A Semi-Supervised Progressive Learning Algorithm for Brain-Computer Interface
IEEE TRANSACTIONS ON NEURAL SYSTEMS AND REHABILITATION ENGINEERING(2022)
摘要
Brain-computer interface (BCI) usually suffers from the problem of low recognition accuracy and large calibration time, especially when identifying motor imagery tasks for subjects with indistinct features and classifying fine grained motion control tasks by electroencephalogram (EEG)-electromyogram (EMG) fusion analysis. To fill the research gap, this paper presents an end-to-end semi-supervised learning framework for EEG classification and EEG-EMG fusion analysis. Benefiting from the proposed metric learning based label estimation strategy, sampling criterion and progressive learning scheme, the proposed framework efficiently extracts distinctive feature embedding from the unlabeled EEG samples and achieves a 5.40% improvement on BCI Competition IV Dataset IIa with 80% unlabeled samples and an average 3.35% improvement on two public BCI datasets. By employing synchronous EMG features as pseudo labels for the unlabeled EEG samples, the proposed framework further extracts deep level features of the synergistic complementarity between the EEG signals and EMG features based on the deep encoders, which improves the performance of hybrid BCI (with a 5.53% improvement for the Upper Limb Motion Dataset and an average 4.34% improvement on two hybrid datasets). Moreover, the ablation experiments show that the proposed framework can substantially improve the performance of the deep encoders (with an average 5.53% improvement). The proposed framework not only largely improves the performance of deep networks in the BCI system, but also significantly reduces the calibration time for EEG-EMG fusion analysis, which shows great potential for building an efficient and high-performance hybrid BCI for the motor rehabilitation process.
更多查看译文
关键词
Synchronous motors, Electroencephalography, Brain modeling, Task analysis, Electromyography, Feature extraction, Training, EEG classification, EEG-EMG fusion, progressive learning, semi-supervised learning, brain computer interface
AI 理解论文
溯源树
样例
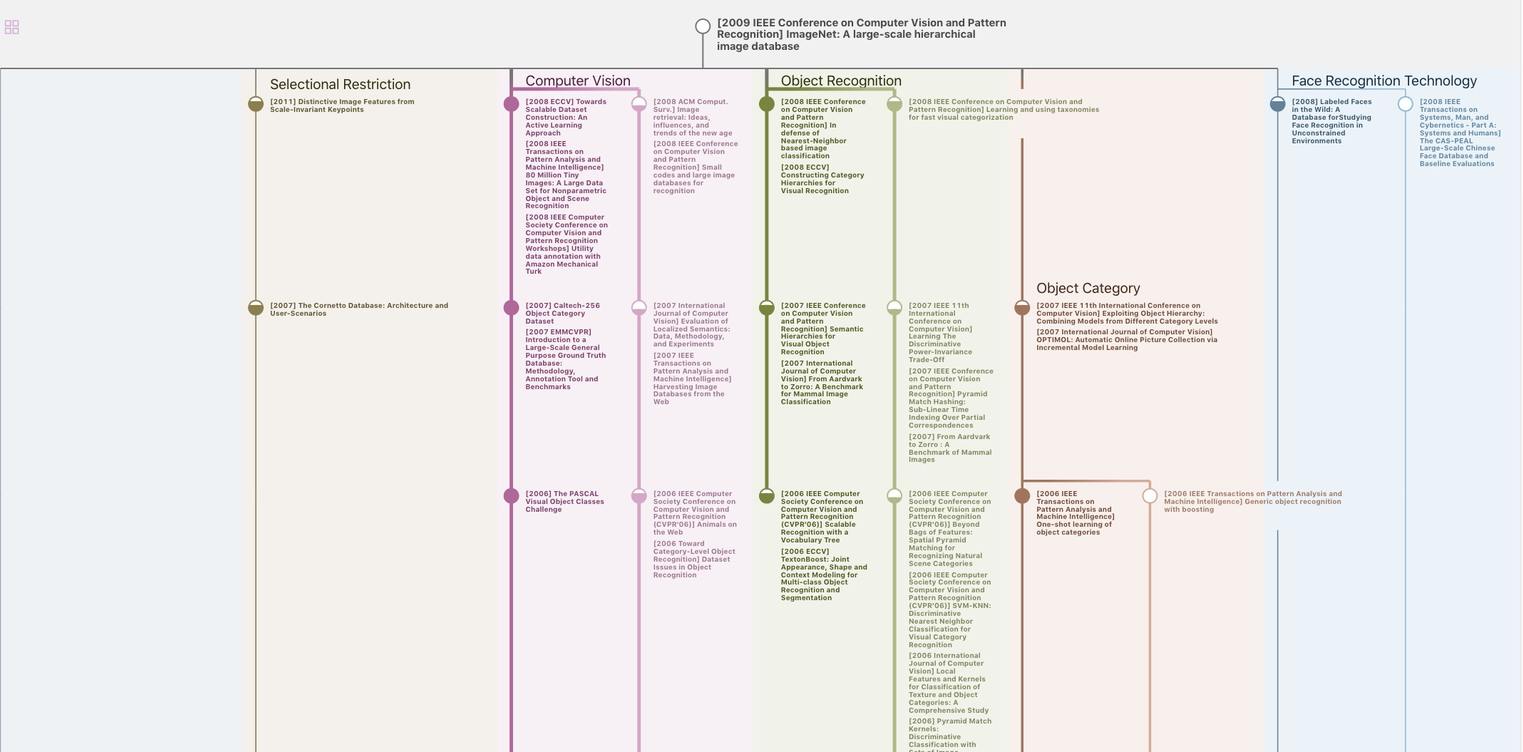
生成溯源树,研究论文发展脉络
Chat Paper
正在生成论文摘要