VREFL: Verifiable and Reconnection-Efficient Federated Learning in IoT scenarios
Journal of Network and Computer Applications(2022)
摘要
The privacy of social IoT data needs to be ensured while it is used by Federated Learning (FL). Secure aggregation is a cryptographic primitive that can ensure the privacy of social IoT data. However, in IoT scenarios, the secrecy of secure aggregation inputs makes the aggregated results less credible and the frequent disconnection and reconnection of IoT workers will cause prohibited communication overhead. In this work, we propose the Verifiable and Reconnection-Efficient Federated Learning (VREFL) framework, which can simultaneously support secure aggregation of local updates, verification of aggregated results, and rapid reconnection of dropped workers. Specifically, the novelty can be twofold: (1) we let each worker generate its homomorphic hash proof for the verification while updating locally. These hash proofs will be bound together through the Merkle Tree (MT) for further investigation by the central server; (2) we use the Chinese Remainder Theory (CRT) to help each worker efficiently update its “obsolete mask” after reconnection. We also theoretically prove that VREFL is secure. In addition, we implement a prototype of VREFL and conduct experiments on real-world datasets with different settings. Extensive experimental results prove that our protocol is feasible and efficient.
更多查看译文
关键词
Federated learning,Secure aggregation,Verifiable computation,Homomorphic hash,Chinese Remainder Theory
AI 理解论文
溯源树
样例
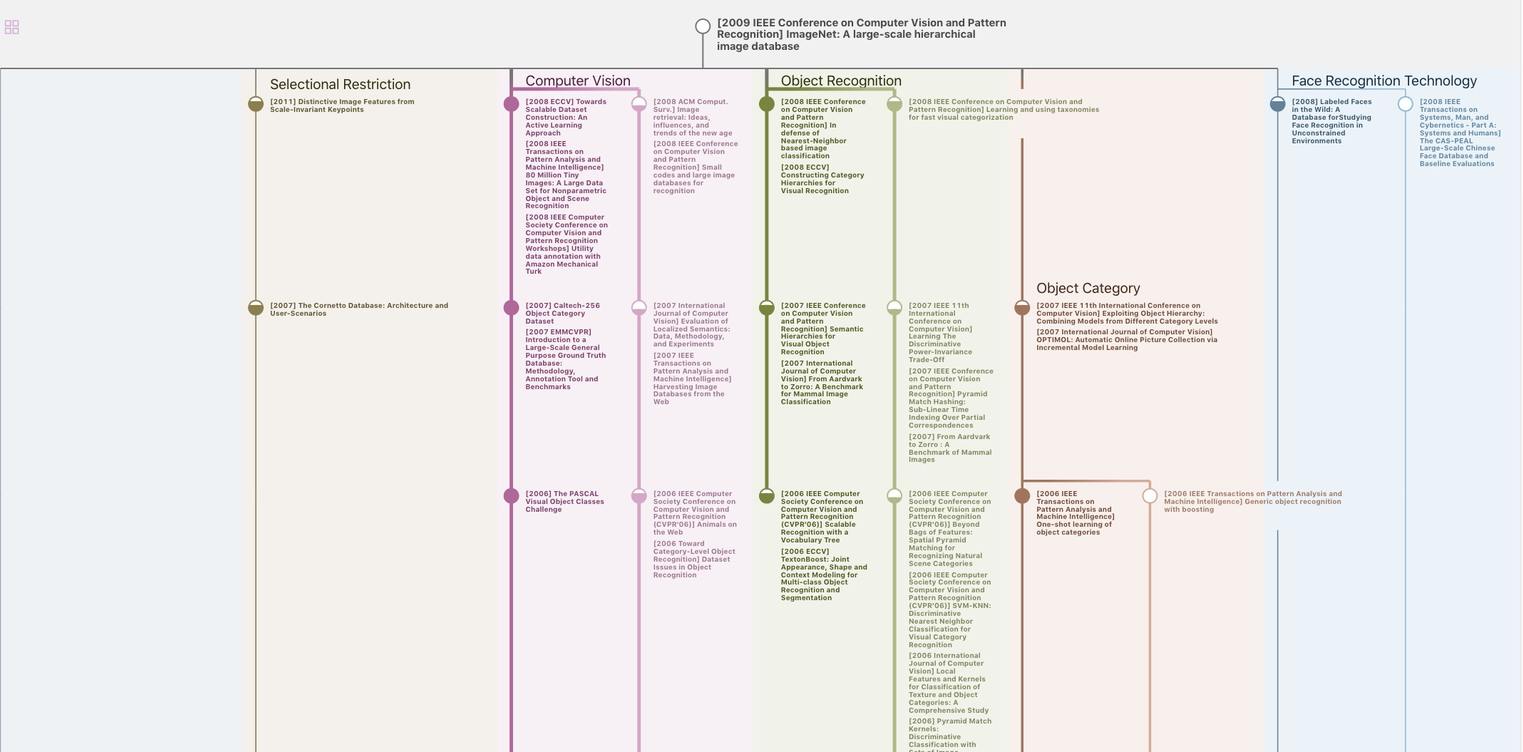
生成溯源树,研究论文发展脉络
Chat Paper
正在生成论文摘要