Markov Chain-based Policies for Multi-stage Stochastic Integer Linear Programming with an Application to Disaster Relief Logistics
arxiv(2022)
摘要
We introduce a novel aggregation framework to address multi-stage stochastic programs with mixed-integer state variables and continuous local variables (MSILPs). Our aggregation framework imposes additional structure to the integer state variables by leveraging the information of the underlying stochastic process, which is modeled as a Markov chain (MC). We present a novel branch-and-cut algorithm integrated with stochastic dual dynamic programming as an exact solution method to the aggregated MSILP, which can also be used in an approximation form to obtain dual bounds and implementable feasible solutions. Moreover, we apply two-stage linear decision rule (2SLDR) approximations and propose MC-based variants to obtain high-quality decision policies with significantly reduced computational effort. We test the proposed methodologies in a novel MSILP model for hurricane disaster relief logistics planning. Our empirical evaluation compares the effectiveness of the various proposed approaches and analyzes the trade-offs between policy flexibility, solution quality, and computational effort. Specifically, the 2SLDR approximation yields provable near-optimal solutions for our test instances supported by the proposed bounding procedure.
更多查看译文
关键词
markov,chain-based,multi-stage
AI 理解论文
溯源树
样例
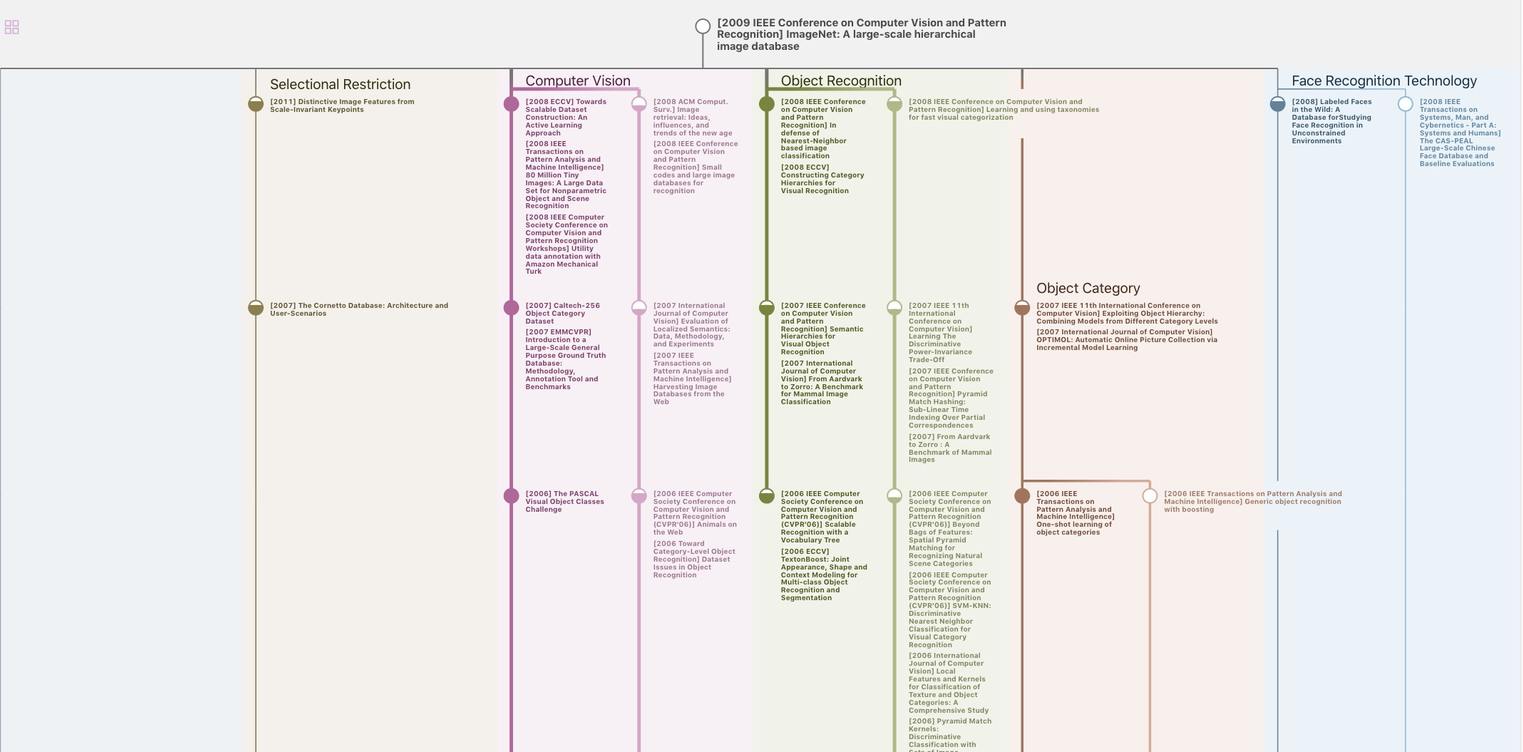
生成溯源树,研究论文发展脉络
Chat Paper
正在生成论文摘要