Inductive-transductive learning for very sparse fashion graphs
Neurocomputing(2022)
摘要
The assortments of global retailers are composed of hundreds of thousands of products linked by several types of relationships such as style compatibility, “bought together”, “watched together”, etc. Graphs are a natural representation for assortments, where products are nodes and relations are edges. Style compatibility relations are produced manually and do not cover the whole graph uniformly. We propose to use inductive learning to enhance a graph encoding style compatibility of a fashion assortment, leveraging rich node information comprising textual descriptions and visual data. Then, we show how the proposed graph enhancement substantially improves the performance on transductive tasks with a minor impact on graph sparsity. Although demonstrated in a challenging and novel industrial application case, the approach we propose is general enough to be applied to any node-level or edge-level prediction task in very sparse, large-scale networks.
更多查看译文
关键词
Deep graph networks,Deep learning for graphs,Recommendation systems,Learning with structured data,Fashion data
AI 理解论文
溯源树
样例
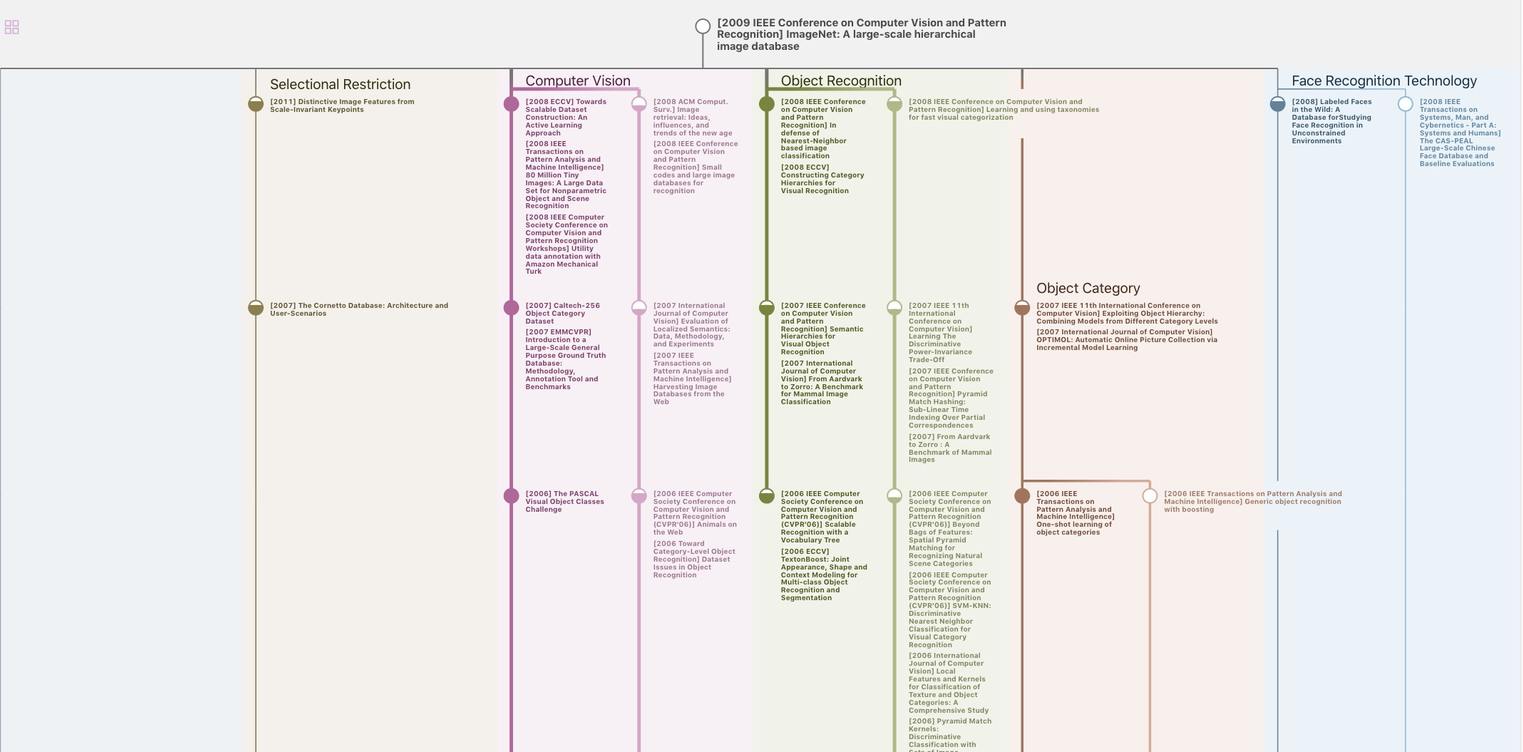
生成溯源树,研究论文发展脉络
Chat Paper
正在生成论文摘要