Comparison of Convolutional Neural Network Architectures for Robustness Against Common Artefacts in Dermatoscopic Images
DERMATOLOGY PRACTICAL & CONCEPTUAL(2022)
摘要
Introduction: Classification of dermatoscopic images via neural networks shows comparable performance to clinicians in experimental conditions but can be affected by artefacts like skin markings or rulers. It is unknown whether specialized neural networks are more robust to artefacts. Objectives: Analyze robustness of 3 neural network architectures, namely ResNet-34, Faster R-CNN and Mask R-CNN. Methods: We identified common artefacts in the HAM10000, PH2 and the 7-point criteria evaluation datasets, and established a template-based method to superimpose artefacts on dermatoscopic images. The HAM10000-dataset with and without superimposed artefacts was used to train the networks, followed by analyzing their robustness against artefacts in test images. Performance was assessed via area under the precision recall curve and classification results. Results: ResNet-34 and Faster R-CNN models trained on regular images perform worse than Mask R-CNN on images with superimposed artefacts. Artefacts added to all tested images led to a decrease in area under the precision-recall curve values of 0.030 for ResNet-34 and 0.045 for Faster R-CNN in comparison to only 0.011 for Mask R-CNN. However, changes in model performance only became significant with 40% or more of the images having superimposed artefacts. A loss in performance occurred when the training was biased by selectively superimposing artefacts on images belonging to a certain class. Conclusions: As Mask R-CNN showed the least decrease in performance when confronted with artefacts, instance segmentation architectures may be helpful to counter the effects of artefacts, warranting further research on related architectures. Our artefact insertion mechanism could be useful for future research.
更多查看译文
关键词
image classification,object detection,instance segmentation,artefacts,dermatoscopy
AI 理解论文
溯源树
样例
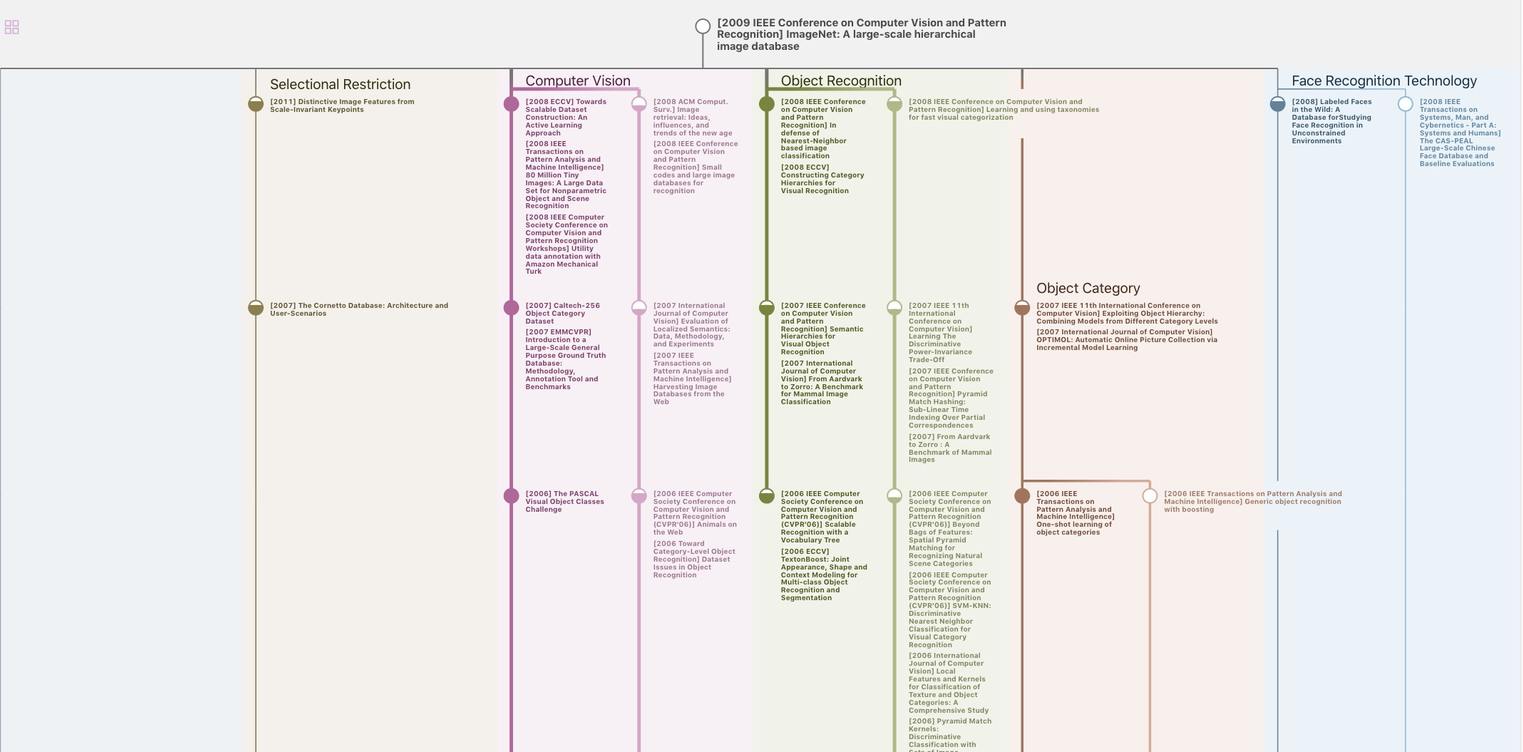
生成溯源树,研究论文发展脉络
Chat Paper
正在生成论文摘要