Constructing Statistical Intervals for Small Area Estimates Based on Generalized Linear Mixed Model in Health Surveys.
Open journal of statistics(2022)
摘要
Generalized Linear Mixed Model (GLMM) has been widely used in small area estimation for health indicators. Bayesian estimation is usually used to construct statistical intervals, however, its computational intensity is a big challenge for large complex surveys. Frequentist approaches, such as bootstrapping, and Monte Carlo (MC) simulation, are also applied but not evaluated in terms of the interval magnitude, width, and the computational time consumed. The 2013 Florida Behavioral Risk Factor Surveillance System data was used as a case study. County-level estimated prevalence of three health-related outcomes was obtained through a GLMM; and their 95% confidence intervals (CIs) were generated from bootstrapping and MC simulation. The intervals were compared to 95% credential intervals through a hierarchial Bayesian model. The results showed that 95% CIs for county-level estimates of each outcome by using MC simulation were similar to the 95% credible intervals generated by Bayesian estimation and were the most computationally efficient. It could be a viable option for constructing statistical intervals for small area estimation in public health practice.
更多查看译文
关键词
Bayesian Estimation,Behavioral Risk Factor Surveillance System,Bootstrapping,Monte Carlo Simulation,Small Area Estimation
AI 理解论文
溯源树
样例
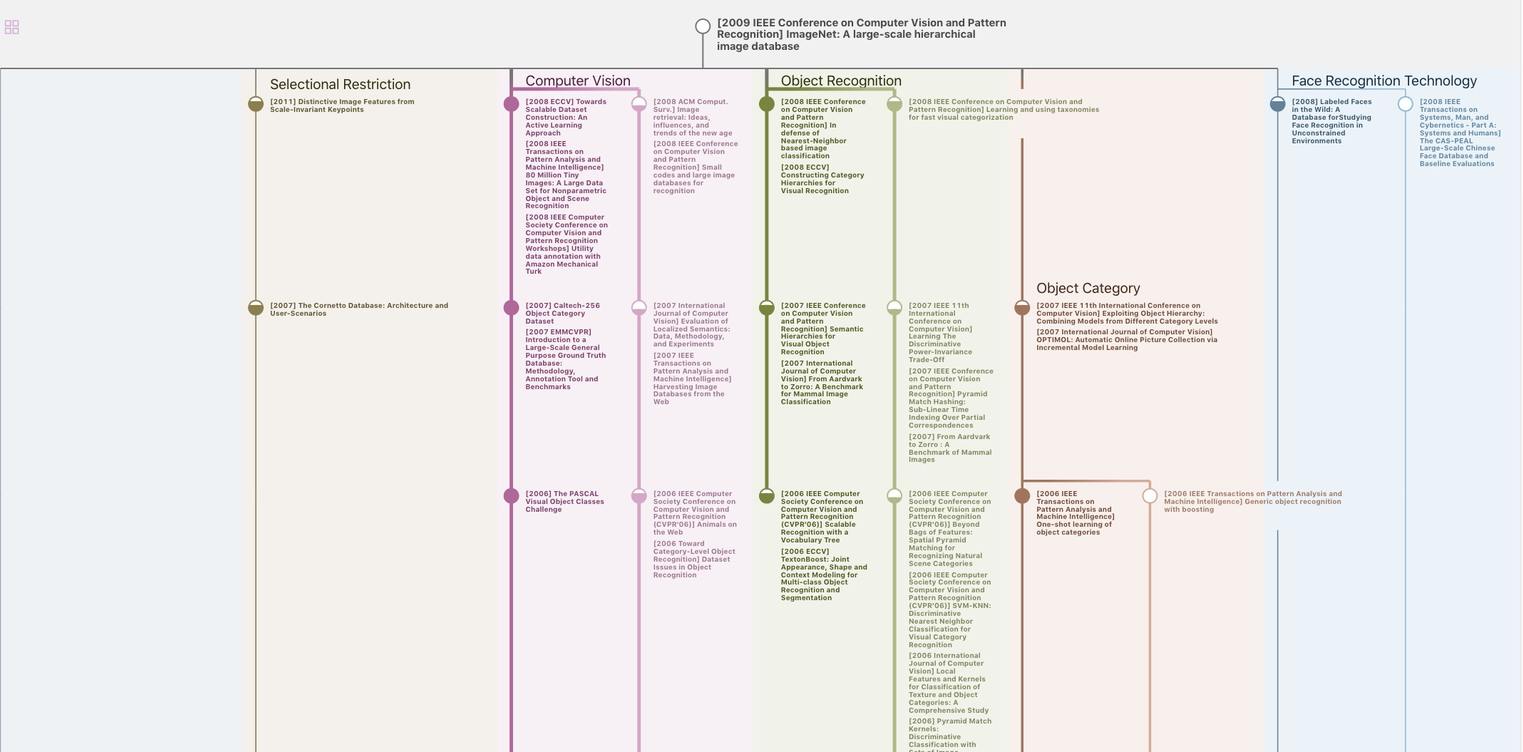
生成溯源树,研究论文发展脉络
Chat Paper
正在生成论文摘要