Semantic Segmentation of the Malignant Breast Imaging Reporting and Data System Lexicon on Breast Ultrasound Images by Using DeepLab v3+
SENSORS(2022)
摘要
In this study, an advanced semantic segmentation method and deep convolutional neural network was applied to identify the Breast Imaging Reporting and Data System (BI-RADS) lexicon for breast ultrasound images, thereby facilitating image interpretation and diagnosis by providing radiologists an objective second opinion. A total of 684 images (380 benign and 308 malignant tumours) from 343 patients (190 benign and 153 malignant breast tumour patients) were analysed in this study. Six malignancy-related standardised BI-RADS features were selected after analysis. The DeepLab v3+ architecture and four decode networks were used, and their semantic segmentation performance was evaluated and compared. Subsequently, DeepLab v3+ with the ResNet-50 decoder showed the best performance in semantic segmentation, with a mean accuracy and mean intersection over union (IU) of 44.04% and 34.92%, respectively. The weighted IU was 84.36%. For the diagnostic performance, the area under the curve was 83.32%. This study aimed to automate identification of the malignant BI-RADS lexicon on breast ultrasound images to facilitate diagnosis and improve its quality. The evaluation showed that DeepLab v3+ with the ResNet-50 decoder was suitable for solving this problem, offering a better balance of performance and computational resource usage than a fully connected network and other decoders.
更多查看译文
关键词
breast cancer, deep convolutional neural network, semantic segmentation, ultrasonic imaging, computer-aided diagnosis
AI 理解论文
溯源树
样例
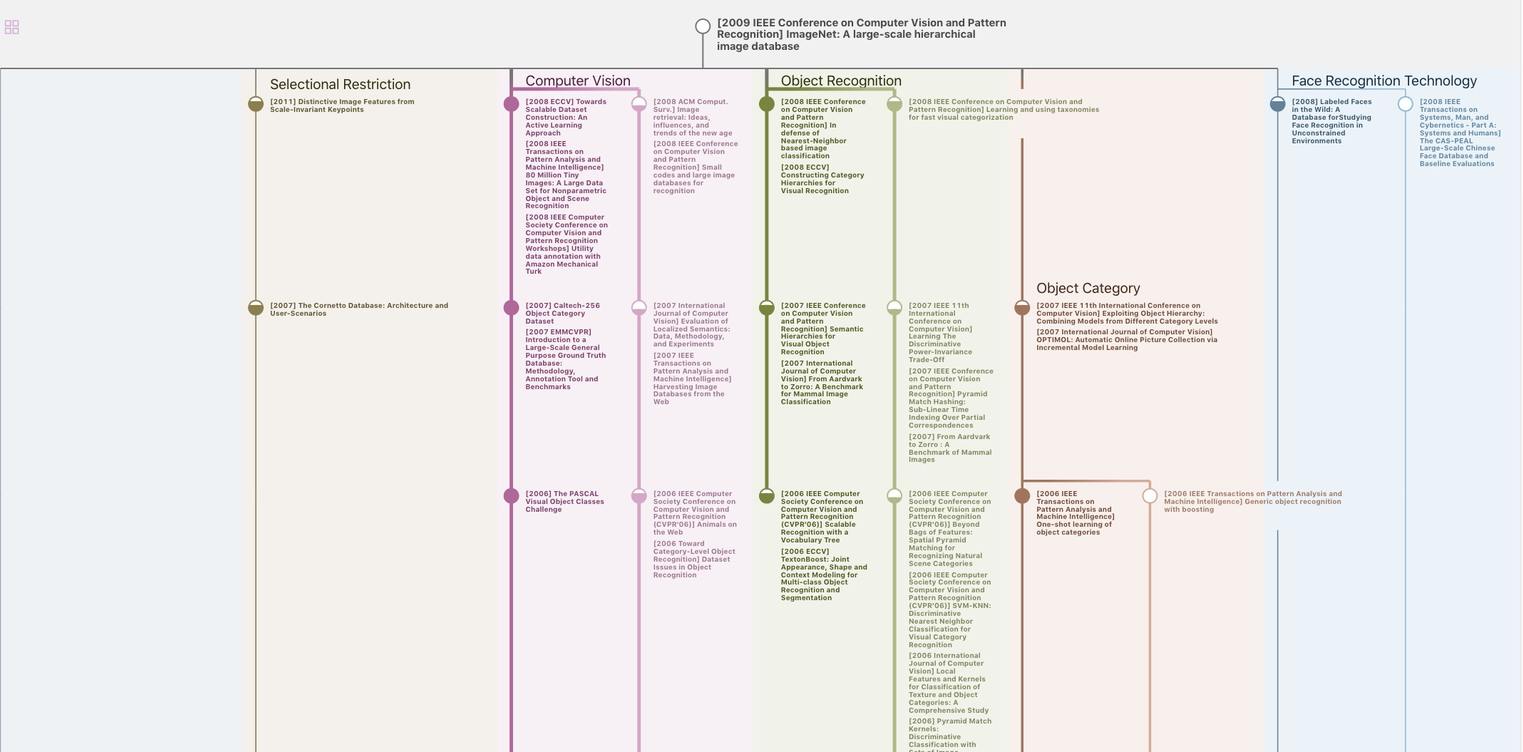
生成溯源树,研究论文发展脉络
Chat Paper
正在生成论文摘要