A dual learning-based recommendation approach
Knowledge-Based Systems(2022)
摘要
Data sparsity and cold start are two critical issues which need to be addressed in recommender systems (RSs). Currently, most methods address these issues by applying user history files or some side information to improve the user model and complete the rating matrix. However, such methods cannot perform well when labeled data is scarce or unavailable. In this paper, we propose a dual learning-based recommendation approach (DLRA). DLRA can trigger initial recommendation and improve the quality of recommendations by using the duality characteristics of RSs, even when the available labeled information is scarce. Specifically, DLRA regards the recommendation task as two independent subtasks — primal task and dual task, and these two tasks show strong duality in DLRA. The primal task is item-centered which aims to find users who can rate high for items, while the dual task is user-centered that aims to recommend the most favorite items to users. These two tasks have strong dualities in terms of the recommendation space, selection probability and recommendation basis. Based on these dualities, we design three dual learning strategies to couple the whole recommendation process and realize the self-tuning and self-improvement of each task model, and finally optimize the whole recommendation model. Based on the dataset of Movielens and BookCrossing, we simulate data sparsity and cold start recommendation scenarios, the experimental results show that DLRA achieves substantial improvement when the labeled data is scare, and it outperforms other hybrid recommendation approaches and deep learning strategies with a smaller predictive error as well as better recommendation accuracy.
更多查看译文
关键词
Recommender system,Dual learning,Data sparsity,Duality,Hybrid filtering recommendation
AI 理解论文
溯源树
样例
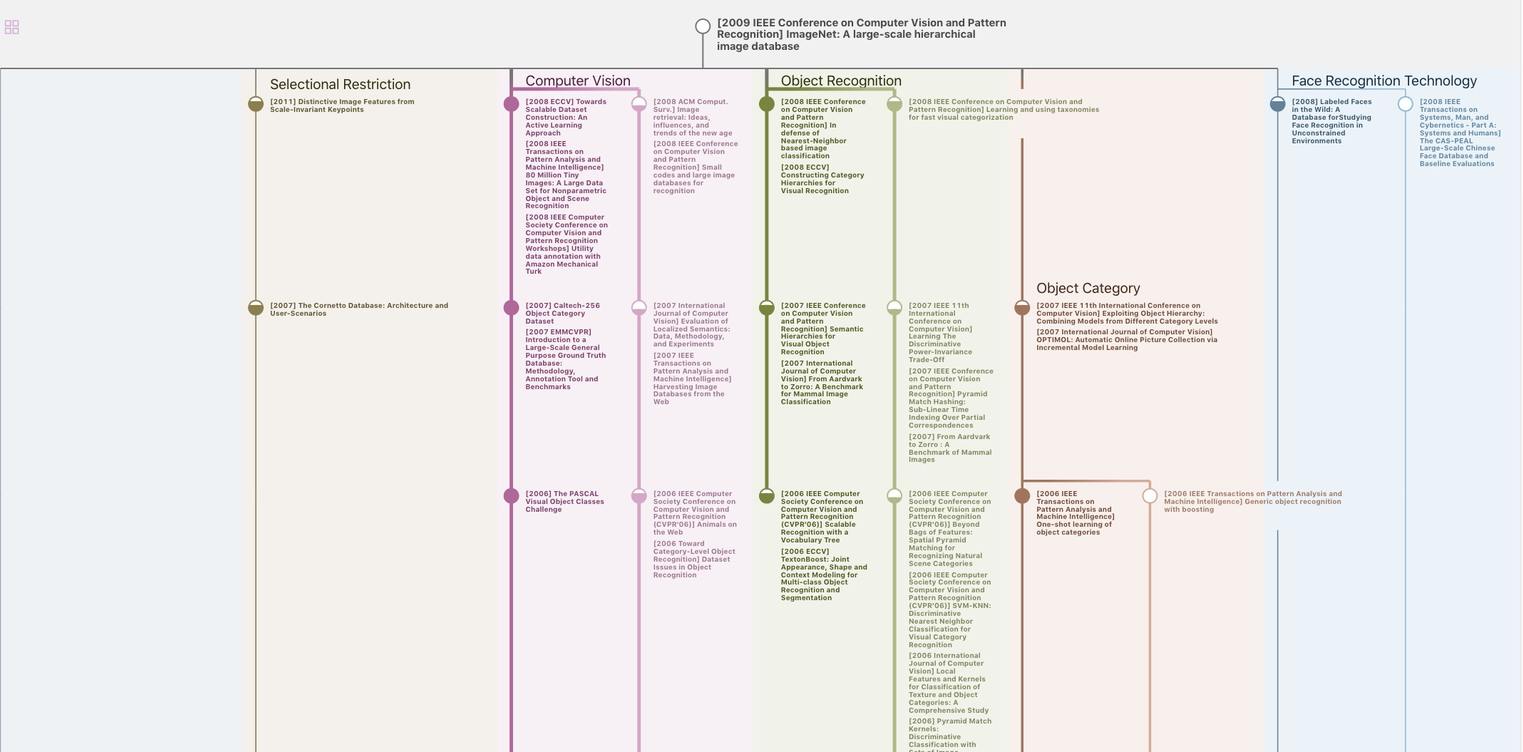
生成溯源树,研究论文发展脉络
Chat Paper
正在生成论文摘要