A Hybrid Complex-valued Neural Network Framework with Applications to Electroencephalogram (EEG)
arxiv(2022)
摘要
In this article, we present a new EEG signal classification framework by integrating the complex-valued and real-valued Convolutional Neural Network(CNN) with discrete Fourier transform (DFT). The proposed neural network architecture consists of one complex-valued convolutional layer, two real-valued convolutional layers, and three fully connected layers. Our method can efficiently utilize the phase information contained in the DFT. We validate our approach using two simulated EEG signals and a benchmark data set and compare it with two widely used frameworks. Our method drastically reduces the number of parameters used and improves accuracy when compared with the existing methods in classifying benchmark data sets, and significantly improves performance in classifying simulated EEG signals.
更多查看译文
关键词
Medical signals, Electroencephalogram (EEG), Machine learning, Neural networks
AI 理解论文
溯源树
样例
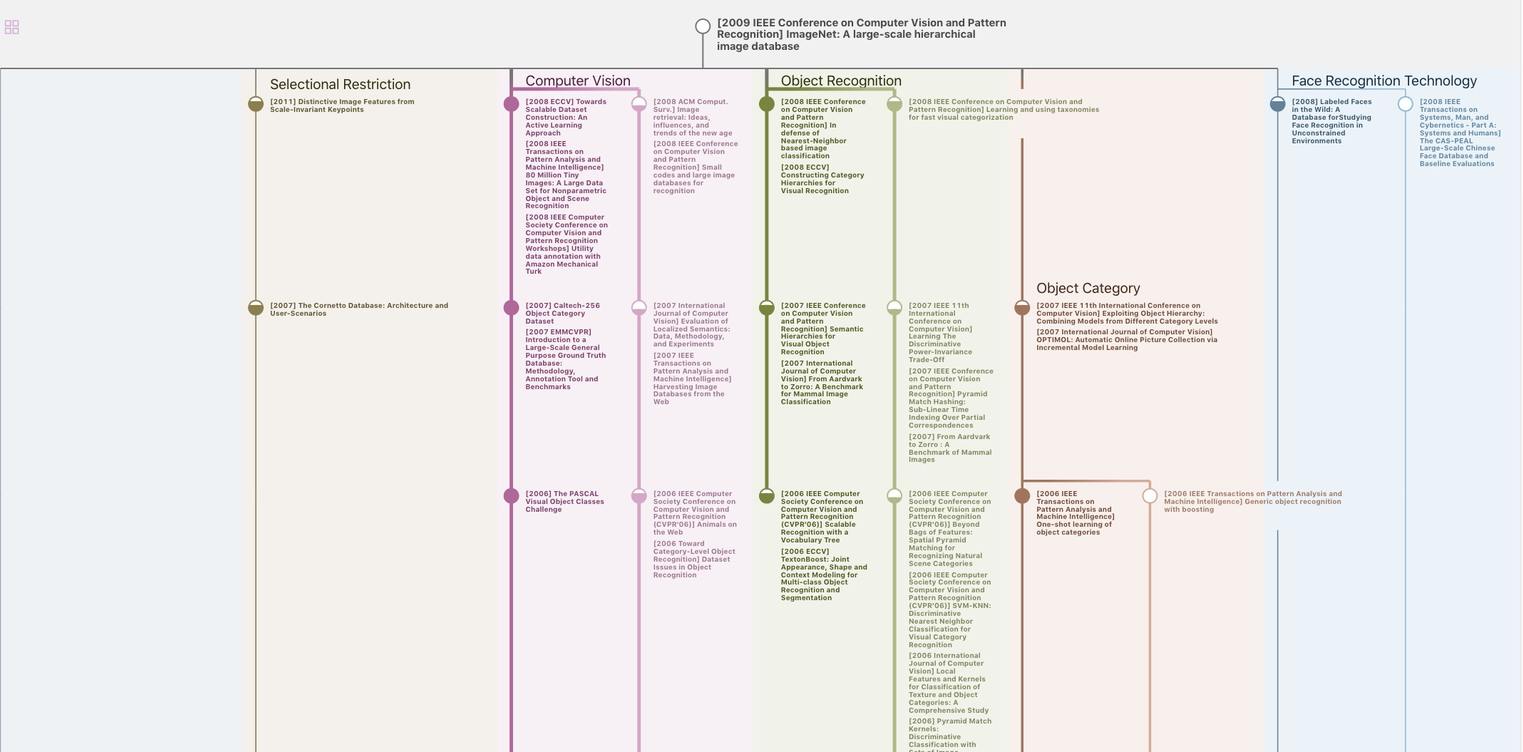
生成溯源树,研究论文发展脉络
Chat Paper
正在生成论文摘要