Approximating Hessian matrices using Bayesian inference: a new approach for quasi-Newton methods in stochastic optimization
OPTIMIZATION METHODS & SOFTWARE(2024)
摘要
Using quasi-Newton methods in stochastic optimization is not a trivial task given the difficulty of extracting curvature information from the noisy gradients. Moreover, pre-conditioning noisy gradient observations tend to amplify the noise. We propose a Bayesian approach to obtain a Hessian matrix approximation for stochastic optimization that minimizes the secant equations residue while retaining the extreme eigenvalues between a specified range. Thus, the proposed approach assists stochastic gradient descent to converge to local minima without augmenting gradient noise. We propose maximizing the log posterior using the Newton-CG method. Numerical results on a stochastic quadratic function and an $ \ell _2 $ & ell;2-regularized logistic regression problem are presented. In all the cases tested, our approach improves the convergence of stochastic gradient descent, compensating for the overhead of solving the log posterior maximization. In particular, pre-conditioning the stochastic gradient with the inverse of our Hessian approximation becomes more advantageous the larger the condition number of the problem is.
更多查看译文
关键词
Stochastic optimization,quasi-Newton,Monte Carlo,variance reduction,control variates,machine learning
AI 理解论文
溯源树
样例
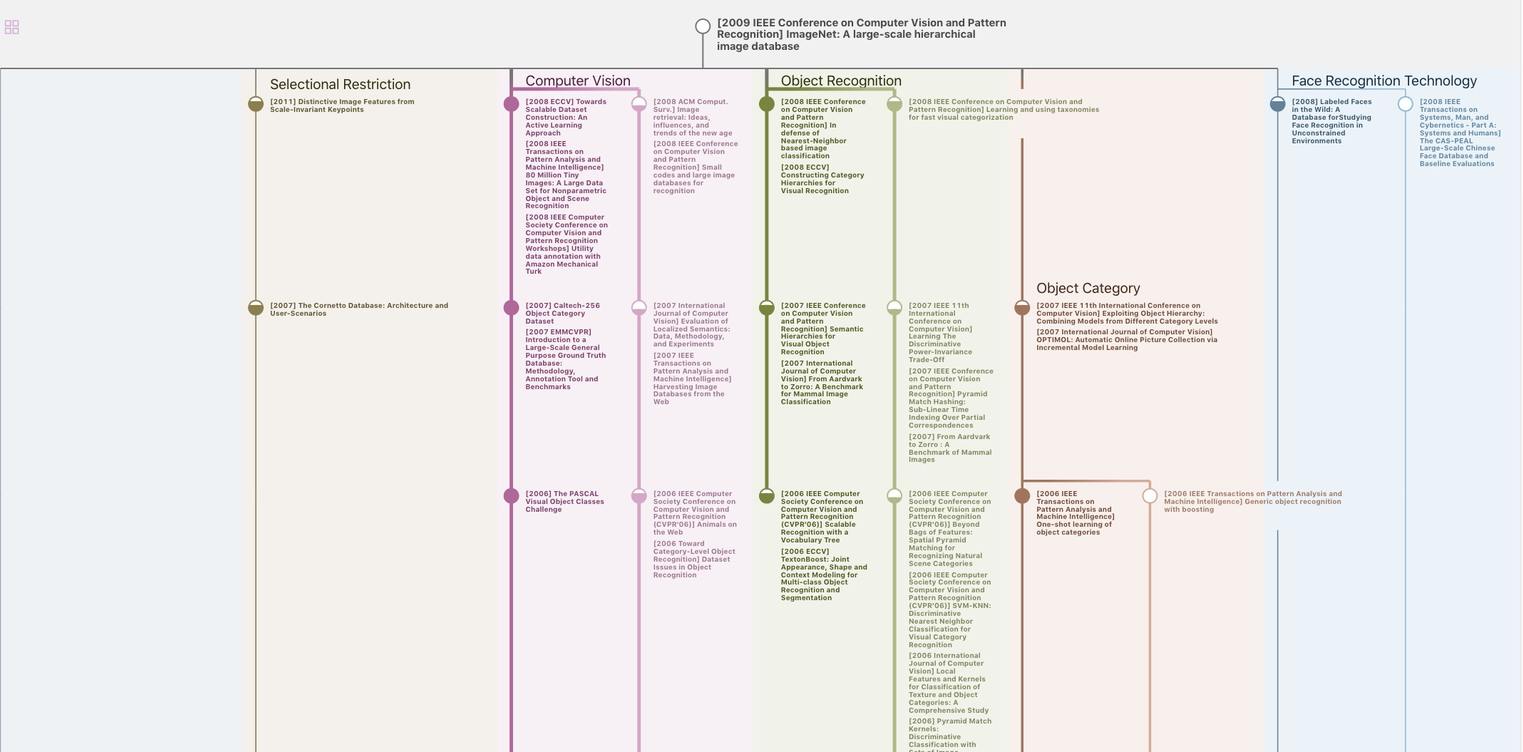
生成溯源树,研究论文发展脉络
Chat Paper
正在生成论文摘要