Bridging the Gap Between Deep Learning and Hypothesis-Driven Analysis via Permutation Testing
PREDICTIVE INTELLIGENCE IN MEDICINE (PRIME 2022)(2022)
摘要
A fundamental approach in neuroscience research is to test hypotheses based on neuropsychological and behavioral measures, i.e., whether certain factors (e.g., related to life events) are associated with an outcome (e.g., depression). In recent years, deep learning has become a potential alternative approach for conducting such analyses by predicting an outcome from a collection of factors and identifying the most "informative" ones driving the prediction. However, this approach has had limited impact as its findings are not linked to statistical significance of factors supporting hypotheses. In this article, we proposed a flexible and scalable approach based on the concept of permutation testing that integrates hypothesis testing into the data-driven deep learning analysis. We apply our approach to the yearly self-reported assessments of 621 adolescent participants of the National Consortium of Alcohol and Neurodevelopment in Adolescence (NCANDA) to predict negative valence, a symptom of major depressive disorder according to the NIMH Research Domain Criteria (RDoC). Our method successfully identifies categories of risk factors that further explain the symptom.
更多查看译文
关键词
Permutation testing, Risk factor identification, Classification, Behavioral data, Outcome prediction, Disease prediction
AI 理解论文
溯源树
样例
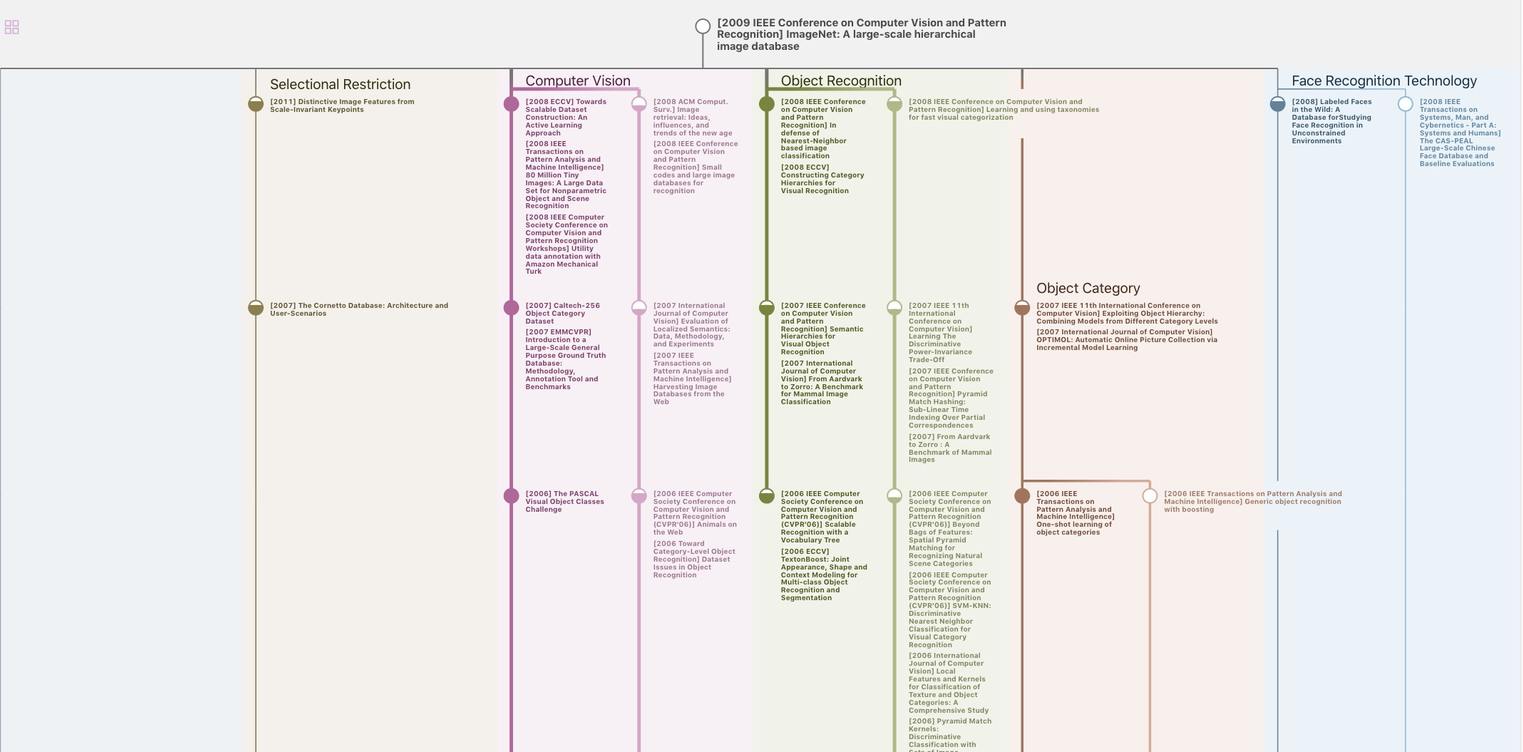
生成溯源树,研究论文发展脉络
Chat Paper
正在生成论文摘要