Natural Language Processing of Radiology Reports to Detect Complications of Ischemic Stroke
Neurocritical Care(2022)
摘要
Background Abstraction of critical data from unstructured radiologic reports using natural language processing (NLP) is a powerful tool to automate the detection of important clinical features and enhance research efforts. We present a set of NLP approaches to identify critical findings in patients with acute ischemic stroke from radiology reports of computed tomography (CT) and magnetic resonance imaging (MRI). Methods We trained machine learning classifiers to identify categorical outcomes of edema, midline shift (MLS), hemorrhagic transformation, and parenchymal hematoma, as well as rule-based systems (RBS) to identify intraventricular hemorrhage (IVH) and continuous MLS measurements within CT/MRI reports. Using a derivation cohort of 2289 reports from 550 individuals with acute middle cerebral artery territory ischemic strokes, we externally validated our models on reports from a separate institution as well as from patients with ischemic strokes in any vascular territory. Results In all data sets, a deep neural network with pretrained biomedical word embeddings (BioClinicalBERT) achieved the highest discrimination performance for binary prediction of edema (area under precision recall curve [AUPRC] > 0.94), MLS (AUPRC > 0.98), hemorrhagic conversion (AUPRC > 0.89), and parenchymal hematoma (AUPRC > 0.76). BioClinicalBERT outperformed lasso regression ( p < 0.001) for all outcomes except parenchymal hematoma ( p = 0.755). Tailored RBS for IVH and continuous MLS outperformed BioClinicalBERT ( p < 0.001) and linear regression, respectively ( p < 0.001). Conclusions Our study demonstrates robust performance and external validity of a core NLP tool kit for identifying both categorical and continuous outcomes of ischemic stroke from unstructured radiographic text data. Medically tailored NLP methods have multiple important big data applications, including scalable electronic phenotyping, augmentation of clinical risk prediction models, and facilitation of automatic alert systems in the hospital setting.
更多查看译文
关键词
Natural language processing, Stroke, Critical care, Diagnostic imaging
AI 理解论文
溯源树
样例
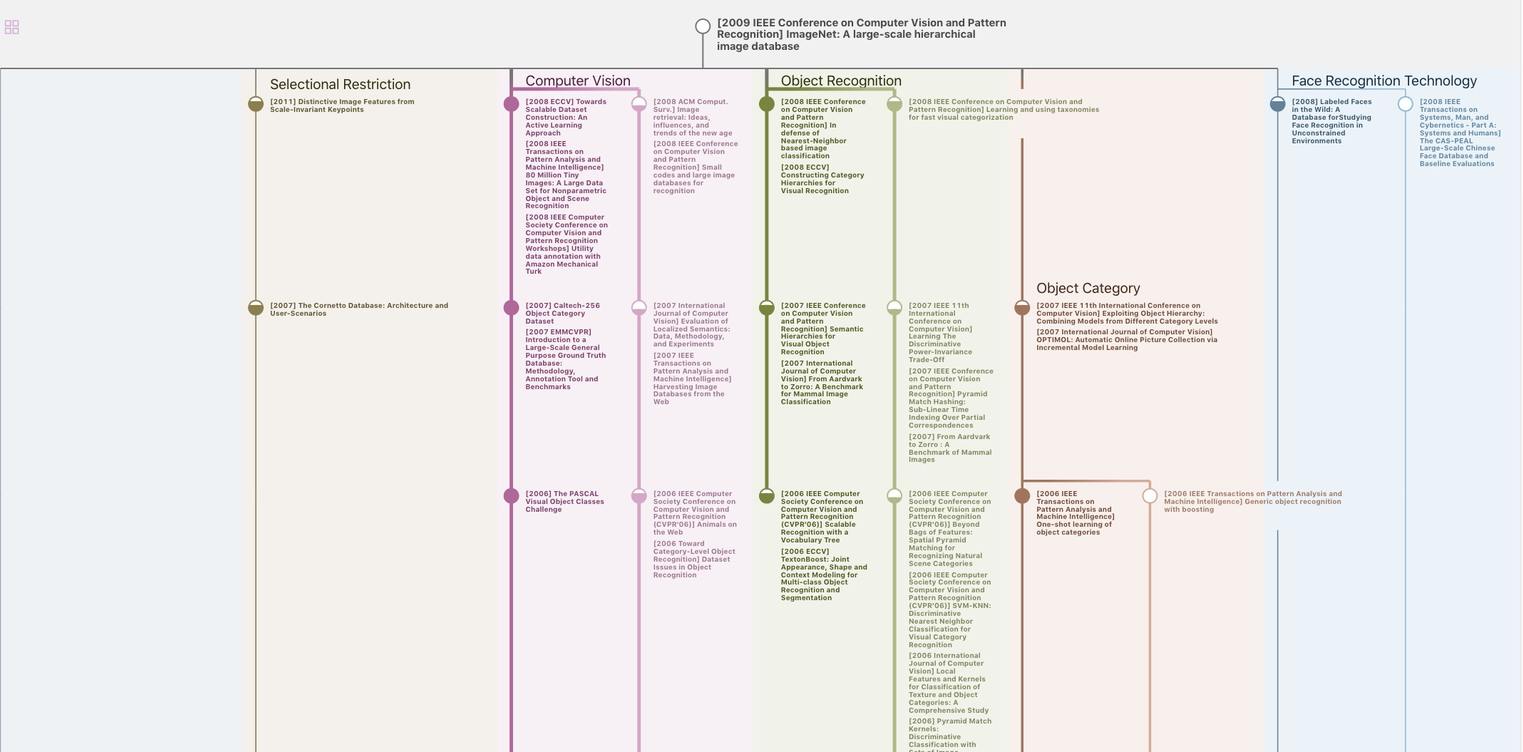
生成溯源树,研究论文发展脉络
Chat Paper
正在生成论文摘要