Enhanced gradient-based MCMC in discrete spaces
arxiv(2022)
摘要
The recent introduction of gradient-based MCMC for discrete spaces holds great promise, and comes with the tantalising possibility of new discrete counterparts to celebrated continuous methods such as MALA and HMC. Towards this goal, we introduce several discrete Metropolis-Hastings samplers that are conceptually-inspired by MALA, and demonstrate their strong empirical performance across a range of challenging sampling problems in Bayesian inference and energy-based modelling. Methodologically, we identify why discrete analogues to preconditioned MALA are generally intractable, motivating us to introduce a new kind of preconditioning based on auxiliary variables and the `Gaussian integral trick'.
更多查看译文
关键词
mcmc,gradient-based
AI 理解论文
溯源树
样例
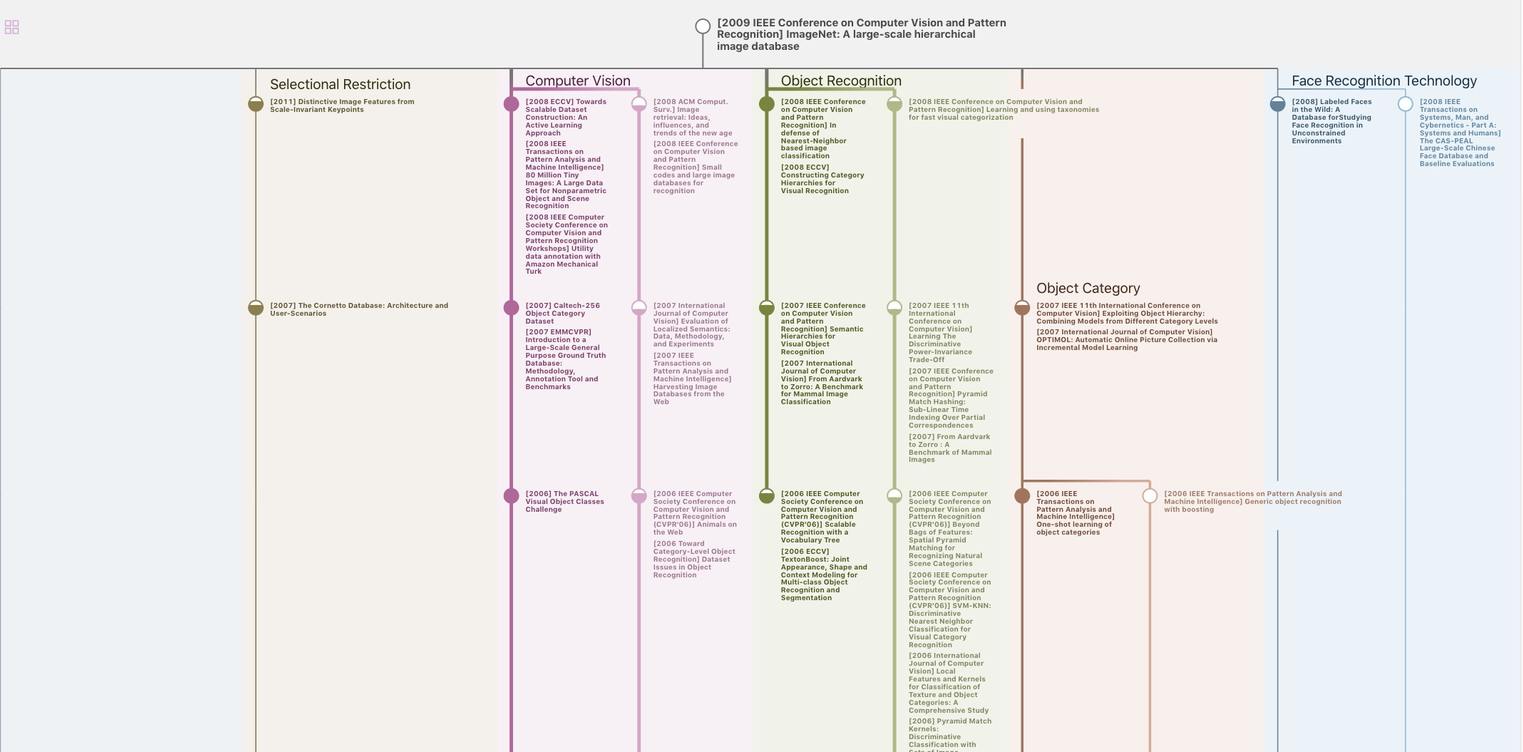
生成溯源树,研究论文发展脉络
Chat Paper
正在生成论文摘要