Comparing methods for assessing a difference in correlations with dependent groups, measurement error, nonnormality, and incomplete data.
Psychological methods(2022)
摘要
I compared multiple methods to estimate and test a difference in correlations (ρ) between two variables that are repeatedly measured or originate from dyads. Fisher's z transformed correlations are often used for testing ρ. However, raw scores are typically used directly to compute correlations under this popular method, whose performance has not been evaluated with measurement error or nonnormality in data. Structural equation modeling (SEM) can handle measurement error via latent-variable modeling. To handle nonnormality for testing a difference in correlations in dependent groups, when measurement error was of no concern, I discussed SEM using maximum likelihood estimation (ML) with sandwich-type standard errors, ML with bootstrap percentile or bias-corrected confidence intervals, and Bayesian credible intervals. In addition, I also examined a mean- and variance-adjusted likelihood ratio test and the Bollen-Stine bootstrapping model fit test. When measurement error existed, ρ was tested under a latent variable model. Furthermore, under the same SEM frameworks with complete data, I also assessed ρ with ignorable missing data. A simulation study was conducted considering whether measurement error existed, whether data were normal, and whether data were complete. Empirical examples were used for illustration. Recommendations were then given based on the findings. (PsycInfo Database Record (c) 2022 APA, all rights reserved).
更多查看译文
关键词
difference in correlations,dependent groups,structural equation modeling
AI 理解论文
溯源树
样例
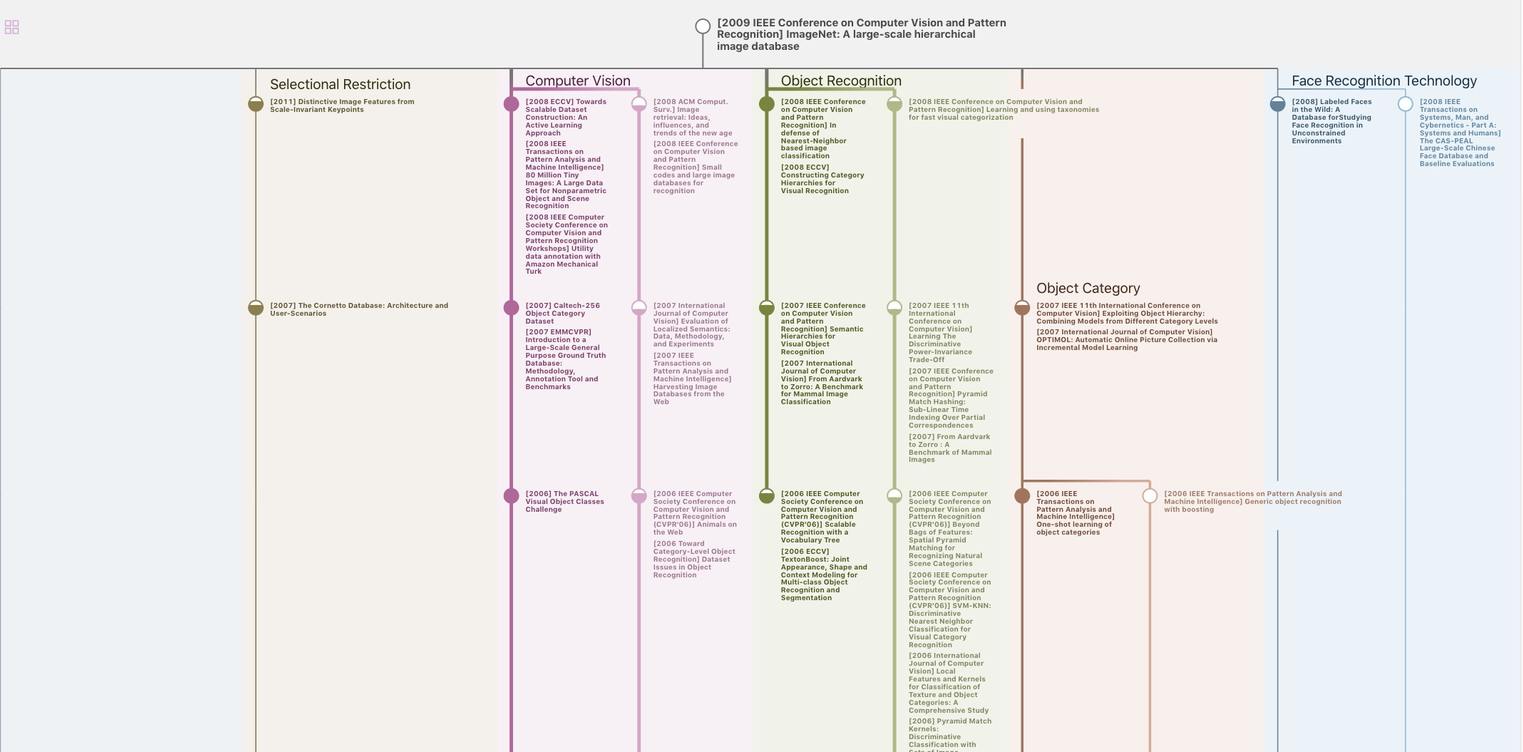
生成溯源树,研究论文发展脉络
Chat Paper
正在生成论文摘要