A Residual CNN Based Denoiser for Reliable Recovery of Bit Stream With Applications to Soft Channel Decoding
IEEE Transactions on Cognitive Communications and Networking(2022)
摘要
The noise existent in practical systems degrades reliability performances, especially when delivering data over fast fading channels with the correlated noise. To address this issue, we propose a residual convolutional neural network aided denoiser (ResCNND) to suppress the noise for more reliable information recovery. The intelligent ResCNND is composed by convolutional layers, batch normalization layers and nonlinear activation functions. The ResCNND aided denoiser is then integrated with different types of channel decoders, and we propose a data preprocessing module to embed the statistical characteristics of channel conditions in terms of channel state information (CSI) as inputs to the neural network. Additionally, we propose the loss function by combining the mean square error loss and the normality test loss to simultaneously reduce the noise power and preserve the Gaussian distribution of residual noises. Then the well trained ResCNND can be used to suppress the noise in real time. In simulations, three types of channel codes, including LDPC, Polar and Turbo codes, are considered. The results demonstrate that the proposed ResCNND-aided receiver can effectively improve the signal-to-noise ratio and bit error rate performances with no requirement of perfect CSI, thereby achieving better reliability and robustness performances than benchmark schemes.
更多查看译文
关键词
Noise reduction,Correlated noise,deep learning,fast fading channel,residual convolutional neural network aided denoiser,soft decoder
AI 理解论文
溯源树
样例
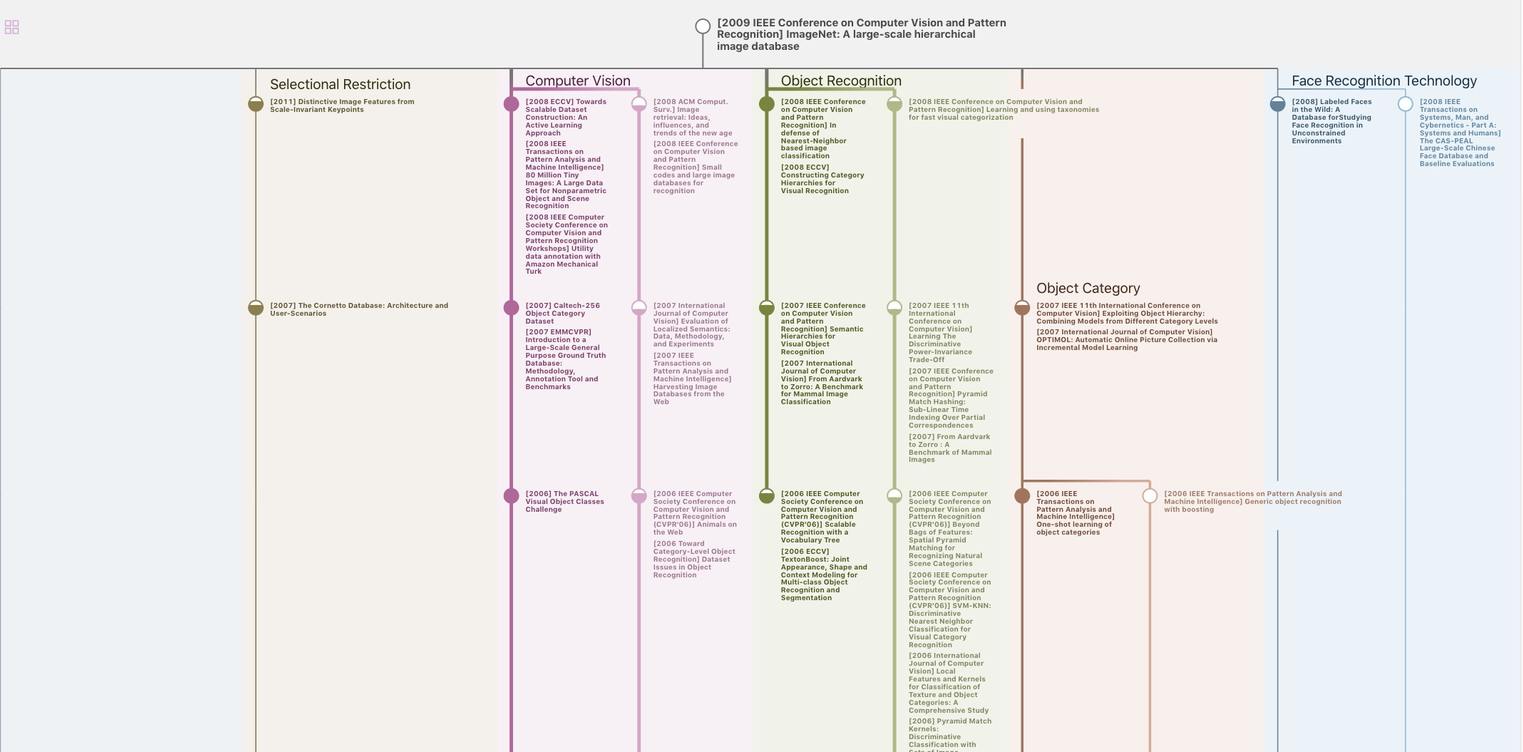
生成溯源树,研究论文发展脉络
Chat Paper
正在生成论文摘要