Ensemble-based unsupervised machine learning method for membership determination of open clusters using Mahalanobis distance
MONTHLY NOTICES OF THE ROYAL ASTRONOMICAL SOCIETY(2022)
摘要
We present an improved method for the determination of membership of an open cluster using ensemble-based unsupervised machine learning techniques. The working principle of this method relies on two stages: (i) choosing a suitable range of three astrometric parameters (pi, mu(alpha)cos delta, mu(delta)) using k-nearest neighbour (kNN) algorithm on the data downloaded for the cluster within a smaller search radius; (ii) application of two component Gaussian mixture modelling (GMM) on the resulting one dimensional Gaussian distribution of Mahalanobis distance (MD) of stars using the range of parameters obtained from the earlier step, but with the data downloaded within a bigger search radius. MD is calculated from the mean of each of the parameters in three dimensions. Thus the use of MD reduces the input of the GMM from the 3D parameter space into a 1D parameter space for the cluster membership determination. The method has been tested on a few clusters including those which have overlaps in some/all the parameters using the data obtained from the Gaia DR3 data base. It is found that the approach can easily separate the cluster members from the field stars. The clean colour-magnitude diagrams and similar direction of proper motions of the member stars obtained for the clusters shows that this method is very efficient and robust in segregating the cluster members from the field stars.
更多查看译文
关键词
methods: data analysis,open clusters and associations: general,methods: statistical-astrometry-parallaxes-proper motions
AI 理解论文
溯源树
样例
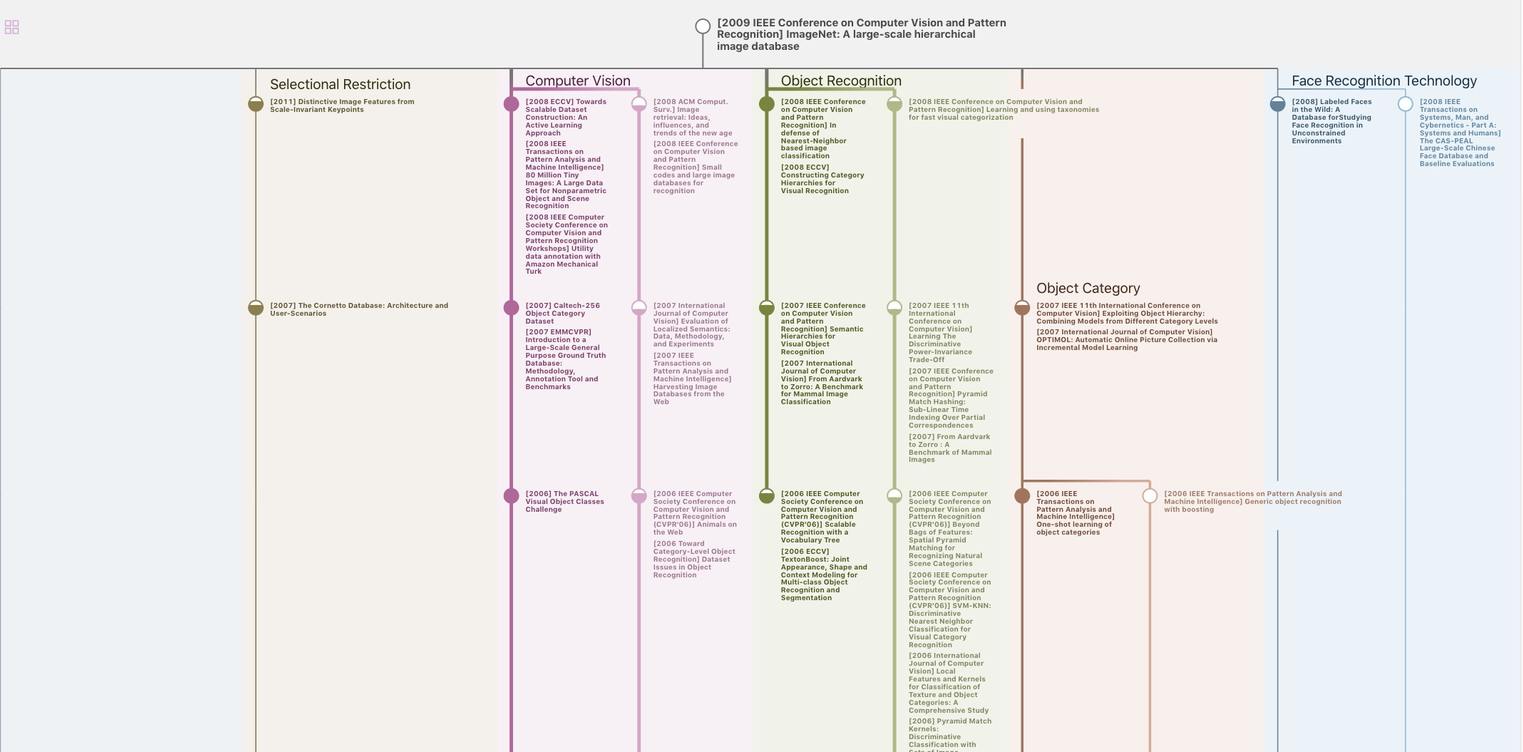
生成溯源树,研究论文发展脉络
Chat Paper
正在生成论文摘要