Health-aware control using hybrid models applied to a gas-lifted oil well network
Computer Aided Chemical Engineering 32nd European Symposium on Computer Aided Process Engineering(2022)
摘要
A health-aware controller (HAC) aims at finding the optimal trade-off between system reliability and productivity. By considering the system health, the controller can avoid unexpected equipment breakdown while improving the system economic performance. In subsea oil extraction industry, new and more stringent environmental, safety, and regulatory requirements are imposed every year. Thus, this industry can benefit from the implementation of this type of controllers for tasks such as equipment monitoring, or flow assurance. One challenge when implementing a health-aware controller is to derive models that represent the failure mechanism of subsea equipment using physics-based relationships. An alternative is to use data-based models. Instead of relying on physical knowledge, this type of models determine the input-output relationships using only data observed experimentally. In this paper, we propose the use of a hybrid HAC, where first-principles relations describe the mass and energy balances, while the system degradation evolution is represented by a data-driven model. We test two different structures, a linear regression and a neural network model. We investigate their performance in open-loop, carrying out an uncertainty analysis on the predicted degradation variability, as well as in closed-loop. The simulation results show that HAC's performance is dependent on the type of data-driven model used for predicting degradation, and the more complex model does not necessarily give a better overall result.
更多查看译文
关键词
hybrid models,health-aware,gas-lifted
AI 理解论文
溯源树
样例
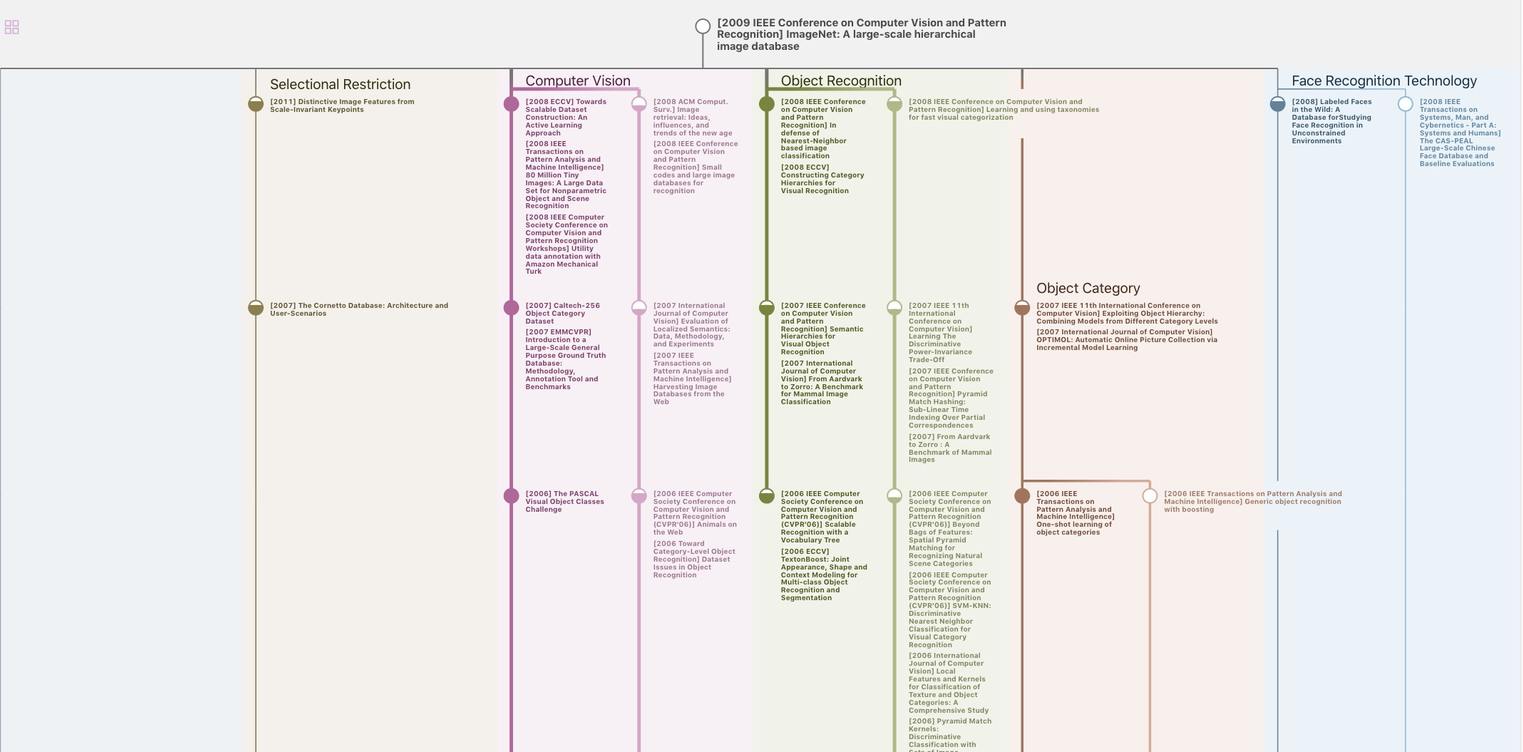
生成溯源树,研究论文发展脉络
Chat Paper
正在生成论文摘要