Optimization of artificial neural networks models applied to the identification of images of asteroids’ resonant arguments
arxiv(2022)
摘要
The asteroidal main belt is crossed by a web of mean motion and secular resonances that occur when there is a commensurability between fundamental frequencies of the asteroids and planets. Traditionally, these objects were identified by visual inspection of the time evolution of their resonant argument, which is a combination of orbital elements of the asteroid and the perturbing planet(s). Since the population of asteroids affected by these resonances is, in some cases, of the order of several thousand, this has become a taxing task for a human observer. Recent works used convolutional neural network ( CNN ) models to perform such task automatically. In this work, we compare the outcome of such models with those of some of the most advanced and publicly available CNN architectures, like the VGG, Inception , and ResNet . The performance of such models is first tested and optimized for overfitting issues, using validation sets and a series of regularization techniques like data augmentation, dropout, and batch normalization. The three best-performing models were then used to predict the labels of larger testing databases containing thousands of images. The VGG model, with and without regularizations, proved to be the most efficient method to predict labels of large datasets. Since the Vera C. Rubin observatory is likely to discover up to four million new asteroids in the next few years, the use of these models might become quite valuable to identify populations of resonant minor bodies.
更多查看译文
关键词
Time domain astronomy,Time series analysis,Minor planets,Asteroids,General
AI 理解论文
溯源树
样例
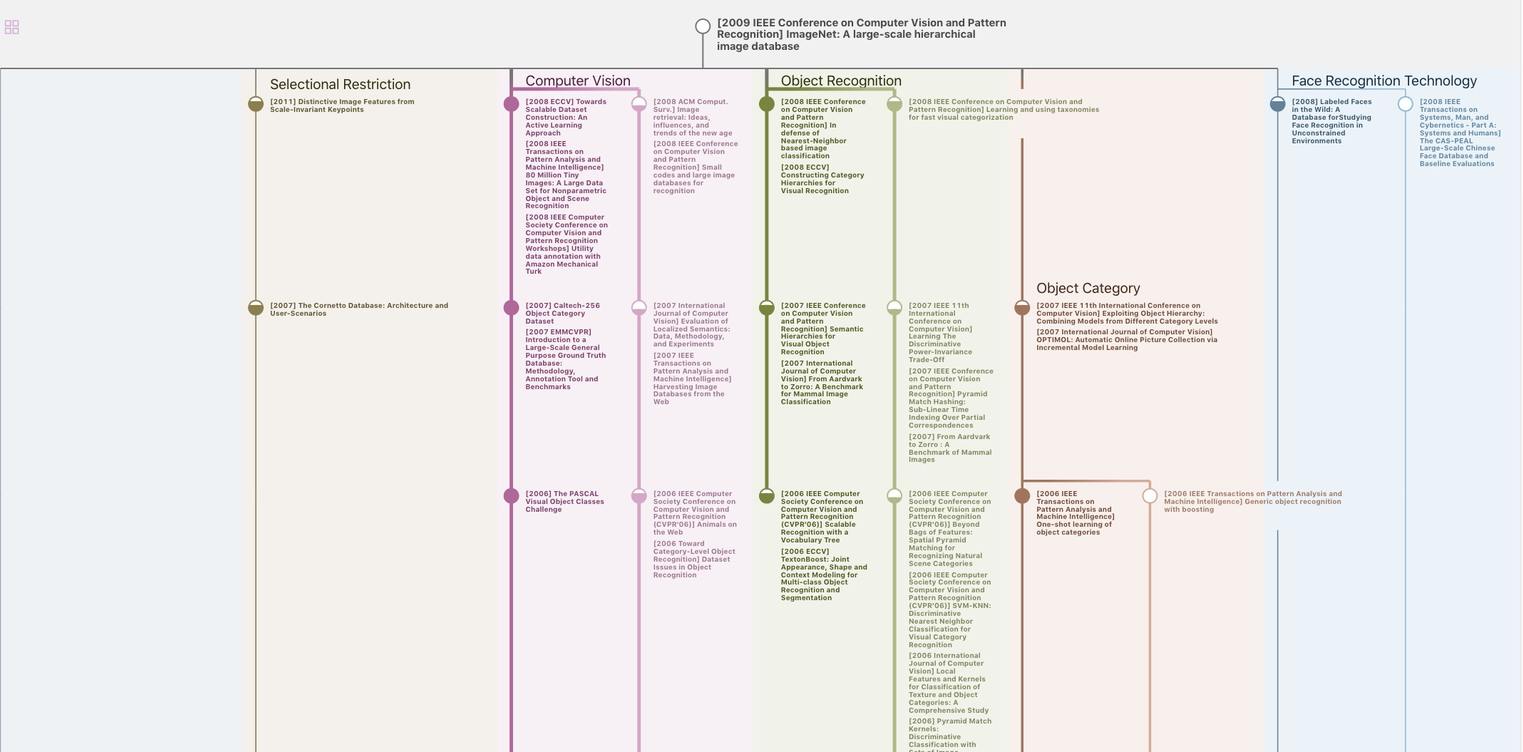
生成溯源树,研究论文发展脉络
Chat Paper
正在生成论文摘要