The least-squares reverse time migration with gradient optimization based on QHAdam
Chinese Journal of Geophysics(2022)
摘要
High-resolution imaging result derived by Least-Squares Reverse Time Migration (LSRTM) is achieved through iterative gradient calculations and model updates usually. But in conventional LSRTM, the gradient without optimization will lead to low convergence efficiency and low imaging accuracy. Besides, the calculation of step-length in iteration introduces extra wave-field extrapolation. This paper introduces a deep learning optimization algorithm, called QHAdam, into conventional time-domain LSRTM, optimizing the gradients and calculating variations of models directly with less computational cost. Experiments based on Marmousi model show that the LSRTM based on QHAdam method has higher computational efficiency compared with conventional algorithm since avoiding the calculation of step-lengths for every iteration except for the first one, and it also has higher convergence efficiency and imaging accuracy than that based on conventional and Adam algorithms.
更多查看译文
关键词
Least-Squares Reverse Time Migration (LSRTM), Gradient optimization, QHAdam, Deep learning
AI 理解论文
溯源树
样例
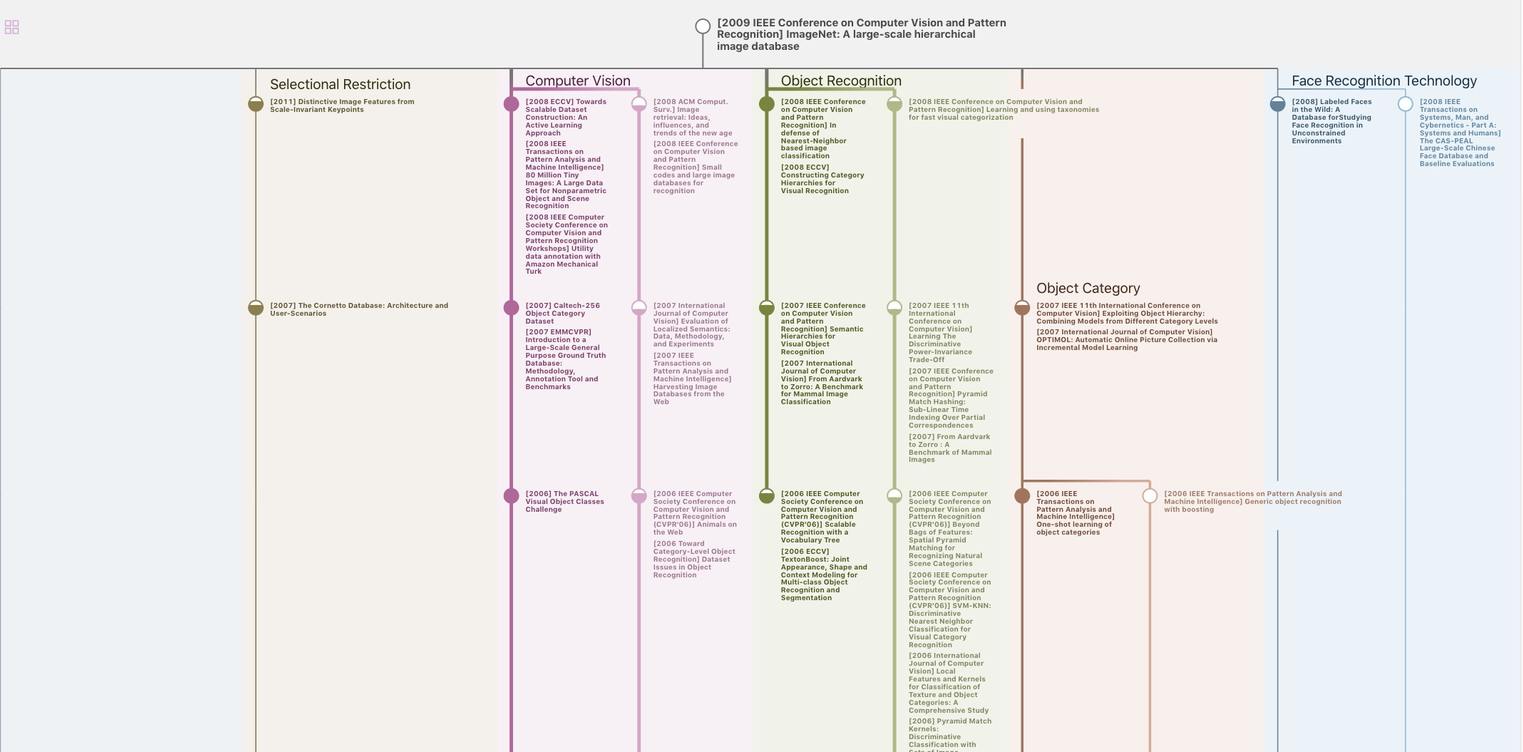
生成溯源树,研究论文发展脉络
Chat Paper
正在生成论文摘要