Integrating autoencoder and heteroscedastic noise neural networks for industrial data analysis and soft-sensor design
Computer Aided Chemical Engineering 32nd European Symposium on Computer Aided Process Engineering(2022)
摘要
Viscosity represents a key indicator of product quality but has traditionally been difficult to measure in-process in real-time. This is particularly true if the process involves complex mixing phenomena operated at dynamic conditions. To address this challenge, a promising solution to monitoring product viscosity is to design soft-sensors which correlate viscosity with easily measured process variables. In this study, we developed an innovative machine learning based soft-sensor construction framework by integrating different types of advanced artificial neural networks. The framework first employs a deep learning autoencoder to generate information-rich statistic latent variables by compressing high-dimensional industrial data, and then adopts a heteroscedastic noise neural network to simultaneously predict product viscosity and process variance based on the extracted latent features. To evaluate its accuracy and robustness, the data-driven soft-sensor was used to predict product viscosity for a number of industrial batches operated over different seasons. It is found that the soft-sensor has both high accuracy (prediction error < 12%) and high robustness in most of the cases, indicating its great potential for industrial batch process monitoring and quality control.
更多查看译文
关键词
heteroscedastic noise,industrial data analysis,autoencoder,neural networks,soft-sensor
AI 理解论文
溯源树
样例
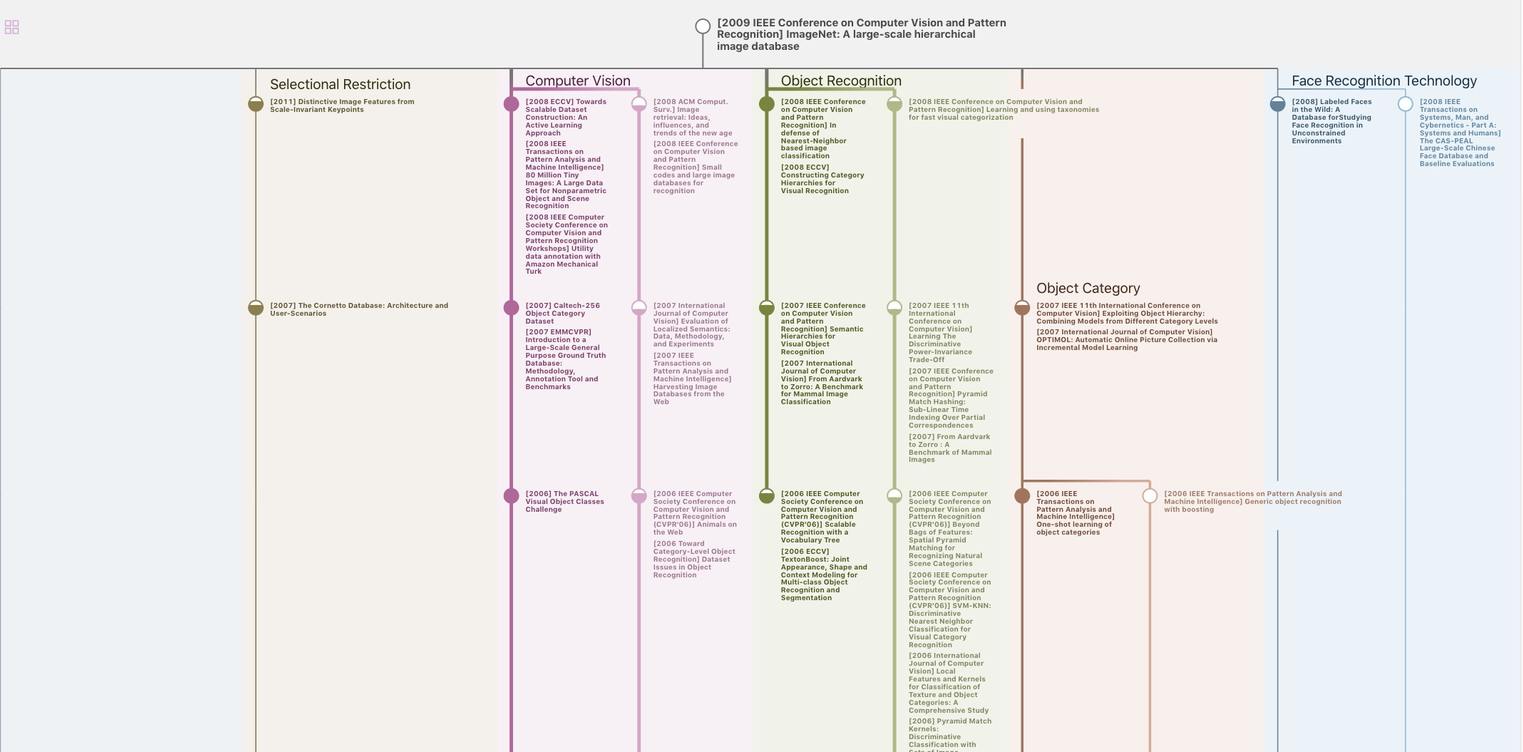
生成溯源树,研究论文发展脉络
Chat Paper
正在生成论文摘要