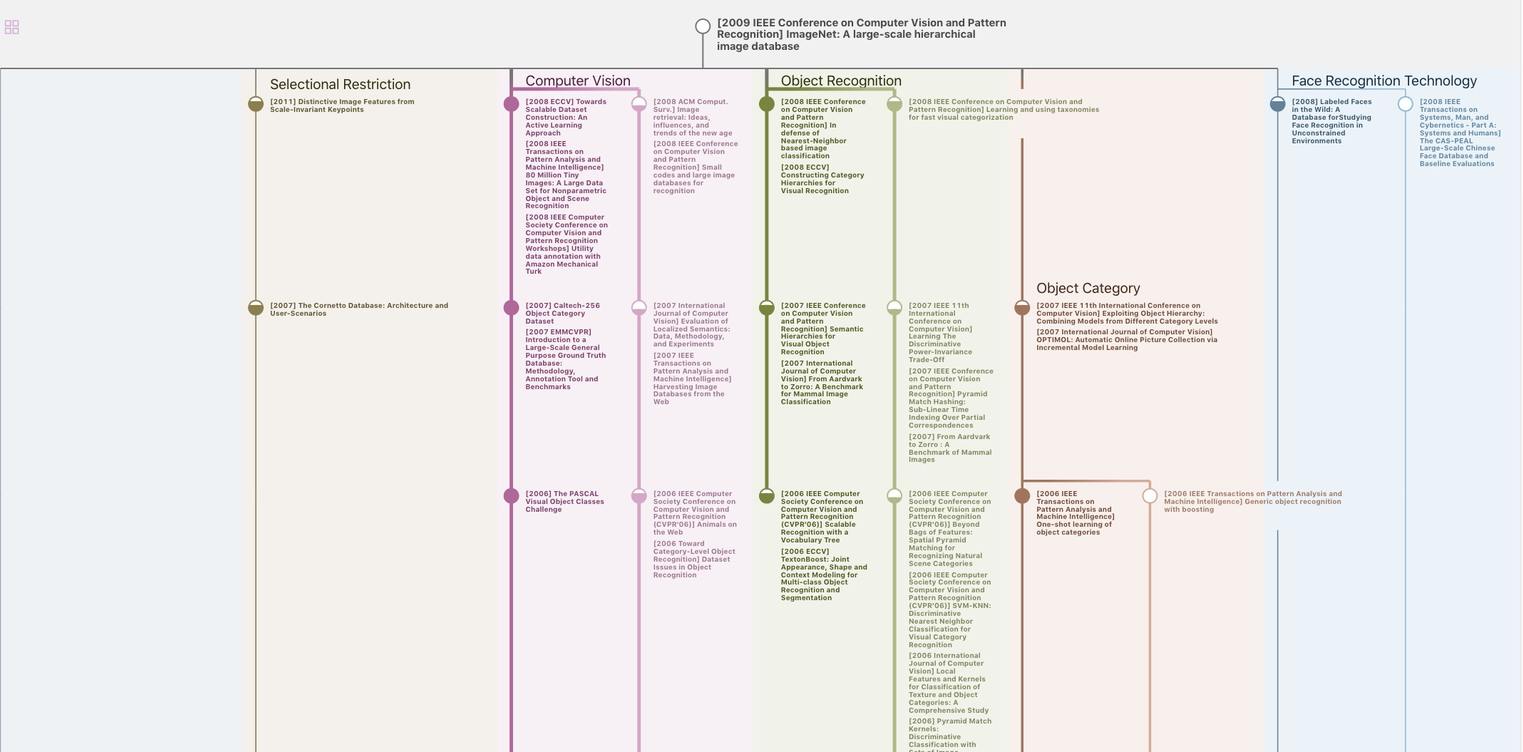
生成溯源树,研究论文发展脉络
Chat Paper
正在生成论文摘要