GPR-GANs: Generation of Synthetic Ground Penetrating Radargrams Using Generative Adversarial Networks
2021 11th International Workshop on Advanced Ground Penetrating Radar (IWAGPR)(2021)
摘要
In the last decade, autonomous driving has come into the focus of research, and the range of research topics has expanded enormously since then. Autonomous vehicles are usually equipped with different sensors for various purposes, e.g. cameras, radars, lidars and ultrasonic sensors for tasks like environment perception and other drive assistance features. Ground penetrating radars (GPR) have gained recent attention and are being widely used by researchers for the localization of autonomous vehicles. The unique patterns and fingerprints collected by GPR also enable us to perform analysis of sub-surface data and detect underground damages for predictive maintenance of roads. While collecting the data using GPR, it is not feasible to scan each and every track/lane on the road which leads to the problem of limited availability of training data for machine learning algorithms like classification and sub-surface damage detection. Augmenting training images for such tasks has proven to be a reasonable approach to increase training data. In contrast to well-examined methods like linear interpolation and augmentation, generative models expand the output space beyond the given feature space. This work addresses two different problems, the GPR sensor faces while recording the data, i.e. lack of unlimited tracks of the road due to the limited coverage area from GPR sensor and missing data within the recorded GPR tracks due to the high speed of the car.
更多查看译文
关键词
Generative Adversarial Networks (GANs),Ground Penetraing Radar (GPR),Radar-grams,Image Extrapolation,Road Damages
AI 理解论文
溯源树
样例
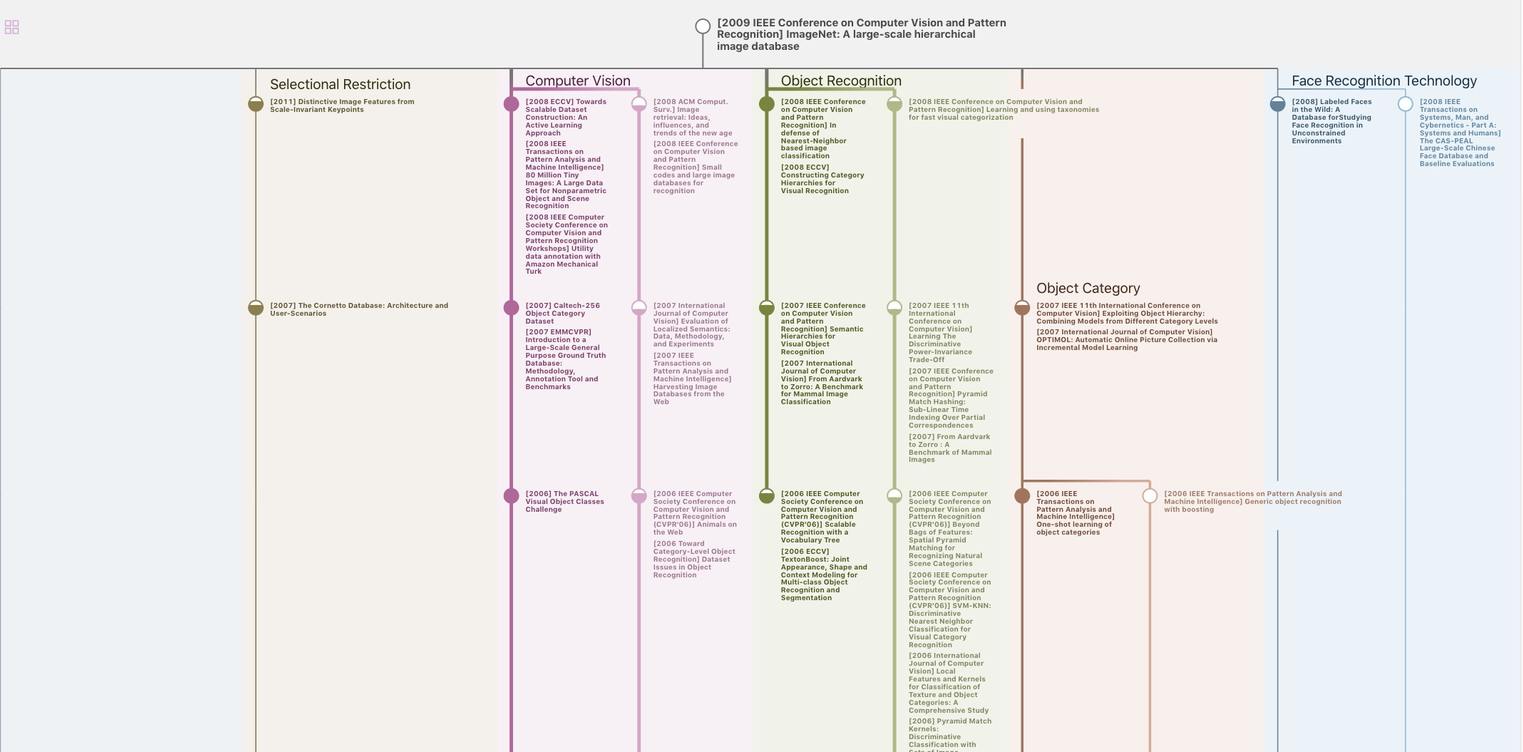
生成溯源树,研究论文发展脉络
Chat Paper
正在生成论文摘要